- Blog Categories
- Software Development
- Data Science
- AI/ML
- Marketing
- General
- MBA
- Management
- Legal
- Software Development Projects and Ideas
- 12 Computer Science Project Ideas
- 28 Beginner Software Projects
- Top 10 Engineering Project Ideas
- Top 10 Easy Final Year Projects
- Top 10 Mini Projects for Engineers
- 25 Best Django Project Ideas
- Top 20 MERN Stack Project Ideas
- Top 12 Real Time Projects
- Top 6 Major CSE Projects
- 12 Robotics Projects for All Levels
- Java Programming Concepts
- Abstract Class in Java and Methods
- Constructor Overloading in Java
- StringBuffer vs StringBuilder
- Java Identifiers: Syntax & Examples
- Types of Variables in Java Explained
- Composition in Java: Examples
- Append in Java: Implementation
- Loose Coupling vs Tight Coupling
- Integrity Constraints in DBMS
- Different Types of Operators Explained
- Career and Interview Preparation in IT
- Top 14 IT Courses for Jobs
- Top 20 Highest Paying Languages
- 23 Top CS Interview Q&A
- Best IT Jobs without Coding
- Software Engineer Salary in India
- 44 Agile Methodology Interview Q&A
- 10 Software Engineering Challenges
- Top 15 Tech's Daily Life Impact
- 10 Best Backends for React
- Cloud Computing Reference Models
- Web Development and Security
- Find Installed NPM Version
- Install Specific NPM Package Version
- Make API Calls in Angular
- Install Bootstrap in Angular
- Use Axios in React: Guide
- StrictMode in React: Usage
- 75 Cyber Security Research Topics
- Top 7 Languages for Ethical Hacking
- Top 20 Docker Commands
- Advantages of OOP
- Data Science Projects and Applications
- 42 Python Project Ideas for Beginners
- 13 Data Science Project Ideas
- 13 Data Structure Project Ideas
- 12 Real-World Python Applications
- Python Banking Project
- Data Science Course Eligibility
- Association Rule Mining Overview
- Cluster Analysis in Data Mining
- Classification in Data Mining
- KDD Process in Data Mining
- Data Structures and Algorithms
- Binary Tree Types Explained
- Binary Search Algorithm
- Sorting in Data Structure
- Binary Tree in Data Structure
- Binary Tree vs Binary Search Tree
- Recursion in Data Structure
- Data Structure Search Methods: Explained
- Binary Tree Interview Q&A
- Linear vs Binary Search
- Priority Queue Overview
- Python Programming and Tools
- Top 30 Python Pattern Programs
- List vs Tuple
- Python Free Online Course
- Method Overriding in Python
- Top 21 Python Developer Skills
- Reverse a Number in Python
- Switch Case Functions in Python
- Info Retrieval System Overview
- Reverse a Number in Python
- Real-World Python Applications
- Data Science Careers and Comparisons
- Data Analyst Salary in India
- Data Scientist Salary in India
- Free Excel Certification Course
- Actuary Salary in India
- Data Analyst Interview Guide
- Pandas Interview Guide
- Tableau Filters Explained
- Data Mining Techniques Overview
- Data Analytics Lifecycle Phases
- Data Science Vs Analytics Comparison
- Artificial Intelligence and Machine Learning Projects
- Exciting IoT Project Ideas
- 16 Exciting AI Project Ideas
- 45+ Interesting ML Project Ideas
- Exciting Deep Learning Projects
- 12 Intriguing Linear Regression Projects
- 13 Neural Network Projects
- 5 Exciting Image Processing Projects
- Top 8 Thrilling AWS Projects
- 12 Engaging AI Projects in Python
- NLP Projects for Beginners
- Concepts and Algorithms in AIML
- Basic CNN Architecture Explained
- 6 Types of Regression Models
- Data Preprocessing Steps
- Bagging vs Boosting in ML
- Multinomial Naive Bayes Overview
- Bayesian Network Example
- Bayes Theorem Guide
- Top 10 Dimensionality Reduction Techniques
- Neural Network Step-by-Step Guide
- Technical Guides and Comparisons
- Make a Chatbot in Python
- Compute Square Roots in Python
- Permutation vs Combination
- Image Segmentation Techniques
- Generative AI vs Traditional AI
- AI vs Human Intelligence
- Random Forest vs Decision Tree
- Neural Network Overview
- Perceptron Learning Algorithm
- Selection Sort Algorithm
- Career and Practical Applications in AIML
- AI Salary in India Overview
- Biological Neural Network Basics
- Top 10 AI Challenges
- Production System in AI
- Top 8 Raspberry Pi Alternatives
- Top 8 Open Source Projects
- 14 Raspberry Pi Project Ideas
- 15 MATLAB Project Ideas
- Top 10 Python NLP Libraries
- Naive Bayes Explained
- Digital Marketing Projects and Strategies
- 10 Best Digital Marketing Projects
- 17 Fun Social Media Projects
- Top 6 SEO Project Ideas
- Digital Marketing Case Studies
- Coca-Cola Marketing Strategy
- Nestle Marketing Strategy Analysis
- Zomato Marketing Strategy
- Monetize Instagram Guide
- Become a Successful Instagram Influencer
- 8 Best Lead Generation Techniques
- Digital Marketing Careers and Salaries
- Digital Marketing Salary in India
- Top 10 Highest Paying Marketing Jobs
- Highest Paying Digital Marketing Jobs
- SEO Salary in India
- Content Writer Salary Guide
- Digital Marketing Executive Roles
- Career in Digital Marketing Guide
- Future of Digital Marketing
- MBA in Digital Marketing Overview
- Digital Marketing Techniques and Channels
- 9 Types of Digital Marketing Channels
- Top 10 Benefits of Marketing Branding
- 100 Best YouTube Channel Ideas
- YouTube Earnings in India
- 7 Reasons to Study Digital Marketing
- Top 10 Digital Marketing Objectives
- 10 Best Digital Marketing Blogs
- Top 5 Industries Using Digital Marketing
- Growth of Digital Marketing in India
- Top Career Options in Marketing
- Interview Preparation and Skills
- 73 Google Analytics Interview Q&A
- 56 Social Media Marketing Q&A
- 78 Google AdWords Interview Q&A
- Top 133 SEO Interview Q&A
- 27+ Digital Marketing Q&A
- Digital Marketing Free Course
- Top 9 Skills for PPC Analysts
- Movies with Successful Social Media Campaigns
- Marketing Communication Steps
- Top 10 Reasons to Be an Affiliate Marketer
- Career Options and Paths
- Top 25 Highest Paying Jobs India
- Top 25 Highest Paying Jobs World
- Top 10 Highest Paid Commerce Job
- Career Options After 12th Arts
- Top 7 Commerce Courses Without Maths
- Top 7 Career Options After PCB
- Best Career Options for Commerce
- Career Options After 12th CS
- Top 10 Career Options After 10th
- 8 Best Career Options After BA
- Projects and Academic Pursuits
- 17 Exciting Final Year Projects
- Top 12 Commerce Project Topics
- Top 13 BCA Project Ideas
- Career Options After 12th Science
- Top 15 CS Jobs in India
- 12 Best Career Options After M.Com
- 9 Best Career Options After B.Sc
- 7 Best Career Options After BCA
- 22 Best Career Options After MCA
- 16 Top Career Options After CE
- Courses and Certifications
- 10 Best Job-Oriented Courses
- Best Online Computer Courses
- Top 15 Trending Online Courses
- Top 19 High Salary Certificate Courses
- 21 Best Programming Courses for Jobs
- What is SGPA? Convert to CGPA
- GPA to Percentage Calculator
- Highest Salary Engineering Stream
- 15 Top Career Options After Engineering
- 6 Top Career Options After BBA
- Job Market and Interview Preparation
- Why Should You Be Hired: 5 Answers
- Top 10 Future Career Options
- Top 15 Highest Paid IT Jobs India
- 5 Common Guesstimate Interview Q&A
- Average CEO Salary: Top Paid CEOs
- Career Options in Political Science
- Top 15 Highest Paying Non-IT Jobs
- Cover Letter Examples for Jobs
- Top 5 Highest Paying Freelance Jobs
- Top 10 Highest Paying Companies India
- Career Options and Paths After MBA
- 20 Best Careers After B.Com
- Career Options After MBA Marketing
- Top 14 Careers After MBA In HR
- Top 10 Highest Paying HR Jobs India
- How to Become an Investment Banker
- Career Options After MBA - High Paying
- Scope of MBA in Operations Management
- Best MBA for Working Professionals India
- MBA After BA - Is It Right For You?
- Best Online MBA Courses India
- MBA Project Ideas and Topics
- 11 Exciting MBA HR Project Ideas
- Top 15 MBA Project Ideas
- 18 Exciting MBA Marketing Projects
- MBA Project Ideas: Consumer Behavior
- What is Brand Management?
- What is Holistic Marketing?
- What is Green Marketing?
- Intro to Organizational Behavior Model
- Tech Skills Every MBA Should Learn
- Most Demanding Short Term Courses MBA
- MBA Salary, Resume, and Skills
- MBA Salary in India
- HR Salary in India
- Investment Banker Salary India
- MBA Resume Samples
- Sample SOP for MBA
- Sample SOP for Internship
- 7 Ways MBA Helps Your Career
- Must-have Skills in Sales Career
- 8 Skills MBA Helps You Improve
- Top 20+ SAP FICO Interview Q&A
- MBA Specializations and Comparative Guides
- Why MBA After B.Tech? 5 Reasons
- How to Answer 'Why MBA After Engineering?'
- Why MBA in Finance
- MBA After BSc: 10 Reasons
- Which MBA Specialization to choose?
- Top 10 MBA Specializations
- MBA vs Masters: Which to Choose?
- Benefits of MBA After CA
- 5 Steps to Management Consultant
- 37 Must-Read HR Interview Q&A
- Fundamentals and Theories of Management
- What is Management? Objectives & Functions
- Nature and Scope of Management
- Decision Making in Management
- Management Process: Definition & Functions
- Importance of Management
- What are Motivation Theories?
- Tools of Financial Statement Analysis
- Negotiation Skills: Definition & Benefits
- Career Development in HRM
- Top 20 Must-Have HRM Policies
- Project and Supply Chain Management
- Top 20 Project Management Case Studies
- 10 Innovative Supply Chain Projects
- Latest Management Project Topics
- 10 Project Management Project Ideas
- 6 Types of Supply Chain Models
- Top 10 Advantages of SCM
- Top 10 Supply Chain Books
- What is Project Description?
- Top 10 Project Management Companies
- Best Project Management Courses Online
- Salaries and Career Paths in Management
- Project Manager Salary in India
- Average Product Manager Salary India
- Supply Chain Management Salary India
- Salary After BBA in India
- PGDM Salary in India
- Top 7 Career Options in Management
- CSPO Certification Cost
- Why Choose Product Management?
- Product Management in Pharma
- Product Design in Operations Management
- Industry-Specific Management and Case Studies
- Amazon Business Case Study
- Service Delivery Manager Job
- Product Management Examples
- Product Management in Automobiles
- Product Management in Banking
- Sample SOP for Business Management
- Video Game Design Components
- Top 5 Business Courses India
- Free Management Online Course
- SCM Interview Q&A
- Fundamentals and Types of Law
- Acceptance in Contract Law
- Offer in Contract Law
- 9 Types of Evidence
- Types of Law in India
- Introduction to Contract Law
- Negotiable Instrument Act
- Corporate Tax Basics
- Intellectual Property Law
- Workmen Compensation Explained
- Lawyer vs Advocate Difference
- Law Education and Courses
- LLM Subjects & Syllabus
- Corporate Law Subjects
- LLM Course Duration
- Top 10 Online LLM Courses
- Online LLM Degree
- Step-by-Step Guide to Studying Law
- Top 5 Law Books to Read
- Why Legal Studies?
- Pursuing a Career in Law
- How to Become Lawyer in India
- Career Options and Salaries in Law
- Career Options in Law India
- Corporate Lawyer Salary India
- How To Become a Corporate Lawyer
- Career in Law: Starting, Salary
- Career Opportunities: Corporate Law
- Business Lawyer: Role & Salary Info
- Average Lawyer Salary India
- Top Career Options for Lawyers
- Types of Lawyers in India
- Steps to Become SC Lawyer in India
- Tutorials
- Software Tutorials
- C Tutorials
- Recursion in C: Fibonacci Series
- Checking String Palindromes in C
- Prime Number Program in C
- Implementing Square Root in C
- Matrix Multiplication in C
- Understanding Double Data Type
- Factorial of a Number in C
- Structure of a C Program
- Building a Calculator Program in C
- Compiling C Programs on Linux
- Java Tutorials
- Handling String Input in Java
- Determining Even and Odd Numbers
- Prime Number Checker
- Sorting a String
- User-Defined Exceptions
- Understanding the Thread Life Cycle
- Swapping Two Numbers
- Using Final Classes
- Area of a Triangle
- Skills
- Explore Skills
- Management Skills
- Software Engineering
- JavaScript
- Data Structure
- React.js
- Core Java
- Node.js
- Blockchain
- SQL
- Full stack development
- Devops
- NFT
- BigData
- Cyber Security
- Cloud Computing
- Database Design with MySQL
- Cryptocurrency
- Python
- Digital Marketings
- Advertising
- Influencer Marketing
- Performance Marketing
- Search Engine Marketing
- Email Marketing
- Content Marketing
- Social Media Marketing
- Display Advertising
- Marketing Analytics
- Web Analytics
- Affiliate Marketing
- MBA
- MBA in Finance
- MBA in HR
- MBA in Marketing
- MBA in Business Analytics
- MBA in Operations Management
- MBA in International Business
- MBA in Information Technology
- MBA in Healthcare Management
- MBA In General Management
- MBA in Agriculture
- MBA in Supply Chain Management
- MBA in Entrepreneurship
- MBA in Project Management
- Management Program
- Consumer Behaviour
- Supply Chain Management
- Financial Analytics
- Introduction to Fintech
- Introduction to HR Analytics
- Fundamentals of Communication
- Art of Effective Communication
- Introduction to Research Methodology
- Mastering Sales Technique
- Business Communication
- Fundamentals of Journalism
- Economics Masterclass
- Free Courses
- Home
- Blog
- Data Science
- Banking Data Analysis Under the Scanner
Banking Data Analysis Under the Scanner
Updated on Jun 30, 2023 | 13 min read
Share:
Table of Contents
Industries of the 21st century are highly dependent on data to develop insights and make data-driven decisions. Data science has thus no longer remained a tangential need, but is imperative to businesses. In this article, we will examine the use of data science and analytics as a key disruptor in the financial services sector by diving into data collection, analysis and integration through diverse banking touchpoints such as branches, mobile apps, online platforms, call centres and ATMs, and correlating it to a bank’s strategy.
It is not surprising that data is deeply correlated with the business efficiency of financial institutions, especially banks. Banking is one of the key business domains, which makes the highest investment in big data and BA (business analytics) technologies. The application of data science in banking has got a lot of acceptance from half the planet. The banking sector has been facing intense competition from non-traditional players like mobile-only banks and UPI (Unified Payments Interface) applications, and needs to look for effective instruments to expand revenues and contain costs.
Internationally, market research companies have predicted that financial services companies will witness data growth at a CAGR of over 25% in 2018-2025. This means that data collection and analysis are not just imperative but also integral to the success of banking strategies worldwide. We dive into the workflow of a data scientist and the impact of data analysis on banking strategies in the future.
Learn Data Science Courses online at upGrad
Data Collection and Management
Over the last decade, data collection in the banking sector has become extremely sophisticated and has transitioned from bank ledgers to online transaction history, including collection from other touchpoints such as apps, call centres and ATMs. In fact, it is believed that half the world’s adult population now uses digital banking, and this statistic is bound to grow rapidly.
This means that every credit card transaction, message and web page opened on a banking website adds to the billions of bytes of data being collected by the global population. Along with this, the intense economic digitisation has provided banks with a fresh pool to tap into in the form of external sources such as mobile operators, geospatial data, credit bureaus and social networks. For a data analyst, the first step is to define and procure information from this pool, to structure and use it effectively as intelligence for making smart business decisions and staying competitive.
Data management is the most important step in effectively harnessing data that is collected painstakingly from various channels. This includes keeping an eye on data ownership, governance and storage, and structuring, data review and cleaning.
All these processes can be accomplished with the help of cloud-based storage, on-site servers, data warehouses and data lakes; by integrating CBS, CRM, LOS and other systems; with analytical and data visualisation tools; and through the creation of an organisational structure to optimise data usage. Additionally, centralised ownership of data ensures there is complete accountability within an organisation.
Integrating Analytics
Given that data is the greatest asset for banks, they can only reap benefits by integrating data with analytics. Raw data alone cannot be of any use unless it is supported by robust analysis. The job of a data analyst includes creating models to predict customer behaviour, understand their preferences and proactively manage expectations.
Forward-looking analytics provide proactive triggers, such as default, attrition, propensity to buy a specific product, to predict future events in the journey of a consumer and, in turn, help the bank take effective actions. Over the years, rapid processing needs, innovative mobile technology, data availability and proliferation of open-source software have produced several use cases of data science in banking.
Some of these include customer targeting and segmentation, customer activation and drop-out prevention, cross-selling models, and credit and risk management. Integrating analytics with data is critical for the following reasons.
1. Risk Modelling
Risk modelling refers to the use of formal econometric techniques to determine the aggregate risk in a financial portfolio. Financial risk modelling is the most important blue-print for a bank when analysing businesses as well as individuals. The techniques used for risk modelling involve evaluating market risk, value at risk (VaR), historical simulation and using the extreme value theory to forecast likely losses for a variety of risks.
The different risks are also clubbed under the credit risk, market risk, model risk, liquidity risk and operational risk categories for businesses. Risk modelling should be done keeping in mind regional and international banking standards. With large amounts of data collected, powerful computing software can be used to perform quantitative risk analysis.
2. Fraud Detection
Data analysis enables auditors and fraud examiners to analyse an organisation’s business data to determine how well it has implemented internal controls to identify fraudulent transactions or activity. Some of the techniques employed for this purpose include calculation of statistical parameters (averages, standard deviations, unnatural high or low values), classification of data, arranging data to point to anomalies, digital analysis for unexpected occurrences, matching values, duplicate testing, gap testing, summing and validating entries.
Our learners also read: Learn Python Online Course Free
Explore our Popular Data Science Certifications
3. Real-Time Predictive Analysis
As the banking industry becomes more dynamic, banks are under constant pressure to stay profitable and, at the same time, understand the needs, wants and preferences of their customers. There is a rapid time crunch between understanding the data and evolving a strategy. To address this time crunch, there is a need for banks to adopt real-time predictive analysis in order to respond in the fastest possible manner.
The benefit of predictive analysis is that it supports ongoing learning from current data rather than relying on historical data. It can detect unfamiliar trends, rather than operating in a defined structure, and requires building models rather than using traditional ones.
It adapts visual discovery tools that are easier to use and considers external data as well. In effect, predictive analysis is more dynamic and bespoke. A few areas where predictive analysis comes in handy include customer relationship enhancement, collateral and liquidity management, cash flow management, helping trade and supply chain financing, risk management and support operations.
4. Understanding Consumer Sentiment
In a fiercely competitive banking sector, understanding and managing consumer sentiment & analysis are integral to the operation of any bank. Banks are rapidly seeking AI (Artificial Intelligence) solutions in the form of sentiment analysis. This is needed to capture a customer’s reaction to a product, situation or event, and respond to it through texts, posts, reviews and other digital content.
Natural Language Processing (NLP) techniques are implemented for nuanced analysis of data. The data is usually collected from chatbots, virtual assistants, online translation services and more such channels. Banks can then use this as subjective information to personalise communication, prioritise customer issues, improve products and services, and enhance overall customer satisfaction.
Top Data Science Skills to Learn to upskill
SL. No | Top Data Science Skills to Learn | |
1 |
Data Analysis Online Courses | Inferential Statistics Online Courses |
2 |
Hypothesis Testing Online Courses | Logistic Regression Online Courses |
3 |
Linear Regression Courses | Linear Algebra for Analysis Online Courses |
5. Customer Segmentation, Profiling and Marketing Segmentation
As we accelerate into the digital world, there is a need for banks to adopt a data-based approach to consumer segmentation, profiling and market segmentation in order to customise their strategies for different audiences.
Banks need to upgrade their customer strategies frequently to fulfil the demands of the new and existing bases, and segmentation is critical to achieving this. Banks need to look at the entire cycle of creating customer value segments and understanding the customer relationship life cycle to create or upgrade their products accordingly.
6. Customer Lifetime Value
Customer Lifetime Value, or CLTV, refers to the complete financial value that a customer brings to the bank over his or her entire relationship with the bank. CLTV is calculated considering loyalty to the bank, the profitability of the customer, the average balances of loans and savings on a per customer basis, the average interest rate margin, average income or revenue per customer generated from non-interest income sources, and the cost of providing customer services and access. Automation in the calculation of the CLTV based on these factors produces faster results, and enables banks to change or upgrade their strategies faster.
upGrad’s Exclusive Data Science Webinar for you –
Transformation & Opportunities in Analytics & Insights
7. Digital Assistants and Chatbots
Digital assistants and chatbots have revolutionised service and business communication for banks. Chatbots are creating a stir in social media. From assisting people with performing simple tasks to giving them a personalised experience, virtual assistants and chatbots have made banking easier.
8. Voice Recognition and Predictive Analysis
Banks have been able to test security features using AI-based voice recognition, which can automatically confirm the identity of a customer when they call into customer service. Given the success of this technology, customers may be able to speak and perform simple tasks such as transferring money, reporting stolen or lost ATM and credit cards, and receiving an accurate response with a virtual assistant.
Most of the urban bank users rely heavily on mobile banking, which has given way to AI-powered banking mobile apps. Most of these apps offer personal, contextual and predictive services. These are intelligent apps that can track the user’s behaviour, and provide them with personalised tips and insights on savings and expenses.
Read our popular Data Science Articles
Aligning with Banking Strategies
The third step after data collection and modelling is to use such intelligence to optimise marketing campaigns, create relevant ads, automate outreach and optimise customer onboarding. Analytics is a key strategic pillar, apart from operations, that can greatly improve functional areas such as risk, compliance, fraud and non-performing asset (NPA) monitoring, and calculation of VaR. Banks can reduce excessive servicing costs and increase profitability if these actions are performed with the least amount of roadblocks.
Future of Data Science in Banking
To push the boundaries of data utilisation, the future of banking is highly dependent on data encryption, AI and cloud computing. Since sensitive data is key to business, fully encrypted data can ensure the security of ledgers that are impossible to tamper with and also guarantee financial security. It can also help in eliminating third-party verifications, thereby speeding up processes and saving on transaction fees.
Other revolutionary fits to the future of data science in banking are seen in AI functions such as automated customer service response, real-time monitoring of regulatory requirements and algorithmic or micro-trading. The future of banking also includes cloud computing, which offers banks unlimited hardware and software resources that can help them scale up or down as per their requirements.
Cloud technology can also help banks lower their infrastructure costs, improve flexibility, increase efficiency and serve clients faster; all of this will contribute to a more efficient infrastructure and ensure a better relationship with the consumers. However, cloud computing means significant regulatory implications for banks. Some of the key issues that banks are trying to address currently include ensuring service continuity for customers in the face of any potential mishap on the cloud and the need to transition back to own databases in such scenarios.
Other important issues being evaluated include the way personal information is stored and used, customer data protection, dependence on third-party providers and security of cloud infrastructure, and potential mixing of financial data with other data on shared servers.
Tips for Successful Data Analytics in the Banking Sector
A few valuable tips that can help with the creation of an effective data analytics strategy in the banking sector are as follows:
Start Slow and Proceed Gradually
You don’t have to hurry and get everything done at once. Doing that will be expensive, irrational, and will fail to deliver the outcomes you need. You should start small and try to figure out what works and what does not. After that, you can gradually proceed and use your small wins for future projects and enjoy greater ROI.
Invest in OCM
If you want better results from data analysis in the banking sector, you will have to focus on developing an effective OCM strategy. Adopting organization-wide digital transformation won’t happen by chance. Instead, you will have to focus on hard work and determination. If you want to efficiently execute and manage banking analytics, you should follow this structured process:
- Begin by analyzing the company landscape to set up your long-term visions as well as short-term goals and metrics.
- Evaluate and calculate the necessary technology and training investments.
- Convey the changes to your employees, including how they will be impacted and how the institution will be benefitted.
- Measure results to determine that all KPIs and ROI goals are on track.
- Establish a feedback loop so that employees can convey what’s working for them and where they are struggling.
- Create a support structure to ensure that employees have someone to turn to when they encounter different issues.
- Make training investments to help employees adjust to the latest systems, protocols, and processes.
- Review your OCM strategy later to determine how far it has been helping with data analytics in banking.
Learn By Trial and Error
While implementing a banking analytics strategy, you should treat it as an iterative process. All projects should be considered meaningful learning opportunities regarding different lines of business and the institution as a whole.
Once you fully understand what works through the trial and error method, you are required to eliminate tools, processes, and policies that no longer serve you. No matter how accustomed your employees are to them, you won’t be able to drive results from outdated or inefficient tools and policies.
Ask the Right Questions
If you want to extract the maximum benefits of data analysis in the banking sector, you will have to ask the right questions. Knowing what you need and what you intend to achieve will help you frame valuable questions. Figuring out what questions will help you acquire maximum information will also prevent the wastage of your valuable resources.
At times, you will be able to extract valuable information from unexpected places. Therefore, you should look for answers from all data sets.
If you want to form a comprehensive view of your customers, you will have to invest in a customer data platform. It will ensure that you can collect data from every contact point and enable that knowledge to be shared all over the institution.
Final Thoughts
The future of data in banking also holds the promise of teams getting rid of a ‘siloed’ approach and using insights for the entire organisation. With data science, enterprises are likely to be more connected, instead of using separate analytics practices.
For example, marketing and digital (web, social and mobile) analytics, credit risk analytics, operations analytics, fraud analytics and compliance analytics will be able to leverage the same data structures. For instance, a team looking at increasing activation and product penetration by cross-selling across channels could potentially uncover fraudulent behaviour and anomalies.
The future of banking will involve leveraging data collected from various sources to get accurate customer insights and use advanced analytics with the help of digital technologies for increased profits and efficiency.
If you are curious to learn about data science applications in banking & various sectors, check out IIIT-B & upGrad’s PG Diploma in Data Science which is created for working professionals and offers 10+ case studies & projects, practical hands-on workshops, mentorship with industry experts, 1-on-1 with industry mentors, 400+ hours of learning and job assistance with top firms.
Frequently Asked Questions (FAQs)
1. What is the hiring process of data scientists at Citibank?
2. Is Python used in investment banking?
3. Should I learn Python or R for finance?
Get Free Consultation
By submitting, I accept the T&C and
Privacy Policy
Start Your Career in Data Science Today
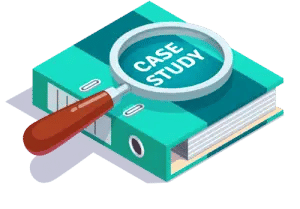