- Blog Categories
- Software Development
- Data Science
- AI/ML
- Marketing
- General
- MBA
- Management
- Legal
- Software Development Projects and Ideas
- 12 Computer Science Project Ideas
- 28 Beginner Software Projects
- Top 10 Engineering Project Ideas
- Top 10 Easy Final Year Projects
- Top 10 Mini Projects for Engineers
- 25 Best Django Project Ideas
- Top 20 MERN Stack Project Ideas
- Top 12 Real Time Projects
- Top 6 Major CSE Projects
- 12 Robotics Projects for All Levels
- Java Programming Concepts
- Abstract Class in Java and Methods
- Constructor Overloading in Java
- StringBuffer vs StringBuilder
- Java Identifiers: Syntax & Examples
- Types of Variables in Java Explained
- Composition in Java: Examples
- Append in Java: Implementation
- Loose Coupling vs Tight Coupling
- Integrity Constraints in DBMS
- Different Types of Operators Explained
- Career and Interview Preparation in IT
- Top 14 IT Courses for Jobs
- Top 20 Highest Paying Languages
- 23 Top CS Interview Q&A
- Best IT Jobs without Coding
- Software Engineer Salary in India
- 44 Agile Methodology Interview Q&A
- 10 Software Engineering Challenges
- Top 15 Tech's Daily Life Impact
- 10 Best Backends for React
- Cloud Computing Reference Models
- Web Development and Security
- Find Installed NPM Version
- Install Specific NPM Package Version
- Make API Calls in Angular
- Install Bootstrap in Angular
- Use Axios in React: Guide
- StrictMode in React: Usage
- 75 Cyber Security Research Topics
- Top 7 Languages for Ethical Hacking
- Top 20 Docker Commands
- Advantages of OOP
- Data Science Projects and Applications
- 42 Python Project Ideas for Beginners
- 13 Data Science Project Ideas
- 13 Data Structure Project Ideas
- 12 Real-World Python Applications
- Python Banking Project
- Data Science Course Eligibility
- Association Rule Mining Overview
- Cluster Analysis in Data Mining
- Classification in Data Mining
- KDD Process in Data Mining
- Data Structures and Algorithms
- Binary Tree Types Explained
- Binary Search Algorithm
- Sorting in Data Structure
- Binary Tree in Data Structure
- Binary Tree vs Binary Search Tree
- Recursion in Data Structure
- Data Structure Search Methods: Explained
- Binary Tree Interview Q&A
- Linear vs Binary Search
- Priority Queue Overview
- Python Programming and Tools
- Top 30 Python Pattern Programs
- List vs Tuple
- Python Free Online Course
- Method Overriding in Python
- Top 21 Python Developer Skills
- Reverse a Number in Python
- Switch Case Functions in Python
- Info Retrieval System Overview
- Reverse a Number in Python
- Real-World Python Applications
- Data Science Careers and Comparisons
- Data Analyst Salary in India
- Data Scientist Salary in India
- Free Excel Certification Course
- Actuary Salary in India
- Data Analyst Interview Guide
- Pandas Interview Guide
- Tableau Filters Explained
- Data Mining Techniques Overview
- Data Analytics Lifecycle Phases
- Data Science Vs Analytics Comparison
- Artificial Intelligence and Machine Learning Projects
- Exciting IoT Project Ideas
- 16 Exciting AI Project Ideas
- 45+ Interesting ML Project Ideas
- Exciting Deep Learning Projects
- 12 Intriguing Linear Regression Projects
- 13 Neural Network Projects
- 5 Exciting Image Processing Projects
- Top 8 Thrilling AWS Projects
- 12 Engaging AI Projects in Python
- NLP Projects for Beginners
- Concepts and Algorithms in AIML
- Basic CNN Architecture Explained
- 6 Types of Regression Models
- Data Preprocessing Steps
- Bagging vs Boosting in ML
- Multinomial Naive Bayes Overview
- Bayesian Network Example
- Bayes Theorem Guide
- Top 10 Dimensionality Reduction Techniques
- Neural Network Step-by-Step Guide
- Technical Guides and Comparisons
- Make a Chatbot in Python
- Compute Square Roots in Python
- Permutation vs Combination
- Image Segmentation Techniques
- Generative AI vs Traditional AI
- AI vs Human Intelligence
- Random Forest vs Decision Tree
- Neural Network Overview
- Perceptron Learning Algorithm
- Selection Sort Algorithm
- Career and Practical Applications in AIML
- AI Salary in India Overview
- Biological Neural Network Basics
- Top 10 AI Challenges
- Production System in AI
- Top 8 Raspberry Pi Alternatives
- Top 8 Open Source Projects
- 14 Raspberry Pi Project Ideas
- 15 MATLAB Project Ideas
- Top 10 Python NLP Libraries
- Naive Bayes Explained
- Digital Marketing Projects and Strategies
- 10 Best Digital Marketing Projects
- 17 Fun Social Media Projects
- Top 6 SEO Project Ideas
- Digital Marketing Case Studies
- Coca-Cola Marketing Strategy
- Nestle Marketing Strategy Analysis
- Zomato Marketing Strategy
- Monetize Instagram Guide
- Become a Successful Instagram Influencer
- 8 Best Lead Generation Techniques
- Digital Marketing Careers and Salaries
- Digital Marketing Salary in India
- Top 10 Highest Paying Marketing Jobs
- Highest Paying Digital Marketing Jobs
- SEO Salary in India
- Content Writer Salary Guide
- Digital Marketing Executive Roles
- Career in Digital Marketing Guide
- Future of Digital Marketing
- MBA in Digital Marketing Overview
- Digital Marketing Techniques and Channels
- 9 Types of Digital Marketing Channels
- Top 10 Benefits of Marketing Branding
- 100 Best YouTube Channel Ideas
- YouTube Earnings in India
- 7 Reasons to Study Digital Marketing
- Top 10 Digital Marketing Objectives
- 10 Best Digital Marketing Blogs
- Top 5 Industries Using Digital Marketing
- Growth of Digital Marketing in India
- Top Career Options in Marketing
- Interview Preparation and Skills
- 73 Google Analytics Interview Q&A
- 56 Social Media Marketing Q&A
- 78 Google AdWords Interview Q&A
- Top 133 SEO Interview Q&A
- 27+ Digital Marketing Q&A
- Digital Marketing Free Course
- Top 9 Skills for PPC Analysts
- Movies with Successful Social Media Campaigns
- Marketing Communication Steps
- Top 10 Reasons to Be an Affiliate Marketer
- Career Options and Paths
- Top 25 Highest Paying Jobs India
- Top 25 Highest Paying Jobs World
- Top 10 Highest Paid Commerce Job
- Career Options After 12th Arts
- Top 7 Commerce Courses Without Maths
- Top 7 Career Options After PCB
- Best Career Options for Commerce
- Career Options After 12th CS
- Top 10 Career Options After 10th
- 8 Best Career Options After BA
- Projects and Academic Pursuits
- 17 Exciting Final Year Projects
- Top 12 Commerce Project Topics
- Top 13 BCA Project Ideas
- Career Options After 12th Science
- Top 15 CS Jobs in India
- 12 Best Career Options After M.Com
- 9 Best Career Options After B.Sc
- 7 Best Career Options After BCA
- 22 Best Career Options After MCA
- 16 Top Career Options After CE
- Courses and Certifications
- 10 Best Job-Oriented Courses
- Best Online Computer Courses
- Top 15 Trending Online Courses
- Top 19 High Salary Certificate Courses
- 21 Best Programming Courses for Jobs
- What is SGPA? Convert to CGPA
- GPA to Percentage Calculator
- Highest Salary Engineering Stream
- 15 Top Career Options After Engineering
- 6 Top Career Options After BBA
- Job Market and Interview Preparation
- Why Should You Be Hired: 5 Answers
- Top 10 Future Career Options
- Top 15 Highest Paid IT Jobs India
- 5 Common Guesstimate Interview Q&A
- Average CEO Salary: Top Paid CEOs
- Career Options in Political Science
- Top 15 Highest Paying Non-IT Jobs
- Cover Letter Examples for Jobs
- Top 5 Highest Paying Freelance Jobs
- Top 10 Highest Paying Companies India
- Career Options and Paths After MBA
- 20 Best Careers After B.Com
- Career Options After MBA Marketing
- Top 14 Careers After MBA In HR
- Top 10 Highest Paying HR Jobs India
- How to Become an Investment Banker
- Career Options After MBA - High Paying
- Scope of MBA in Operations Management
- Best MBA for Working Professionals India
- MBA After BA - Is It Right For You?
- Best Online MBA Courses India
- MBA Project Ideas and Topics
- 11 Exciting MBA HR Project Ideas
- Top 15 MBA Project Ideas
- 18 Exciting MBA Marketing Projects
- MBA Project Ideas: Consumer Behavior
- What is Brand Management?
- What is Holistic Marketing?
- What is Green Marketing?
- Intro to Organizational Behavior Model
- Tech Skills Every MBA Should Learn
- Most Demanding Short Term Courses MBA
- MBA Salary, Resume, and Skills
- MBA Salary in India
- HR Salary in India
- Investment Banker Salary India
- MBA Resume Samples
- Sample SOP for MBA
- Sample SOP for Internship
- 7 Ways MBA Helps Your Career
- Must-have Skills in Sales Career
- 8 Skills MBA Helps You Improve
- Top 20+ SAP FICO Interview Q&A
- MBA Specializations and Comparative Guides
- Why MBA After B.Tech? 5 Reasons
- How to Answer 'Why MBA After Engineering?'
- Why MBA in Finance
- MBA After BSc: 10 Reasons
- Which MBA Specialization to choose?
- Top 10 MBA Specializations
- MBA vs Masters: Which to Choose?
- Benefits of MBA After CA
- 5 Steps to Management Consultant
- 37 Must-Read HR Interview Q&A
- Fundamentals and Theories of Management
- What is Management? Objectives & Functions
- Nature and Scope of Management
- Decision Making in Management
- Management Process: Definition & Functions
- Importance of Management
- What are Motivation Theories?
- Tools of Financial Statement Analysis
- Negotiation Skills: Definition & Benefits
- Career Development in HRM
- Top 20 Must-Have HRM Policies
- Project and Supply Chain Management
- Top 20 Project Management Case Studies
- 10 Innovative Supply Chain Projects
- Latest Management Project Topics
- 10 Project Management Project Ideas
- 6 Types of Supply Chain Models
- Top 10 Advantages of SCM
- Top 10 Supply Chain Books
- What is Project Description?
- Top 10 Project Management Companies
- Best Project Management Courses Online
- Salaries and Career Paths in Management
- Project Manager Salary in India
- Average Product Manager Salary India
- Supply Chain Management Salary India
- Salary After BBA in India
- PGDM Salary in India
- Top 7 Career Options in Management
- CSPO Certification Cost
- Why Choose Product Management?
- Product Management in Pharma
- Product Design in Operations Management
- Industry-Specific Management and Case Studies
- Amazon Business Case Study
- Service Delivery Manager Job
- Product Management Examples
- Product Management in Automobiles
- Product Management in Banking
- Sample SOP for Business Management
- Video Game Design Components
- Top 5 Business Courses India
- Free Management Online Course
- SCM Interview Q&A
- Fundamentals and Types of Law
- Acceptance in Contract Law
- Offer in Contract Law
- 9 Types of Evidence
- Types of Law in India
- Introduction to Contract Law
- Negotiable Instrument Act
- Corporate Tax Basics
- Intellectual Property Law
- Workmen Compensation Explained
- Lawyer vs Advocate Difference
- Law Education and Courses
- LLM Subjects & Syllabus
- Corporate Law Subjects
- LLM Course Duration
- Top 10 Online LLM Courses
- Online LLM Degree
- Step-by-Step Guide to Studying Law
- Top 5 Law Books to Read
- Why Legal Studies?
- Pursuing a Career in Law
- How to Become Lawyer in India
- Career Options and Salaries in Law
- Career Options in Law India
- Corporate Lawyer Salary India
- How To Become a Corporate Lawyer
- Career in Law: Starting, Salary
- Career Opportunities: Corporate Law
- Business Lawyer: Role & Salary Info
- Average Lawyer Salary India
- Top Career Options for Lawyers
- Types of Lawyers in India
- Steps to Become SC Lawyer in India
- Tutorials
- Software Tutorials
- C Tutorials
- Recursion in C: Fibonacci Series
- Checking String Palindromes in C
- Prime Number Program in C
- Implementing Square Root in C
- Matrix Multiplication in C
- Understanding Double Data Type
- Factorial of a Number in C
- Structure of a C Program
- Building a Calculator Program in C
- Compiling C Programs on Linux
- Java Tutorials
- Handling String Input in Java
- Determining Even and Odd Numbers
- Prime Number Checker
- Sorting a String
- User-Defined Exceptions
- Understanding the Thread Life Cycle
- Swapping Two Numbers
- Using Final Classes
- Area of a Triangle
- Skills
- Explore Skills
- Management Skills
- Software Engineering
- JavaScript
- Data Structure
- React.js
- Core Java
- Node.js
- Blockchain
- SQL
- Full stack development
- Devops
- NFT
- BigData
- Cyber Security
- Cloud Computing
- Database Design with MySQL
- Cryptocurrency
- Python
- Digital Marketings
- Advertising
- Influencer Marketing
- Performance Marketing
- Search Engine Marketing
- Email Marketing
- Content Marketing
- Social Media Marketing
- Display Advertising
- Marketing Analytics
- Web Analytics
- Affiliate Marketing
- MBA
- MBA in Finance
- MBA in HR
- MBA in Marketing
- MBA in Business Analytics
- MBA in Operations Management
- MBA in International Business
- MBA in Information Technology
- MBA in Healthcare Management
- MBA In General Management
- MBA in Agriculture
- MBA in Supply Chain Management
- MBA in Entrepreneurship
- MBA in Project Management
- Management Program
- Consumer Behaviour
- Supply Chain Management
- Financial Analytics
- Introduction to Fintech
- Introduction to HR Analytics
- Fundamentals of Communication
- Art of Effective Communication
- Introduction to Research Methodology
- Mastering Sales Technique
- Business Communication
- Fundamentals of Journalism
- Economics Masterclass
- Free Courses
- Home
- Blog
- Data Science
- Fake News Detection Project in Python [With Coding]
Fake News Detection Project in Python [With Coding]
Updated on Mar 01, 2024 | 12 min read
Share:
Ever read a piece of news which just seems bogus? We all encounter such news articles, and instinctively recognise that something doesn’t feel right. Because of so many posts out there, it is nearly impossible to separate the right from the wrong. Here, we are not only talking about spurious claims and the factual points, but rather, the things which look wrong intricately in the language itself.
Did you ever wonder how to develop a fake news detection project? But there is no easy way out to find which news is fake and which is not, especially these days, with the speed of spread of news on social media. Still, some solutions could help out in identifying these wrongdoings.
There are two ways of claiming that some news is fake or not: First, an attack on the factual points. Second, the language. The former can only be done through substantial searches into the internet with automated query systems. It could be an overwhelming task, especially for someone who is just getting started with data science and natural language processing.
The latter is possible through a natural language processing pipeline followed by a machine learning pipeline. It is how we would implement our fake news detection project in Python. It is another one of the problems that are recognized as a machine learning problem posed as a natural language processing problem. If you are a beginner and interested to learn more about data science, check out our data science online courses from top universities.
Read: Python Project Ideas & Topics
This article will briefly discuss a fake news detection project with a fake news detection code. On that note, the fake news detection final year project is a great way of adding weight to your resume, as the number of imposter emails, texts and websites are continuously growing and distorting particular issue or individual. Hence, fake news detection using Python can be a great way of providing a meaningful solution to real-time issues while showcasing your programming language abilities.
There are many datasets out there for this type of application, but we would be using the one mentioned here. The data contains about 7500+ news feeds with two target labels: fake or real. The dataset also consists of the title of the specific news piece.
The steps in the pipeline for natural language processing would be as follows:
- Acquiring and loading the data
- Cleaning the dataset
- Removing extra symbols
- Removing punctuations
- Removing the stopwords
- Stemming
- Tokenization
- Feature extractions
- TF-IDF vectorizer
- Counter vectorizer with TF-IDF transformer
- Machine learning model training and verification
Before we start discussing the implementation steps of the fake news detection project, let us import the necessary libraries:
Basic Working of the Fake News Detection Project
Just knowing the fake news detection code will not be enough for you to get an overview of the project, hence, learning the basic working mechanism can be helpful. So here’s the in-depth elaboration of the fake news detection final year project.
The project’s main focus is at its front end as the users will be uploading the URL of the news website whose authenticity they want to check. This entered URL is then sent to the backend of the software/ website, where some predictive feature of machine learning will be used to check the URL’s credibility.
In this entire authentication process of fake news detection using Python, the software will crawl the contents of the given web page, and a feature for storing the crawled data will be there. However, the data could only be stored locally. Then the crawled data will be sent for development and analysis for future prediction. This will be performed with the help of the SQLite database. The flask platform can be used to build the backend.
Therefore, once the front end receives the data, it will be sent to the backend, and the predicted authentication result will be displayed on the user’s screen.
The basic working of the backend part is composed of two elements: web crawling and the voting mechanism.
The very first step of web crawling will be to extract the headline from the URL by downloading its HTML. Then, the “Title” tags are found, and their HTML is downloaded. At the same time, the body content will also be examined by using tags of HTML code. Elements such as keywords, word frequency, etc., are judged. The python library named “newspaper” is a great tool for extracting keywords.
The majority-voting scheme seemed the best-suited one for this project, with a wide range of classification models. In this scheme, the given news will be classified as real or fake based on the major votes it gets from the models.
Check out the trending Python Tutorial concepts in 2024
upGrad’s Exclusive Data Science Webinar for you –
Transformation & Opportunities in Analytics & Insights
Explore our Popular Data Science Courses
Front End and Back End Elements:
The elements used for the front-end development of the fake news detection project include
- HTML (Hyper Text Markup Language)
- CSS (Cascading Style Sheets)
- JAVASCRIPT
Back end building elements
- SVM (Support Vector Machine)
- Decision Tree
- Random Forest (Decision tree version)
- Random Forest (Random tree version)
Code:
import pandas as pd
import numpy as np
import re
import string
from nltk.corpus import stopwords
from nltk.tokenize import word_tokenize
stop_words = set(stopwords.words(‘english’))
The first step is to acquire the data. We have already provided the link to the CSV file; but, it is also crucial to discuss the other way to generate your data. Therefore, in a fake news detection project documentation plays a vital role. The fake news detection project can be executed both in the form of a web-based application or a browser extension.
One of the methods is web scraping. For this, we need to code a web crawler and specify the sites from which you need to get the data. But be careful, there are two problems with this approach.
First, it may be illegal to scrap many sites, so you need to take care of that. And second, the data would be very raw. The whole pipeline would be appended with a list of steps to convert that raw data into a workable CSV file or dataset. Hence, we use the pre-set CSV file with organised data.
Even the fake news detection in Python relies on human-created data to be used as reliable or fake. Therefore, we have to list at least 25 reliable news sources and a minimum of 750 fake news websites to create the most efficient fake news detection project documentation. These websites will be crawled, and the gathered information will be stored in the local machine for additional processing.
The processing may include URL extraction, author analysis, and similar steps. Then with the help of a Recurrent Neural Network (RNN), data classification or prediction will be applied to the back end server.
Therefore it is fair to say that fake news detection in Python has a very simple mechanism where the user would enter the URL of the article they want to check the authenticity in the website’s front end, and the web front end will notify them about the credibility of the source.
Read our popular Data Science Articles
However, if interested, you can check out upGrad’s course on Data science, in which there are enough resources available with proper explanations on Data engineering and web scraping. Do make sure to check those out here.
Below is the detailed discussion with all the dos and don’ts on fake news detection using machine learning source code.
It is how we import our dataset and append the labels. Do note how we drop the unnecessary columns from the dataset. If required on a higher value, you can keep those columns up. But right now, our fake news detection project would work smoothly on just the text and target label columns.
Code:
df_text = pd.read_csv(‘fake_or_real_news.csv’, encoding=‘latin-1’)
df_text.columns = [‘id’, ‘title’, ‘text’, ‘label’]df_text.drop([‘id’, ‘title’], axis=1)
Moving on, the next step from fake news detection using machine learning source code is to clean the existing data. Why is this step necessary? It is crucial to understand that we are working with a machine and teaching it to bifurcate the fake and the real. Right now, we have textual data, but computers work on numbers. So first is required to convert them to numbers, and a step before that is to make sure we are only transforming those texts which are necessary for the understanding.
The first step in the cleaning pipeline is to check if the dataset contains any extra symbols to clear away. It could be web addresses or any of the other referencing symbol(s), like at(@) or hashtags. Here is the code:
Code:
# Remove urls
text = re.sub(r”http\S+|www\S+|https\S+”, ”, text, flags=re.MULTILINE)
# Remove user @ references and ‘#’ from text
text = re.sub(r’\@\w+|\#’,”, text)
Once we remove that, the next step is to clear away the other symbols: the punctuations. If we think about it, the punctuations have no clear input in understanding the reality of particular news. Sometimes, it may be possible that if there are a lot of punctuations, then the news is not real, for example, overuse of exclamations.
But those are rare cases and would require specific rule-based analysis. So, for this fake news detection project, we would be removing the punctuations. Here is how to do it:
Code:
text = text.translate(str.maketrans(”, ”, string.punctuation))
The next step is to stem the word to its core and tokenize the words. Tokenization means to make every sentence into a list of words or tokens. Here is a two-line code which needs to be appended:
Code:
tokens = word_tokenize(text)
words = [w for w in tokens if not w in stop_words]
The next step is a crucial one. The conversion of tokens into meaningful numbers. This step is also known as feature extraction. For our application, we are going with the TF-IDF method to extract and build the features for our machine learning pipeline.
TF-IDF essentially means term frequency-inverse document frequency. As suggested by the name, we scoop the information about the dataset via its frequency of terms as well as the frequency of terms in the entire dataset, or collection of documents.
TF-IDF can easily be calculated by mixing both values of TF and IDF. Both formulas involve simple ratios.
TF = no. of times the term appears in the document / total number of terms.
IDF = log of ( total no. of documents / no. of documents in which the term appears )
Now Python has two implementations for the TF-IDF conversion. First is a TF-IDF vectoriser and second is the TF-IDF transformer. The difference is that the transformer requires a bag-of-words implementation before the transformation, while the vectoriser combines both the steps into one. We could also use the count vectoriser that is a simple implementation of bag-of-words. But the TF-IDF would work better on the particular dataset.
Top Data Science Skills to Learn to upskill
SL. No | Top Data Science Skills to Learn | |
1 |
Data Analysis Online Courses | Inferential Statistics Online Courses |
2 |
Hypothesis Testing Online Courses | Logistic Regression Online Courses |
3 |
Linear Regression Courses | Linear Algebra for Analysis Online Courses |
Here is how TF-IDF could be implemented.
Code:
from sklearn.feature_extraction.text import TfidfVectorizer
tf_vector = TfidfVectorizer(sublinear_tf=True)
tf_vector.fit(df_text[‘text’])
The next step is the Machine learning pipeline. Just like the typical ML pipeline, we need to get the data into X and y. To do so, we use X as the matrix provided as an output by the TF-IDF vectoriser, which needs to be flattened.
We can use the travel function in Python to convert the matrix into an array. The y values cannot be directly appended as they are still labels and not numbers. To convert them to 0s and 1s, we use sklearn’s label encoder.
This encoder transforms the label texts into numbered targets. For example, assume that we have a list of labels like this: [‘real’, ‘fake’, ‘fake’, ‘fake’]
What we essentially require is a list like this: [1, 0, 0, 0]. What label encoder does is, it takes all the distinct labels and makes a list. For our example, the list would be [‘fake’, ‘real’].
Here is the implementation of the same:
Code:
X_text = tf_vector.transform(df_text[‘text’].ravel())
y_values = np.array(df_text[‘label’].ravel())
from sklearn import preprocessing
le = preprocessing.LabelEncoder()
le.fit(y_values)le.transform(y_values)
Once done, the training and testing splits are done. It can be achieved by using sklearn’s preprocessing package and importing the train test split function.
Code:
from sklearn.model_selection import train_test_split
X_train, X_test, y_train, y_test = train_test_split(X_text, y_values, test_size=0.15, random_state=120)
The final step is to use the models. There are many good machine learning models available, but even the simple base models would work well on our implementation of fake news detection projects. We first implement a logistic regression model. Here is how to implement using sklearn.
Our learners also read: Top Python Courses for Free
Code:
from sklearn.metrics import accuracy_score
from sklearn.linear_model import LogisticRegression
model = LogisticRegression(solver=‘lbfgs’)
model.fit(X_train, y_train)
y_predict = model.predict(X_test)
print(accuracy_score(y_test, y_predict))
The model performs pretty well. A 92 percent accuracy on a regression model is pretty decent. You can also implement other models available and check the accuracies.
So, this is how you can implement a fake news detection project using Python.
Note that there are many things to do here. The NLP pipeline is not yet fully complete. There are many other functions available which can be applied to get even better feature extractions.
Also Read: Python Open Source Project Ideas
The way fake news is adapting technology, better and better processing models would be required. And these models would be more into natural language understanding and less posed as a machine learning model itself. The models can also be fine-tuned according to the features used. The dataset could be made dynamically adaptable to make it work on current data. But that would require a model exhaustively trained on the current news articles.
So, if more data is available, better models could be made and the applicability of fake news detection projects can be improved. But the internal scheme and core pipelines would remain the same. The pipelines explained are highly adaptable to any experiments you may want to conduct. Feel free to try out and play with different functions.
If you are curious about learning data science to be in the front of fast-paced technological advancements, check out upGrad & IIIT-B’s Executive PG Programme in Data Science and upskill yourself for the future.
Frequently Asked Questions (FAQs)
1. What are the requisite skills required to develop a fake news detection project in Python?
2. How do companies use the Fake News Detection Projects of Python?
3. What are some other real-life applications of python?
Get Free Consultation
By submitting, I accept the T&C and
Privacy Policy
Start Your Career in Data Science Today
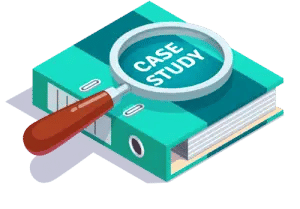
Top Resources