- Blog Categories
- Software Development
- Data Science
- AI/ML
- Marketing
- General
- MBA
- Management
- Legal
- Software Development Projects and Ideas
- 12 Computer Science Project Ideas
- 28 Beginner Software Projects
- Top 10 Engineering Project Ideas
- Top 10 Easy Final Year Projects
- Top 10 Mini Projects for Engineers
- 25 Best Django Project Ideas
- Top 20 MERN Stack Project Ideas
- Top 12 Real Time Projects
- Top 6 Major CSE Projects
- 12 Robotics Projects for All Levels
- Java Programming Concepts
- Abstract Class in Java and Methods
- Constructor Overloading in Java
- StringBuffer vs StringBuilder
- Java Identifiers: Syntax & Examples
- Types of Variables in Java Explained
- Composition in Java: Examples
- Append in Java: Implementation
- Loose Coupling vs Tight Coupling
- Integrity Constraints in DBMS
- Different Types of Operators Explained
- Career and Interview Preparation in IT
- Top 14 IT Courses for Jobs
- Top 20 Highest Paying Languages
- 23 Top CS Interview Q&A
- Best IT Jobs without Coding
- Software Engineer Salary in India
- 44 Agile Methodology Interview Q&A
- 10 Software Engineering Challenges
- Top 15 Tech's Daily Life Impact
- 10 Best Backends for React
- Cloud Computing Reference Models
- Web Development and Security
- Find Installed NPM Version
- Install Specific NPM Package Version
- Make API Calls in Angular
- Install Bootstrap in Angular
- Use Axios in React: Guide
- StrictMode in React: Usage
- 75 Cyber Security Research Topics
- Top 7 Languages for Ethical Hacking
- Top 20 Docker Commands
- Advantages of OOP
- Data Science Projects and Applications
- 42 Python Project Ideas for Beginners
- 13 Data Science Project Ideas
- 13 Data Structure Project Ideas
- 12 Real-World Python Applications
- Python Banking Project
- Data Science Course Eligibility
- Association Rule Mining Overview
- Cluster Analysis in Data Mining
- Classification in Data Mining
- KDD Process in Data Mining
- Data Structures and Algorithms
- Binary Tree Types Explained
- Binary Search Algorithm
- Sorting in Data Structure
- Binary Tree in Data Structure
- Binary Tree vs Binary Search Tree
- Recursion in Data Structure
- Data Structure Search Methods: Explained
- Binary Tree Interview Q&A
- Linear vs Binary Search
- Priority Queue Overview
- Python Programming and Tools
- Top 30 Python Pattern Programs
- List vs Tuple
- Python Free Online Course
- Method Overriding in Python
- Top 21 Python Developer Skills
- Reverse a Number in Python
- Switch Case Functions in Python
- Info Retrieval System Overview
- Reverse a Number in Python
- Real-World Python Applications
- Data Science Careers and Comparisons
- Data Analyst Salary in India
- Data Scientist Salary in India
- Free Excel Certification Course
- Actuary Salary in India
- Data Analyst Interview Guide
- Pandas Interview Guide
- Tableau Filters Explained
- Data Mining Techniques Overview
- Data Analytics Lifecycle Phases
- Data Science Vs Analytics Comparison
- Artificial Intelligence and Machine Learning Projects
- Exciting IoT Project Ideas
- 16 Exciting AI Project Ideas
- 45+ Interesting ML Project Ideas
- Exciting Deep Learning Projects
- 12 Intriguing Linear Regression Projects
- 13 Neural Network Projects
- 5 Exciting Image Processing Projects
- Top 8 Thrilling AWS Projects
- 12 Engaging AI Projects in Python
- NLP Projects for Beginners
- Concepts and Algorithms in AIML
- Basic CNN Architecture Explained
- 6 Types of Regression Models
- Data Preprocessing Steps
- Bagging vs Boosting in ML
- Multinomial Naive Bayes Overview
- Bayesian Network Example
- Bayes Theorem Guide
- Top 10 Dimensionality Reduction Techniques
- Neural Network Step-by-Step Guide
- Technical Guides and Comparisons
- Make a Chatbot in Python
- Compute Square Roots in Python
- Permutation vs Combination
- Image Segmentation Techniques
- Generative AI vs Traditional AI
- AI vs Human Intelligence
- Random Forest vs Decision Tree
- Neural Network Overview
- Perceptron Learning Algorithm
- Selection Sort Algorithm
- Career and Practical Applications in AIML
- AI Salary in India Overview
- Biological Neural Network Basics
- Top 10 AI Challenges
- Production System in AI
- Top 8 Raspberry Pi Alternatives
- Top 8 Open Source Projects
- 14 Raspberry Pi Project Ideas
- 15 MATLAB Project Ideas
- Top 10 Python NLP Libraries
- Naive Bayes Explained
- Digital Marketing Projects and Strategies
- 10 Best Digital Marketing Projects
- 17 Fun Social Media Projects
- Top 6 SEO Project Ideas
- Digital Marketing Case Studies
- Coca-Cola Marketing Strategy
- Nestle Marketing Strategy Analysis
- Zomato Marketing Strategy
- Monetize Instagram Guide
- Become a Successful Instagram Influencer
- 8 Best Lead Generation Techniques
- Digital Marketing Careers and Salaries
- Digital Marketing Salary in India
- Top 10 Highest Paying Marketing Jobs
- Highest Paying Digital Marketing Jobs
- SEO Salary in India
- Content Writer Salary Guide
- Digital Marketing Executive Roles
- Career in Digital Marketing Guide
- Future of Digital Marketing
- MBA in Digital Marketing Overview
- Digital Marketing Techniques and Channels
- 9 Types of Digital Marketing Channels
- Top 10 Benefits of Marketing Branding
- 100 Best YouTube Channel Ideas
- YouTube Earnings in India
- 7 Reasons to Study Digital Marketing
- Top 10 Digital Marketing Objectives
- 10 Best Digital Marketing Blogs
- Top 5 Industries Using Digital Marketing
- Growth of Digital Marketing in India
- Top Career Options in Marketing
- Interview Preparation and Skills
- 73 Google Analytics Interview Q&A
- 56 Social Media Marketing Q&A
- 78 Google AdWords Interview Q&A
- Top 133 SEO Interview Q&A
- 27+ Digital Marketing Q&A
- Digital Marketing Free Course
- Top 9 Skills for PPC Analysts
- Movies with Successful Social Media Campaigns
- Marketing Communication Steps
- Top 10 Reasons to Be an Affiliate Marketer
- Career Options and Paths
- Top 25 Highest Paying Jobs India
- Top 25 Highest Paying Jobs World
- Top 10 Highest Paid Commerce Job
- Career Options After 12th Arts
- Top 7 Commerce Courses Without Maths
- Top 7 Career Options After PCB
- Best Career Options for Commerce
- Career Options After 12th CS
- Top 10 Career Options After 10th
- 8 Best Career Options After BA
- Projects and Academic Pursuits
- 17 Exciting Final Year Projects
- Top 12 Commerce Project Topics
- Top 13 BCA Project Ideas
- Career Options After 12th Science
- Top 15 CS Jobs in India
- 12 Best Career Options After M.Com
- 9 Best Career Options After B.Sc
- 7 Best Career Options After BCA
- 22 Best Career Options After MCA
- 16 Top Career Options After CE
- Courses and Certifications
- 10 Best Job-Oriented Courses
- Best Online Computer Courses
- Top 15 Trending Online Courses
- Top 19 High Salary Certificate Courses
- 21 Best Programming Courses for Jobs
- What is SGPA? Convert to CGPA
- GPA to Percentage Calculator
- Highest Salary Engineering Stream
- 15 Top Career Options After Engineering
- 6 Top Career Options After BBA
- Job Market and Interview Preparation
- Why Should You Be Hired: 5 Answers
- Top 10 Future Career Options
- Top 15 Highest Paid IT Jobs India
- 5 Common Guesstimate Interview Q&A
- Average CEO Salary: Top Paid CEOs
- Career Options in Political Science
- Top 15 Highest Paying Non-IT Jobs
- Cover Letter Examples for Jobs
- Top 5 Highest Paying Freelance Jobs
- Top 10 Highest Paying Companies India
- Career Options and Paths After MBA
- 20 Best Careers After B.Com
- Career Options After MBA Marketing
- Top 14 Careers After MBA In HR
- Top 10 Highest Paying HR Jobs India
- How to Become an Investment Banker
- Career Options After MBA - High Paying
- Scope of MBA in Operations Management
- Best MBA for Working Professionals India
- MBA After BA - Is It Right For You?
- Best Online MBA Courses India
- MBA Project Ideas and Topics
- 11 Exciting MBA HR Project Ideas
- Top 15 MBA Project Ideas
- 18 Exciting MBA Marketing Projects
- MBA Project Ideas: Consumer Behavior
- What is Brand Management?
- What is Holistic Marketing?
- What is Green Marketing?
- Intro to Organizational Behavior Model
- Tech Skills Every MBA Should Learn
- Most Demanding Short Term Courses MBA
- MBA Salary, Resume, and Skills
- MBA Salary in India
- HR Salary in India
- Investment Banker Salary India
- MBA Resume Samples
- Sample SOP for MBA
- Sample SOP for Internship
- 7 Ways MBA Helps Your Career
- Must-have Skills in Sales Career
- 8 Skills MBA Helps You Improve
- Top 20+ SAP FICO Interview Q&A
- MBA Specializations and Comparative Guides
- Why MBA After B.Tech? 5 Reasons
- How to Answer 'Why MBA After Engineering?'
- Why MBA in Finance
- MBA After BSc: 10 Reasons
- Which MBA Specialization to choose?
- Top 10 MBA Specializations
- MBA vs Masters: Which to Choose?
- Benefits of MBA After CA
- 5 Steps to Management Consultant
- 37 Must-Read HR Interview Q&A
- Fundamentals and Theories of Management
- What is Management? Objectives & Functions
- Nature and Scope of Management
- Decision Making in Management
- Management Process: Definition & Functions
- Importance of Management
- What are Motivation Theories?
- Tools of Financial Statement Analysis
- Negotiation Skills: Definition & Benefits
- Career Development in HRM
- Top 20 Must-Have HRM Policies
- Project and Supply Chain Management
- Top 20 Project Management Case Studies
- 10 Innovative Supply Chain Projects
- Latest Management Project Topics
- 10 Project Management Project Ideas
- 6 Types of Supply Chain Models
- Top 10 Advantages of SCM
- Top 10 Supply Chain Books
- What is Project Description?
- Top 10 Project Management Companies
- Best Project Management Courses Online
- Salaries and Career Paths in Management
- Project Manager Salary in India
- Average Product Manager Salary India
- Supply Chain Management Salary India
- Salary After BBA in India
- PGDM Salary in India
- Top 7 Career Options in Management
- CSPO Certification Cost
- Why Choose Product Management?
- Product Management in Pharma
- Product Design in Operations Management
- Industry-Specific Management and Case Studies
- Amazon Business Case Study
- Service Delivery Manager Job
- Product Management Examples
- Product Management in Automobiles
- Product Management in Banking
- Sample SOP for Business Management
- Video Game Design Components
- Top 5 Business Courses India
- Free Management Online Course
- SCM Interview Q&A
- Fundamentals and Types of Law
- Acceptance in Contract Law
- Offer in Contract Law
- 9 Types of Evidence
- Types of Law in India
- Introduction to Contract Law
- Negotiable Instrument Act
- Corporate Tax Basics
- Intellectual Property Law
- Workmen Compensation Explained
- Lawyer vs Advocate Difference
- Law Education and Courses
- LLM Subjects & Syllabus
- Corporate Law Subjects
- LLM Course Duration
- Top 10 Online LLM Courses
- Online LLM Degree
- Step-by-Step Guide to Studying Law
- Top 5 Law Books to Read
- Why Legal Studies?
- Pursuing a Career in Law
- How to Become Lawyer in India
- Career Options and Salaries in Law
- Career Options in Law India
- Corporate Lawyer Salary India
- How To Become a Corporate Lawyer
- Career in Law: Starting, Salary
- Career Opportunities: Corporate Law
- Business Lawyer: Role & Salary Info
- Average Lawyer Salary India
- Top Career Options for Lawyers
- Types of Lawyers in India
- Steps to Become SC Lawyer in India
- Tutorials
- Software Tutorials
- C Tutorials
- Recursion in C: Fibonacci Series
- Checking String Palindromes in C
- Prime Number Program in C
- Implementing Square Root in C
- Matrix Multiplication in C
- Understanding Double Data Type
- Factorial of a Number in C
- Structure of a C Program
- Building a Calculator Program in C
- Compiling C Programs on Linux
- Java Tutorials
- Handling String Input in Java
- Determining Even and Odd Numbers
- Prime Number Checker
- Sorting a String
- User-Defined Exceptions
- Understanding the Thread Life Cycle
- Swapping Two Numbers
- Using Final Classes
- Area of a Triangle
- Skills
- Explore Skills
- Management Skills
- Software Engineering
- JavaScript
- Data Structure
- React.js
- Core Java
- Node.js
- Blockchain
- SQL
- Full stack development
- Devops
- NFT
- BigData
- Cyber Security
- Cloud Computing
- Database Design with MySQL
- Cryptocurrency
- Python
- Digital Marketings
- Advertising
- Influencer Marketing
- Performance Marketing
- Search Engine Marketing
- Email Marketing
- Content Marketing
- Social Media Marketing
- Display Advertising
- Marketing Analytics
- Web Analytics
- Affiliate Marketing
- MBA
- MBA in Finance
- MBA in HR
- MBA in Marketing
- MBA in Business Analytics
- MBA in Operations Management
- MBA in International Business
- MBA in Information Technology
- MBA in Healthcare Management
- MBA In General Management
- MBA in Agriculture
- MBA in Supply Chain Management
- MBA in Entrepreneurship
- MBA in Project Management
- Management Program
- Consumer Behaviour
- Supply Chain Management
- Financial Analytics
- Introduction to Fintech
- Introduction to HR Analytics
- Fundamentals of Communication
- Art of Effective Communication
- Introduction to Research Methodology
- Mastering Sales Technique
- Business Communication
- Fundamentals of Journalism
- Economics Masterclass
- Free Courses
- Home
- Blog
- Data Science
- Data Processing in Hadoop Ecosystem: Complete Data Flow Explained
Data Processing in Hadoop Ecosystem: Complete Data Flow Explained
Updated on Jan 28, 2025 | 14 min read
Share:
Table of Contents
With the exponential growth of the World Wide Web over the years, the data being generated also grew exponentially. This led to a massive amount of data being created and it was difficult to process and store this humungous amount of data with the traditional relational database systems.
Also, the data created was not only in the structured form but also in the unstructured format like videos, images, etc. Relational databases cannot process this kind of data. To counter these issues, Hadoop came into existence.
Before we dive into the data processing of Hadoop, let us have an overview of Hadoop and its components. Apache Hadoop is a framework that allows the storing and processing of huge quantities of data swiftly and efficiently. It can be used to store huge quantities of structured and unstructured data. Learn more about the Hadoop ecosystem and components.
Let’s begin with a workflow overview of data processing in Hadoop.
Data Processing in Hadoop: Complete Workflow
Data processing in Hadoop follows a structured flow that ensures large datasets are efficiently processed across distributed systems. The process starts with raw data being divided into smaller chunks, processed in parallel, and finally aggregated to generate meaningful output.
Understanding this step-by-step workflow is essential to optimizing performance and managing large-scale data effectively.
InputSplit and RecordReader
Before processing begins, Hadoop logically divides the dataset into manageable parts. This ensures that data is efficiently read and distributed across the cluster, optimizing resource utilization and parallel execution. Logical splitting prevents unnecessary data fragmentation, reducing processing overhead and enhancing cluster efficiency.
Below is how it works:
- InputSplit divides data into logical chunks: These chunks do not physically split files but create partitions for parallel execution. The size of each split depends on the HDFS block size (default 128MB) and can be customized based on cluster configuration. For example, a 10GB file might be divided into five 2GB splits, allowing multiple nodes to process different parts simultaneously without excessive disk seeks.
- RecordReader converts InputSplit data into key-value pairs: Hadoop processes data in key-value pairs. The RecordReader reads raw data from an InputSplit and structures it for the mapper. For instance, in a text processing job, it may convert each line into a key-value pair where the key is the byte offset and the value is the line content.
Also Read: Hadoop YARN Architecture: Comprehensive Guide to YARN Components and Functionality
With data split into smaller logical units and structured into key-value pairs, the next step involves processing this data to extract meaningful information. This is where the mapper and combiner come into play.
Mapper and Combiner
Once data is split and formatted, it enters the mapper phase. The mapper plays a critical role in processing and transforming input data before passing it to the next stage.A combiner, an optional step, optimizes performance by reducing data locally, minimizing the volume of intermediate data that needs to be transferred to reducers.
Below is how this stage functions:
- The mapper processes each key-value pair and transforms it: It extracts meaningful information by applying logic on input data. For example, in a word count program, the mapper receives lines of text, breaks them into words, and assigns each word a count of one. If the input is "Hadoop is powerful. Hadoop is scalable.", the mapper emits key-value pairs like ("Hadoop", 1), ("is", 1), ("powerful", 1), and so on.
- The combiner performs local aggregation to reduce intermediate data: Since mappers generate large amounts of intermediate data, the combiner helps by merging values locally before sending them to reducers. For example, in the same word count program, if the mapper processes 1,000 occurrences of "Hadoop" across different InputSplits, the combiner sums up word counts locally, reducing multiple ("Hadoop", 1) pairs to a single ("Hadoop", 1000) before the shuffle phase. This minimizes data transfer and speeds up processing.
Also Read: Top 10 Hadoop Commands [With Usages]
Now that the data has been processed and locally aggregated, it needs to be efficiently distributed to ensure balanced workload distribution. The partitioner and shuffle step handle this crucial process. Let’s take a close look in the next section.
Partitioner and Shuffle
Once the mapper and optional combiner complete processing, data must be organized efficiently before reaching the reducer. The partitioner and shuffle phase ensures a smooth and evenly distributed data flow in Hadoop.
- The partitioner assigns key-value pairs to reducers based on keys – It ensures that related data reaches the same reducer. For example, in a word count job, words starting with 'A' may go to Reducer 1, while words starting with 'B' go to Reducer 2.
- Shuffling transfers and sorts intermediate data before reduction – After partitioning, data is shuffled across nodes, sorting it by key. This ensures that all values associated with the same key are sent to the same reducer. It prevents duplicate processing by grouping related data before reduction. For instance, if "Hadoop" appears in multiple splits, all occurrences are grouped together before reaching the reducer.
With data properly partitioned and transferred to reducers, the final stage focuses on aggregation and output formatting. This ensures that the results are structured and stored appropriately for further analysis.
Reducer and OutputFormat
The reducer aggregates and finalizes data, producing the final output. The OutputFormat ensures that processed data is stored in the required format for further use, offering flexibility for integration with various systems.
Below is how this stage works:
- The reducer processes grouped key-value pairs and applies aggregation logic: It takes data from multiple mappers, processes it, and generates the final output. For example, in a word count program, if the word "Hadoop" appears 1,500 times across different splits, the reducer sums up all occurrences and outputs "Hadoop: 1500".
- OutputFormat determines how final data is stored and structured: Hadoop provides built-in options like TextOutputFormat, SequenceFileOutputFormat, and AvroOutputFormat, allowing data to be stored in various formats. Additionally, custom OutputFormats can be defined for specific needs, such as structured storage in databases or exporting results in JSON for log processing jobs. This flexibility allows seamless integration with data lakes, BI tools, and analytics platforms.
With the entire data flow in Hadoop completed, it’s important to understand the essential components that power this ecosystem. These building blocks ensure efficient data storage, processing, and retrieval.
What are the Building Blocks of Hadoop?
Hadoop’s ecosystem is built on several essential components that work together to enable efficient data storage, processing, and management. These building blocks ensure that large datasets are processed in a distributed manner, allowing organizations to handle massive volumes of structured and unstructured data.
Let’s explore the building blocks in detail.
1. HDFS (The Storage Layer)
As the name suggests, Hadoop Distributed File System is the storage layer of Hadoop and is responsible for storing the data in a distributed environment (master and slave configuration). It splits the data into several blocks of data and stores them across different data nodes. These data blocks are also replicated across different data nodes to prevent loss of data when one of the nodes goes down.
It has two main processes running for processing of the data: –
a. NameNode
It is running on the master machine. It saves the locations of all the files stored in the file system and tracks where the data resides across the cluster i.e. it stores the metadata of the files. When the client applications want to make certain operations on the data, it interacts with the NameNode. When the NameNode receives the request, it responds by returning a list of Data Node servers where the required data resides.
b. DataNode
This process runs on every slave machine. One of its functionalities is to store each HDFS data block in a separate file in its local file system. In other words, it contains the actual data in form of blocks. It sends heartbeat signals periodically and waits for the request from the NameNode to access the data.
2. MapReduce (The processing layer)
It is a programming technique based on Java that is used on top of the Hadoop framework for faster processing of huge quantities of data. It processes this huge data in a distributed environment using many Data Nodes which enables parallel processing and faster execution of operations in a fault-tolerant way.
A MapReduce job splits the data set into multiple chunks of data which are further converted into key-value pairs in order to be processed by the mappers. The raw format of the data may not be suitable for processing. Thus, the input data compatible with the map phase is generated using the InputSplit function and RecordReader.
InputSplit is the logical representation of the data which is to be processed by an individual mapper. RecordReader converts these splits into records which take the form of key-value pairs. It basically converts the byte-oriented representation of the input into a record-oriented representation.
These records are then fed to the mappers for further processing the data. MapReduce jobs primarily consist of three phases namely the Map phase, the Shuffle phase, and the Reduce phase.
a. Map Phase
It is the first phase in the processing of the data. The main task in the map phase is to process each input from the RecordReader and convert it into intermediate tuples (key-value pairs). This intermediate output is stored in the local disk by the mappers.
The values of these key-value pairs can differ from the ones received as input from the RecordReader. The map phase can also contain combiners which are also called as local reducers. They perform aggregations on the data but only within the scope of one mapper.
As the computations are performed across different data nodes, it is essential that all the values associated with the same key are merged together into one reducer. This task is performed by the partitioner. It performs a hash function over these key-value pairs to merge them together.
It also ensures that all the tasks are partitioned evenly to the reducers. Partitioners generally come into the picture when we are working with more than one reducer.
b. Shuffle and Sort Phase
This phase transfers the intermediate output obtained from the mappers to the reducers. This process is called shuffling. The output from the mappers is also sorted before transferring it to the reducers. The sorting is done on the basis of the keys in the key-value pairs. It helps the reducers to perform the computations on the data even before the entire data is received and eventually helps in reducing the time required for computations.
As the keys are sorted, whenever the reducer gets a different key as the input it starts to perform the reduced tasks on the previously received data.
c. Reduce Phase
The output of the map phase serves as an input to the reduce phase. It takes these key-value pairs and applies the reduce function on them to produce the desired result. The keys and the values associated with the key are passed on to the reduce function to perform certain operations.
We can filter the data or combine it to obtain the aggregated output. Post the execution of the reduce function, it can create zero or more key-value pairs. This result is written back in the Hadoop Distributed File System.
3. YARN (The Management Layer)
Yet Another Resource Navigator is the resource managing component of Hadoop. There are background processes running at each node (Node Manager on the slave machines and Resource Manager on the master node) that communicate with each other for the allocation of resources. The Resource Manager is the centrepiece of the YARN layer which manages resources among all the applications and passes on the requests to the Node Manager.
The Node Manager monitors the resource utilization like memory, CPU, and disk of the machine and conveys the same to the Resource Manager. It is installed on every Data Node and is responsible for executing the tasks on the Data Nodes.
From distributed storage to parallel data processing, each component plays a key role in maintaining a smooth data flow in Hadoop. The next section explores the key benefits of this data flow and its real-world applications.
Key Benefits and Use Cases of Data Flow in Hadoop
Hadoop enables scalable, cost-effective, and fault-tolerant data processing across distributed environments. Below are some of the major benefits and practical use cases of data processing in Hadoop.
- Scalability for large-scale data processing – Hadoop’s distributed architecture allows you to scale storage and processing power horizontally. For example, companies handling petabytes of data, such as Facebook and Twitter, use Hadoop clusters to process user interactions, ad targeting, and recommendation algorithms.
- Cost-effective storage and processing – Traditional relational databases can be expensive for storing and processing big data. Hadoop provides a low-cost alternative by using commodity hardware, making it ideal for businesses dealing with vast data volumes. A retail giant like Walmart, for instance, uses Hadoop to analyze consumer purchasing behavior at scale.
- Fault tolerance and high availability – Hadoop replicates data across multiple nodes, ensuring no single point of failure. If one node fails, another automatically takes over, maintaining uninterrupted processing. This feature is critical for financial institutions that cannot afford data loss or downtime.
- Efficient handling of structured and unstructured data – Unlike traditional databases that struggle with unstructured data, Hadoop seamlessly processes images, videos, social media posts, and sensor data. Companies like Netflix rely on Hadoop to analyze streaming preferences and enhance user recommendations.
- Real-time and batch data processing – Hadoop supports both real-time and batch processing through its ecosystem tools like Apache Spark and MapReduce. This flexibility is essential for cybersecurity firms detecting fraudulent transactions in real-time while also analyzing historical trends.
- Optimized data flow for analytics and machine learning – With frameworks like Apache Mahout and Spark MLlib, Hadoop simplifies large-scale machine learning applications. Organizations use this capability to build predictive models for healthcare, finance, and e-commerce.
- Real-time fraud detection in financial services – Hadoop enables banks and financial institutions to detect fraudulent transactions in real time by analyzing large volumes of customer transaction data. PayPal and Mastercard use Hadoop to identify anomalies and trigger fraud alerts within milliseconds.
- IoT data processing in smart cities – Hadoop helps smart cities process vast IoT sensor data from traffic systems, surveillance cameras, and environmental monitors. Barcelona and Singapore use Hadoop-based analytics to optimize urban planning, reduce congestion, and improve public safety.
Also Read: Top 10 Hadoop Tools to Make Your Big Data Journey Easy
With its efficient data flow, Hadoop is a cornerstone for businesses aiming to manage vast amounts of information. Understanding how to work with Hadoop clusters can significantly improve your ability to handle big data challenges. The next section explores how upGrad can help you gain expertise in this field.
How upGrad Can Help You Excel Hadoop Clusters in Big Data?
upGrad is a leading online learning platform with over 10 million learners, 200+ courses, and 1400+ hiring partners. Whether you want to master Hadoop, advance your data engineering skills, or explore big data technologies, upGrad provides industry-relevant courses designed for career growth.
Below are some top courses that can help you gain expertise in data flow in Hadoop and big data processing.
Boost your career with our popular Software Engineering courses, offering hands-on training and expert guidance to turn you into a skilled software developer.
Explore our Popular Software Engineering Courses
Master in-demand Software Development skills like coding, system design, DevOps, and agile methodologies to excel in today’s competitive tech industry.
In-Demand Software Development Skills
Stay informed with our widely-read Software Development articles, covering everything from coding techniques to the latest advancements in software engineering.
Read our Popular Articles related to Software
Frequently Asked Questions (FAQs)
1. Between Hadoop and MapReduce, which one is a better choice?
2. What is the hardware configuration of Namenode and Datanode?
3. What are some of the techniques for MapReduce job optimization?
4. How Does Hadoop Ensure Data Security?
5. What Is the Role of YARN in Hadoop?
6. How Does Hadoop Handle Data Skew?
7. What Are the Challenges of Small Files in HDFS?
8. How Does Hadoop Integrate with Cloud Services?
9. What Is the Function of the Secondary NameNode?
10. How Does Hadoop Achieve Fault Tolerance?
11. What Are the Limitations of Hadoop's MapReduce?
Get Free Consultation
By submitting, I accept the T&C and
Privacy Policy
Start Your Career in Data Science Today
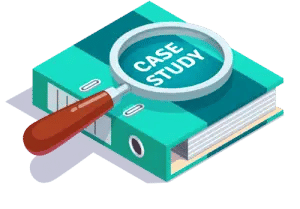
Top Resources