- Blog Categories
- Software Development
- Data Science
- AI/ML
- Marketing
- General
- MBA
- Management
- Legal
- Software Development Projects and Ideas
- 12 Computer Science Project Ideas
- 28 Beginner Software Projects
- Top 10 Engineering Project Ideas
- Top 10 Easy Final Year Projects
- Top 10 Mini Projects for Engineers
- 25 Best Django Project Ideas
- Top 20 MERN Stack Project Ideas
- Top 12 Real Time Projects
- Top 6 Major CSE Projects
- 12 Robotics Projects for All Levels
- Java Programming Concepts
- Abstract Class in Java and Methods
- Constructor Overloading in Java
- StringBuffer vs StringBuilder
- Java Identifiers: Syntax & Examples
- Types of Variables in Java Explained
- Composition in Java: Examples
- Append in Java: Implementation
- Loose Coupling vs Tight Coupling
- Integrity Constraints in DBMS
- Different Types of Operators Explained
- Career and Interview Preparation in IT
- Top 14 IT Courses for Jobs
- Top 20 Highest Paying Languages
- 23 Top CS Interview Q&A
- Best IT Jobs without Coding
- Software Engineer Salary in India
- 44 Agile Methodology Interview Q&A
- 10 Software Engineering Challenges
- Top 15 Tech's Daily Life Impact
- 10 Best Backends for React
- Cloud Computing Reference Models
- Web Development and Security
- Find Installed NPM Version
- Install Specific NPM Package Version
- Make API Calls in Angular
- Install Bootstrap in Angular
- Use Axios in React: Guide
- StrictMode in React: Usage
- 75 Cyber Security Research Topics
- Top 7 Languages for Ethical Hacking
- Top 20 Docker Commands
- Advantages of OOP
- Data Science Projects and Applications
- 42 Python Project Ideas for Beginners
- 13 Data Science Project Ideas
- 13 Data Structure Project Ideas
- 12 Real-World Python Applications
- Python Banking Project
- Data Science Course Eligibility
- Association Rule Mining Overview
- Cluster Analysis in Data Mining
- Classification in Data Mining
- KDD Process in Data Mining
- Data Structures and Algorithms
- Binary Tree Types Explained
- Binary Search Algorithm
- Sorting in Data Structure
- Binary Tree in Data Structure
- Binary Tree vs Binary Search Tree
- Recursion in Data Structure
- Data Structure Search Methods: Explained
- Binary Tree Interview Q&A
- Linear vs Binary Search
- Priority Queue Overview
- Python Programming and Tools
- Top 30 Python Pattern Programs
- List vs Tuple
- Python Free Online Course
- Method Overriding in Python
- Top 21 Python Developer Skills
- Reverse a Number in Python
- Switch Case Functions in Python
- Info Retrieval System Overview
- Reverse a Number in Python
- Real-World Python Applications
- Data Science Careers and Comparisons
- Data Analyst Salary in India
- Data Scientist Salary in India
- Free Excel Certification Course
- Actuary Salary in India
- Data Analyst Interview Guide
- Pandas Interview Guide
- Tableau Filters Explained
- Data Mining Techniques Overview
- Data Analytics Lifecycle Phases
- Data Science Vs Analytics Comparison
- Artificial Intelligence and Machine Learning Projects
- Exciting IoT Project Ideas
- 16 Exciting AI Project Ideas
- 45+ Interesting ML Project Ideas
- Exciting Deep Learning Projects
- 12 Intriguing Linear Regression Projects
- 13 Neural Network Projects
- 5 Exciting Image Processing Projects
- Top 8 Thrilling AWS Projects
- 12 Engaging AI Projects in Python
- NLP Projects for Beginners
- Concepts and Algorithms in AIML
- Basic CNN Architecture Explained
- 6 Types of Regression Models
- Data Preprocessing Steps
- Bagging vs Boosting in ML
- Multinomial Naive Bayes Overview
- Bayesian Network Example
- Bayes Theorem Guide
- Top 10 Dimensionality Reduction Techniques
- Neural Network Step-by-Step Guide
- Technical Guides and Comparisons
- Make a Chatbot in Python
- Compute Square Roots in Python
- Permutation vs Combination
- Image Segmentation Techniques
- Generative AI vs Traditional AI
- AI vs Human Intelligence
- Random Forest vs Decision Tree
- Neural Network Overview
- Perceptron Learning Algorithm
- Selection Sort Algorithm
- Career and Practical Applications in AIML
- AI Salary in India Overview
- Biological Neural Network Basics
- Top 10 AI Challenges
- Production System in AI
- Top 8 Raspberry Pi Alternatives
- Top 8 Open Source Projects
- 14 Raspberry Pi Project Ideas
- 15 MATLAB Project Ideas
- Top 10 Python NLP Libraries
- Naive Bayes Explained
- Digital Marketing Projects and Strategies
- 10 Best Digital Marketing Projects
- 17 Fun Social Media Projects
- Top 6 SEO Project Ideas
- Digital Marketing Case Studies
- Coca-Cola Marketing Strategy
- Nestle Marketing Strategy Analysis
- Zomato Marketing Strategy
- Monetize Instagram Guide
- Become a Successful Instagram Influencer
- 8 Best Lead Generation Techniques
- Digital Marketing Careers and Salaries
- Digital Marketing Salary in India
- Top 10 Highest Paying Marketing Jobs
- Highest Paying Digital Marketing Jobs
- SEO Salary in India
- Content Writer Salary Guide
- Digital Marketing Executive Roles
- Career in Digital Marketing Guide
- Future of Digital Marketing
- MBA in Digital Marketing Overview
- Digital Marketing Techniques and Channels
- 9 Types of Digital Marketing Channels
- Top 10 Benefits of Marketing Branding
- 100 Best YouTube Channel Ideas
- YouTube Earnings in India
- 7 Reasons to Study Digital Marketing
- Top 10 Digital Marketing Objectives
- 10 Best Digital Marketing Blogs
- Top 5 Industries Using Digital Marketing
- Growth of Digital Marketing in India
- Top Career Options in Marketing
- Interview Preparation and Skills
- 73 Google Analytics Interview Q&A
- 56 Social Media Marketing Q&A
- 78 Google AdWords Interview Q&A
- Top 133 SEO Interview Q&A
- 27+ Digital Marketing Q&A
- Digital Marketing Free Course
- Top 9 Skills for PPC Analysts
- Movies with Successful Social Media Campaigns
- Marketing Communication Steps
- Top 10 Reasons to Be an Affiliate Marketer
- Career Options and Paths
- Top 25 Highest Paying Jobs India
- Top 25 Highest Paying Jobs World
- Top 10 Highest Paid Commerce Job
- Career Options After 12th Arts
- Top 7 Commerce Courses Without Maths
- Top 7 Career Options After PCB
- Best Career Options for Commerce
- Career Options After 12th CS
- Top 10 Career Options After 10th
- 8 Best Career Options After BA
- Projects and Academic Pursuits
- 17 Exciting Final Year Projects
- Top 12 Commerce Project Topics
- Top 13 BCA Project Ideas
- Career Options After 12th Science
- Top 15 CS Jobs in India
- 12 Best Career Options After M.Com
- 9 Best Career Options After B.Sc
- 7 Best Career Options After BCA
- 22 Best Career Options After MCA
- 16 Top Career Options After CE
- Courses and Certifications
- 10 Best Job-Oriented Courses
- Best Online Computer Courses
- Top 15 Trending Online Courses
- Top 19 High Salary Certificate Courses
- 21 Best Programming Courses for Jobs
- What is SGPA? Convert to CGPA
- GPA to Percentage Calculator
- Highest Salary Engineering Stream
- 15 Top Career Options After Engineering
- 6 Top Career Options After BBA
- Job Market and Interview Preparation
- Why Should You Be Hired: 5 Answers
- Top 10 Future Career Options
- Top 15 Highest Paid IT Jobs India
- 5 Common Guesstimate Interview Q&A
- Average CEO Salary: Top Paid CEOs
- Career Options in Political Science
- Top 15 Highest Paying Non-IT Jobs
- Cover Letter Examples for Jobs
- Top 5 Highest Paying Freelance Jobs
- Top 10 Highest Paying Companies India
- Career Options and Paths After MBA
- 20 Best Careers After B.Com
- Career Options After MBA Marketing
- Top 14 Careers After MBA In HR
- Top 10 Highest Paying HR Jobs India
- How to Become an Investment Banker
- Career Options After MBA - High Paying
- Scope of MBA in Operations Management
- Best MBA for Working Professionals India
- MBA After BA - Is It Right For You?
- Best Online MBA Courses India
- MBA Project Ideas and Topics
- 11 Exciting MBA HR Project Ideas
- Top 15 MBA Project Ideas
- 18 Exciting MBA Marketing Projects
- MBA Project Ideas: Consumer Behavior
- What is Brand Management?
- What is Holistic Marketing?
- What is Green Marketing?
- Intro to Organizational Behavior Model
- Tech Skills Every MBA Should Learn
- Most Demanding Short Term Courses MBA
- MBA Salary, Resume, and Skills
- MBA Salary in India
- HR Salary in India
- Investment Banker Salary India
- MBA Resume Samples
- Sample SOP for MBA
- Sample SOP for Internship
- 7 Ways MBA Helps Your Career
- Must-have Skills in Sales Career
- 8 Skills MBA Helps You Improve
- Top 20+ SAP FICO Interview Q&A
- MBA Specializations and Comparative Guides
- Why MBA After B.Tech? 5 Reasons
- How to Answer 'Why MBA After Engineering?'
- Why MBA in Finance
- MBA After BSc: 10 Reasons
- Which MBA Specialization to choose?
- Top 10 MBA Specializations
- MBA vs Masters: Which to Choose?
- Benefits of MBA After CA
- 5 Steps to Management Consultant
- 37 Must-Read HR Interview Q&A
- Fundamentals and Theories of Management
- What is Management? Objectives & Functions
- Nature and Scope of Management
- Decision Making in Management
- Management Process: Definition & Functions
- Importance of Management
- What are Motivation Theories?
- Tools of Financial Statement Analysis
- Negotiation Skills: Definition & Benefits
- Career Development in HRM
- Top 20 Must-Have HRM Policies
- Project and Supply Chain Management
- Top 20 Project Management Case Studies
- 10 Innovative Supply Chain Projects
- Latest Management Project Topics
- 10 Project Management Project Ideas
- 6 Types of Supply Chain Models
- Top 10 Advantages of SCM
- Top 10 Supply Chain Books
- What is Project Description?
- Top 10 Project Management Companies
- Best Project Management Courses Online
- Salaries and Career Paths in Management
- Project Manager Salary in India
- Average Product Manager Salary India
- Supply Chain Management Salary India
- Salary After BBA in India
- PGDM Salary in India
- Top 7 Career Options in Management
- CSPO Certification Cost
- Why Choose Product Management?
- Product Management in Pharma
- Product Design in Operations Management
- Industry-Specific Management and Case Studies
- Amazon Business Case Study
- Service Delivery Manager Job
- Product Management Examples
- Product Management in Automobiles
- Product Management in Banking
- Sample SOP for Business Management
- Video Game Design Components
- Top 5 Business Courses India
- Free Management Online Course
- SCM Interview Q&A
- Fundamentals and Types of Law
- Acceptance in Contract Law
- Offer in Contract Law
- 9 Types of Evidence
- Types of Law in India
- Introduction to Contract Law
- Negotiable Instrument Act
- Corporate Tax Basics
- Intellectual Property Law
- Workmen Compensation Explained
- Lawyer vs Advocate Difference
- Law Education and Courses
- LLM Subjects & Syllabus
- Corporate Law Subjects
- LLM Course Duration
- Top 10 Online LLM Courses
- Online LLM Degree
- Step-by-Step Guide to Studying Law
- Top 5 Law Books to Read
- Why Legal Studies?
- Pursuing a Career in Law
- How to Become Lawyer in India
- Career Options and Salaries in Law
- Career Options in Law India
- Corporate Lawyer Salary India
- How To Become a Corporate Lawyer
- Career in Law: Starting, Salary
- Career Opportunities: Corporate Law
- Business Lawyer: Role & Salary Info
- Average Lawyer Salary India
- Top Career Options for Lawyers
- Types of Lawyers in India
- Steps to Become SC Lawyer in India
- Tutorials
- Software Tutorials
- C Tutorials
- Recursion in C: Fibonacci Series
- Checking String Palindromes in C
- Prime Number Program in C
- Implementing Square Root in C
- Matrix Multiplication in C
- Understanding Double Data Type
- Factorial of a Number in C
- Structure of a C Program
- Building a Calculator Program in C
- Compiling C Programs on Linux
- Java Tutorials
- Handling String Input in Java
- Determining Even and Odd Numbers
- Prime Number Checker
- Sorting a String
- User-Defined Exceptions
- Understanding the Thread Life Cycle
- Swapping Two Numbers
- Using Final Classes
- Area of a Triangle
- Skills
- Explore Skills
- Management Skills
- Software Engineering
- JavaScript
- Data Structure
- React.js
- Core Java
- Node.js
- Blockchain
- SQL
- Full stack development
- Devops
- NFT
- BigData
- Cyber Security
- Cloud Computing
- Database Design with MySQL
- Cryptocurrency
- Python
- Digital Marketings
- Advertising
- Influencer Marketing
- Performance Marketing
- Search Engine Marketing
- Email Marketing
- Content Marketing
- Social Media Marketing
- Display Advertising
- Marketing Analytics
- Web Analytics
- Affiliate Marketing
- MBA
- MBA in Finance
- MBA in HR
- MBA in Marketing
- MBA in Business Analytics
- MBA in Operations Management
- MBA in International Business
- MBA in Information Technology
- MBA in Healthcare Management
- MBA In General Management
- MBA in Agriculture
- MBA in Supply Chain Management
- MBA in Entrepreneurship
- MBA in Project Management
- Management Program
- Consumer Behaviour
- Supply Chain Management
- Financial Analytics
- Introduction to Fintech
- Introduction to HR Analytics
- Fundamentals of Communication
- Art of Effective Communication
- Introduction to Research Methodology
- Mastering Sales Technique
- Business Communication
- Fundamentals of Journalism
- Economics Masterclass
- Free Courses
- Home
- Blog
- Data Science
- Hadoop vs MongoDB: Which is More Secure for Big Data?
Hadoop vs MongoDB: Which is More Secure for Big Data?
Updated on Jun 30, 2023 | 9 min read
Share:
Table of Contents
By 2020, the global data generated will stand at 44 Zettabytes. As the amount of data continues to pile up, traditional data processing methods cannot suffice for processing vast volumes of data. This is where Big Data technologies and frameworks come in – these structures are designed to handle, process, analyze, interpret, and store vast volumes of data.
While there are numerous Big Data frameworks, today, we’re going to focus on two in particular – Hadoop and MongoDB.
What is Hadoop?
Hadoop was created by Doug Cutting. It is a Javed-based open-source platform for processing, modifying, and storing Big Data. Hadoop comprises of four core components, each designed to perform specific tasks associated with Big Data Analytics:
- Hadoop Distributed File System (HDFS) – It is a highly scalable, fault-tolerant file system that facilitates seamless data storage, access, and sharing across a huge network of connected servers.
- MapReduce – It is a software development framework used for processing large datasets in parallel by performing two crucial functions: mapping and reducing.
- YARN (Yet Another Resource Negotiator) – It is Hadoop’s architectural framework for scheduling and resource management.
- Hadoop Common – It is an assortment of libraries and functions that support the other three Hadoop components. YARN allows for simultaneous streaming, interactive, and batch processing.
What is MongoDB?
MongoDB is an open-source NoSQL database management framework. It is a document-oriented system that is highly scalable and flexible. One of the key features of MongoDB is that it can accommodate high volumes of distributed datasets and store data in collections (in key-value sets). MongoDB comprises of three core components:
- mongod: It is the primary daemon process for MongoDB.
- mongos: It is a controller and query router for sharded clusters.
- mongo: It is an interactive MongoDB shell.
Hadoop vs. MongoDB: A Comparison
- While Hadoop is a Java-based software application, MongoDB is a database written in C++. Hadoop is a suite/collection of products, but MongoDB is a standalone product in itself.
- Hadoop acts as a supplement to the RDBMS system for archiving data, whereas MongoDB can replace the existing RDBMS completely.
- Hadoop is best-suited for large-scale batch processing and long-duration ETL tasks, whereas MongoDB is excellent for real-time data mining and processing.
- MongoDB is highly useful in Geospatial Analysis since it comes with geospatial indexing which is absent in Hadoop.
- When it comes to the data format, Hadoop is pretty flexible. However, MongoDB can only import CSV and JSON data formats.
Integration with Other Technologies
Both Hadoop and MongoDB can be integrated with several different technologies to expand their functionality. For instance, Apache Spark, a quick and versatile cluster computing system, can be linked with Hadoop to use its in-memory processing capabilities for quicker data analysis. Apache Kafka, a distributed streaming technology, can be integrated with MongoDB to ingest and analyze real-time data streams.
Use Cases
Hadoop and MongoDB are used in various use cases based on their advantages. Hadoop is frequently used for massive batch processing and analytics in the banking, retail and telecommunications sectors. Due to its flexible document model and real-time capabilities, MongoDB is frequently utilized in use cases like content management systems, catalog management, customer data management, and IoT applications.
Performance and Scalability
The basic difference between MongoDB and Hadoop is that Hadoop’s distributed architecture enables horizontal scaling by adding affordable commodity hardware to the cluster. It can manage enormous amounts of data by spreading the effort across several nodes. By partitioning and distributing data across various servers, sharding allows MongoDB to scale horizontally. Due to this, MongoDB can effectively handle high workloads in writing and reading.
Data Consistency
Hadoop and MongoDB take distinct methods for data consistency. Updates to the data may not be instantly accessible to all cluster nodes since Hadoop’s distributed file system, HDFS, offers eventual consistency. On the other hand, MongoDB enables many data consistency levels, allowing customers to select the level that best meets their application needs.
Data Processing Paradigm
MongoDB’s query language and aggregation architecture enable real-time querying and ad-hoc data analysis, unlike Hadoop’s MapReduce framework, which is well-suited for batch processing and offline analytics. MongoDB’s document-oriented approach is perfect for use cases where data models change over time since it allows for flexible schema building and simple retrieval of layered data structures.
Machine Learning Integration
Hadoop and MongoDB can be connected with machine learning frameworks to perform sophisticated analytics and predictive modeling. Apache Mahout, an open-source machine learning toolkit, may be used with Hadoop without any issues for applications like clustering, classification, and recommendation. The use of machine learning techniques on MongoDB data is made possible by MongoDB’s integration with well-known machine learning platforms like Apache Spark MLlib and sci-kit-learn.
Cost Considerations
This is an important factor to consider while weighing the MongoDB and Hadoop differences. Cost elements such as hardware infrastructure, storage needs, and operational overhead should be considered when assessing the expenses related to Hadoop and MongoDB. Due to its distributed nature, Hadoop frequently necessitates a cluster of computers, which can result in increased hardware and maintenance costs. Due to MongoDB’s adaptable scaling, businesses can start small and grow as needed, potentially saving money on initial infrastructure expenditures.
Evolving Trends
Improvements in stream processing tools like Apache Flink and Apache Beam have been made in the Hadoop ecosystem. MongoDB has provided features including multi-document transactions, distributed transactions, and automated sharding upgrades to boost scalability and transactional capabilities further.
Explore Our Software Development Free Courses
Which is more secure and better for Big Data?
Both Hadoop and MongoDB are built for handling and managing Big Data, and both have their fair share of advantages and disadvantages. As we mentioned before, Hadoop is the best fit for batch processing, but it cannot handle real-time data, although you can run ad-hoc SQL queries with Hive.
On the contrary, MongoDB’s greatest strength is its flexibility and capability to replace the existing RDBMS. It is also excellent at handling real-time data analytics. So, if your company has real-time data with low latency or you need to create a new system by replacing the existing RDBMS, MongoDB is the way to go. However, if you need large-scale batch solutions, Hadoop is the tool for you.
upGrad’s Exclusive Software Development Webinar for you –
SAAS Business – What is So Different?
Although both Hadoop and MongoDB are highly scalable, flexible, fault-tolerant, and capable of handling large volumes of data. But when it comes to security, both have numerous drawbacks.
Hadoop’s shortcomings on the security front emerge from one central point – its complexity. Since Hadoop is an amalgamation of interrelated and co-operating components, it becomes difficult to configure and manage the platform. Also, if less experienced professionals are handling it, they may leave the attack vectors exposed to threats. More importantly, when Hadoop was designed, the concept of “security” was left out – initially, it was restricted only to private clusters in stable environments. And although now Hadoop has the necessary security features like authentication and authorization, they can be turned off as a default option.
Explore our Popular Software Engineering Courses
As of now, there are four documented vulnerabilities of Hadoop in the CVE (Common Vulnerabilities and Exposures) database, and its average CVSS (Common Vulnerability Scoring System) score is 6.3. Hence, it falls in the medium-risk segment.
Coming to MongoDB, its security shortcomings may not be as highly publicized or highlighted like Hadoop, but it has many crucial vulnerabilities nonetheless. Since both Hadoop and MongoDB have originated from private data centers and then integrated with cloud platforms, they have generated an ocean of attack vectors. Just like Hadoop, MongoDB has no access control. MongoDB records seven documented vulnerabilities in the CVE database with an average CVSS score of 6. Thus, it also falls in the medium-risk segment.
In-Demand Software Development Skills
So, as you can see, while both Hadoop and MongoDB can efficiently take care of the Big Data needs of your organization, they are not very reliable from the security perspective. Web applications built on these frameworks are usually shipped with the security features turned off by default. This only points to bad security practices, not just at the vendor’s end but also at the developer’s. The key to overcoming these drawbacks in security is to integrate Hadoop and MongoDB platforms with the proper control mechanisms that can promptly identify and remediate vulnerabilities within the software delivery pipeline, thereby facilitating security monitoring and evaluation for all endpoints in the system.
The debate of Hadoop vs MongoDB continues to grow but both are powerful management and processing solutions for big data, each with advantages and applications. Making an informed choice regarding which technology is more appropriate for your particular use case will involve understanding your organization’s specific requirements, including data processing requirements, real-time capabilities, scalability, and security considerations.
Popular Articles related to Software Development
If you are interested to know more about Big Data, check out our Advanced Certificate Programme in Big Data from IIIT Bangalore.
Learn Software Development Courses online from the World’s top Universities. Earn Executive PG Programs, Advanced Certificate Programs or Masters Programs to fast-track your career.
Frequently Asked Questions (FAQs)
1. What is the future scope of Hadoop?
2. What is the use of Hadoop in communication, media, and entertainment?
3. Where is MongoDB going at?
Get Free Consultation
By submitting, I accept the T&C and
Privacy Policy
Start Your Career in Data Science Today
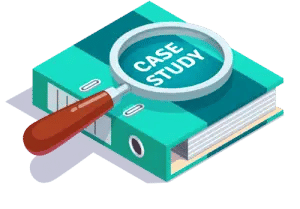