- Blog Categories
- Software Development
- Data Science
- AI/ML
- Marketing
- General
- MBA
- Management
- Legal
- Software Development Projects and Ideas
- 12 Computer Science Project Ideas
- 28 Beginner Software Projects
- Top 10 Engineering Project Ideas
- Top 10 Easy Final Year Projects
- Top 10 Mini Projects for Engineers
- 25 Best Django Project Ideas
- Top 20 MERN Stack Project Ideas
- Top 12 Real Time Projects
- Top 6 Major CSE Projects
- 12 Robotics Projects for All Levels
- Java Programming Concepts
- Abstract Class in Java and Methods
- Constructor Overloading in Java
- StringBuffer vs StringBuilder
- Java Identifiers: Syntax & Examples
- Types of Variables in Java Explained
- Composition in Java: Examples
- Append in Java: Implementation
- Loose Coupling vs Tight Coupling
- Integrity Constraints in DBMS
- Different Types of Operators Explained
- Career and Interview Preparation in IT
- Top 14 IT Courses for Jobs
- Top 20 Highest Paying Languages
- 23 Top CS Interview Q&A
- Best IT Jobs without Coding
- Software Engineer Salary in India
- 44 Agile Methodology Interview Q&A
- 10 Software Engineering Challenges
- Top 15 Tech's Daily Life Impact
- 10 Best Backends for React
- Cloud Computing Reference Models
- Web Development and Security
- Find Installed NPM Version
- Install Specific NPM Package Version
- Make API Calls in Angular
- Install Bootstrap in Angular
- Use Axios in React: Guide
- StrictMode in React: Usage
- 75 Cyber Security Research Topics
- Top 7 Languages for Ethical Hacking
- Top 20 Docker Commands
- Advantages of OOP
- Data Science Projects and Applications
- 42 Python Project Ideas for Beginners
- 13 Data Science Project Ideas
- 13 Data Structure Project Ideas
- 12 Real-World Python Applications
- Python Banking Project
- Data Science Course Eligibility
- Association Rule Mining Overview
- Cluster Analysis in Data Mining
- Classification in Data Mining
- KDD Process in Data Mining
- Data Structures and Algorithms
- Binary Tree Types Explained
- Binary Search Algorithm
- Sorting in Data Structure
- Binary Tree in Data Structure
- Binary Tree vs Binary Search Tree
- Recursion in Data Structure
- Data Structure Search Methods: Explained
- Binary Tree Interview Q&A
- Linear vs Binary Search
- Priority Queue Overview
- Python Programming and Tools
- Top 30 Python Pattern Programs
- List vs Tuple
- Python Free Online Course
- Method Overriding in Python
- Top 21 Python Developer Skills
- Reverse a Number in Python
- Switch Case Functions in Python
- Info Retrieval System Overview
- Reverse a Number in Python
- Real-World Python Applications
- Data Science Careers and Comparisons
- Data Analyst Salary in India
- Data Scientist Salary in India
- Free Excel Certification Course
- Actuary Salary in India
- Data Analyst Interview Guide
- Pandas Interview Guide
- Tableau Filters Explained
- Data Mining Techniques Overview
- Data Analytics Lifecycle Phases
- Data Science Vs Analytics Comparison
- Artificial Intelligence and Machine Learning Projects
- Exciting IoT Project Ideas
- 16 Exciting AI Project Ideas
- 45+ Interesting ML Project Ideas
- Exciting Deep Learning Projects
- 12 Intriguing Linear Regression Projects
- 13 Neural Network Projects
- 5 Exciting Image Processing Projects
- Top 8 Thrilling AWS Projects
- 12 Engaging AI Projects in Python
- NLP Projects for Beginners
- Concepts and Algorithms in AIML
- Basic CNN Architecture Explained
- 6 Types of Regression Models
- Data Preprocessing Steps
- Bagging vs Boosting in ML
- Multinomial Naive Bayes Overview
- Bayesian Network Example
- Bayes Theorem Guide
- Top 10 Dimensionality Reduction Techniques
- Neural Network Step-by-Step Guide
- Technical Guides and Comparisons
- Make a Chatbot in Python
- Compute Square Roots in Python
- Permutation vs Combination
- Image Segmentation Techniques
- Generative AI vs Traditional AI
- AI vs Human Intelligence
- Random Forest vs Decision Tree
- Neural Network Overview
- Perceptron Learning Algorithm
- Selection Sort Algorithm
- Career and Practical Applications in AIML
- AI Salary in India Overview
- Biological Neural Network Basics
- Top 10 AI Challenges
- Production System in AI
- Top 8 Raspberry Pi Alternatives
- Top 8 Open Source Projects
- 14 Raspberry Pi Project Ideas
- 15 MATLAB Project Ideas
- Top 10 Python NLP Libraries
- Naive Bayes Explained
- Digital Marketing Projects and Strategies
- 10 Best Digital Marketing Projects
- 17 Fun Social Media Projects
- Top 6 SEO Project Ideas
- Digital Marketing Case Studies
- Coca-Cola Marketing Strategy
- Nestle Marketing Strategy Analysis
- Zomato Marketing Strategy
- Monetize Instagram Guide
- Become a Successful Instagram Influencer
- 8 Best Lead Generation Techniques
- Digital Marketing Careers and Salaries
- Digital Marketing Salary in India
- Top 10 Highest Paying Marketing Jobs
- Highest Paying Digital Marketing Jobs
- SEO Salary in India
- Content Writer Salary Guide
- Digital Marketing Executive Roles
- Career in Digital Marketing Guide
- Future of Digital Marketing
- MBA in Digital Marketing Overview
- Digital Marketing Techniques and Channels
- 9 Types of Digital Marketing Channels
- Top 10 Benefits of Marketing Branding
- 100 Best YouTube Channel Ideas
- YouTube Earnings in India
- 7 Reasons to Study Digital Marketing
- Top 10 Digital Marketing Objectives
- 10 Best Digital Marketing Blogs
- Top 5 Industries Using Digital Marketing
- Growth of Digital Marketing in India
- Top Career Options in Marketing
- Interview Preparation and Skills
- 73 Google Analytics Interview Q&A
- 56 Social Media Marketing Q&A
- 78 Google AdWords Interview Q&A
- Top 133 SEO Interview Q&A
- 27+ Digital Marketing Q&A
- Digital Marketing Free Course
- Top 9 Skills for PPC Analysts
- Movies with Successful Social Media Campaigns
- Marketing Communication Steps
- Top 10 Reasons to Be an Affiliate Marketer
- Career Options and Paths
- Top 25 Highest Paying Jobs India
- Top 25 Highest Paying Jobs World
- Top 10 Highest Paid Commerce Job
- Career Options After 12th Arts
- Top 7 Commerce Courses Without Maths
- Top 7 Career Options After PCB
- Best Career Options for Commerce
- Career Options After 12th CS
- Top 10 Career Options After 10th
- 8 Best Career Options After BA
- Projects and Academic Pursuits
- 17 Exciting Final Year Projects
- Top 12 Commerce Project Topics
- Top 13 BCA Project Ideas
- Career Options After 12th Science
- Top 15 CS Jobs in India
- 12 Best Career Options After M.Com
- 9 Best Career Options After B.Sc
- 7 Best Career Options After BCA
- 22 Best Career Options After MCA
- 16 Top Career Options After CE
- Courses and Certifications
- 10 Best Job-Oriented Courses
- Best Online Computer Courses
- Top 15 Trending Online Courses
- Top 19 High Salary Certificate Courses
- 21 Best Programming Courses for Jobs
- What is SGPA? Convert to CGPA
- GPA to Percentage Calculator
- Highest Salary Engineering Stream
- 15 Top Career Options After Engineering
- 6 Top Career Options After BBA
- Job Market and Interview Preparation
- Why Should You Be Hired: 5 Answers
- Top 10 Future Career Options
- Top 15 Highest Paid IT Jobs India
- 5 Common Guesstimate Interview Q&A
- Average CEO Salary: Top Paid CEOs
- Career Options in Political Science
- Top 15 Highest Paying Non-IT Jobs
- Cover Letter Examples for Jobs
- Top 5 Highest Paying Freelance Jobs
- Top 10 Highest Paying Companies India
- Career Options and Paths After MBA
- 20 Best Careers After B.Com
- Career Options After MBA Marketing
- Top 14 Careers After MBA In HR
- Top 10 Highest Paying HR Jobs India
- How to Become an Investment Banker
- Career Options After MBA - High Paying
- Scope of MBA in Operations Management
- Best MBA for Working Professionals India
- MBA After BA - Is It Right For You?
- Best Online MBA Courses India
- MBA Project Ideas and Topics
- 11 Exciting MBA HR Project Ideas
- Top 15 MBA Project Ideas
- 18 Exciting MBA Marketing Projects
- MBA Project Ideas: Consumer Behavior
- What is Brand Management?
- What is Holistic Marketing?
- What is Green Marketing?
- Intro to Organizational Behavior Model
- Tech Skills Every MBA Should Learn
- Most Demanding Short Term Courses MBA
- MBA Salary, Resume, and Skills
- MBA Salary in India
- HR Salary in India
- Investment Banker Salary India
- MBA Resume Samples
- Sample SOP for MBA
- Sample SOP for Internship
- 7 Ways MBA Helps Your Career
- Must-have Skills in Sales Career
- 8 Skills MBA Helps You Improve
- Top 20+ SAP FICO Interview Q&A
- MBA Specializations and Comparative Guides
- Why MBA After B.Tech? 5 Reasons
- How to Answer 'Why MBA After Engineering?'
- Why MBA in Finance
- MBA After BSc: 10 Reasons
- Which MBA Specialization to choose?
- Top 10 MBA Specializations
- MBA vs Masters: Which to Choose?
- Benefits of MBA After CA
- 5 Steps to Management Consultant
- 37 Must-Read HR Interview Q&A
- Fundamentals and Theories of Management
- What is Management? Objectives & Functions
- Nature and Scope of Management
- Decision Making in Management
- Management Process: Definition & Functions
- Importance of Management
- What are Motivation Theories?
- Tools of Financial Statement Analysis
- Negotiation Skills: Definition & Benefits
- Career Development in HRM
- Top 20 Must-Have HRM Policies
- Project and Supply Chain Management
- Top 20 Project Management Case Studies
- 10 Innovative Supply Chain Projects
- Latest Management Project Topics
- 10 Project Management Project Ideas
- 6 Types of Supply Chain Models
- Top 10 Advantages of SCM
- Top 10 Supply Chain Books
- What is Project Description?
- Top 10 Project Management Companies
- Best Project Management Courses Online
- Salaries and Career Paths in Management
- Project Manager Salary in India
- Average Product Manager Salary India
- Supply Chain Management Salary India
- Salary After BBA in India
- PGDM Salary in India
- Top 7 Career Options in Management
- CSPO Certification Cost
- Why Choose Product Management?
- Product Management in Pharma
- Product Design in Operations Management
- Industry-Specific Management and Case Studies
- Amazon Business Case Study
- Service Delivery Manager Job
- Product Management Examples
- Product Management in Automobiles
- Product Management in Banking
- Sample SOP for Business Management
- Video Game Design Components
- Top 5 Business Courses India
- Free Management Online Course
- SCM Interview Q&A
- Fundamentals and Types of Law
- Acceptance in Contract Law
- Offer in Contract Law
- 9 Types of Evidence
- Types of Law in India
- Introduction to Contract Law
- Negotiable Instrument Act
- Corporate Tax Basics
- Intellectual Property Law
- Workmen Compensation Explained
- Lawyer vs Advocate Difference
- Law Education and Courses
- LLM Subjects & Syllabus
- Corporate Law Subjects
- LLM Course Duration
- Top 10 Online LLM Courses
- Online LLM Degree
- Step-by-Step Guide to Studying Law
- Top 5 Law Books to Read
- Why Legal Studies?
- Pursuing a Career in Law
- How to Become Lawyer in India
- Career Options and Salaries in Law
- Career Options in Law India
- Corporate Lawyer Salary India
- How To Become a Corporate Lawyer
- Career in Law: Starting, Salary
- Career Opportunities: Corporate Law
- Business Lawyer: Role & Salary Info
- Average Lawyer Salary India
- Top Career Options for Lawyers
- Types of Lawyers in India
- Steps to Become SC Lawyer in India
- Tutorials
- Software Tutorials
- C Tutorials
- Recursion in C: Fibonacci Series
- Checking String Palindromes in C
- Prime Number Program in C
- Implementing Square Root in C
- Matrix Multiplication in C
- Understanding Double Data Type
- Factorial of a Number in C
- Structure of a C Program
- Building a Calculator Program in C
- Compiling C Programs on Linux
- Java Tutorials
- Handling String Input in Java
- Determining Even and Odd Numbers
- Prime Number Checker
- Sorting a String
- User-Defined Exceptions
- Understanding the Thread Life Cycle
- Swapping Two Numbers
- Using Final Classes
- Area of a Triangle
- Skills
- Explore Skills
- Management Skills
- Software Engineering
- JavaScript
- Data Structure
- React.js
- Core Java
- Node.js
- Blockchain
- SQL
- Full stack development
- Devops
- NFT
- BigData
- Cyber Security
- Cloud Computing
- Database Design with MySQL
- Cryptocurrency
- Python
- Digital Marketings
- Advertising
- Influencer Marketing
- Performance Marketing
- Search Engine Marketing
- Email Marketing
- Content Marketing
- Social Media Marketing
- Display Advertising
- Marketing Analytics
- Web Analytics
- Affiliate Marketing
- MBA
- MBA in Finance
- MBA in HR
- MBA in Marketing
- MBA in Business Analytics
- MBA in Operations Management
- MBA in International Business
- MBA in Information Technology
- MBA in Healthcare Management
- MBA In General Management
- MBA in Agriculture
- MBA in Supply Chain Management
- MBA in Entrepreneurship
- MBA in Project Management
- Management Program
- Consumer Behaviour
- Supply Chain Management
- Financial Analytics
- Introduction to Fintech
- Introduction to HR Analytics
- Fundamentals of Communication
- Art of Effective Communication
- Introduction to Research Methodology
- Mastering Sales Technique
- Business Communication
- Fundamentals of Journalism
- Economics Masterclass
- Free Courses
- Home
- Blog
- Data Science
- R vs Python Data Science: The Difference
R vs Python Data Science: The Difference
Updated on Oct 28, 2024 | 11 min read
Share:
Table of Contents
In terms of data science programming languages, R and Python are at the top of the list. Of course , understanding two of them is the best option. R and Python are time-consuming to learn, and not everyone has that luxury. Python is a popular programming language with an easy-to-understand syntax. R, on the other hand, was created by statisticians and includes their special language. If one wants to inculcate Data science tools in his/her skillset, it can be followed at upGrad data science for beginners.
Both R and Python are widely used open-source programming languages. New libraries or tools are introduced to their respective catalogues regularly. R is mostly used for statistical analysis, whereas Python is more suitable for building end-to-end data science pipelines. For more information on data science course fees click here.
These two open-source languages seem remarkably similar in many aspects. Both languages are free to download and use for data science operations related to data processing and mechanization to data analysis and research. The most significant distinction would be that Python is a general-purpose programming language, whereas R is a statistical analysis tool. In this blog, we'll go over some of the r vs python data science content, as well as how they're used in data science and statistics. More on Data Science Bootcamp Training can be followed at data science bootcamps.
What is Python?
Python is a general-purpose, object-oriented programming language that uses white space extensively to improve code readability. Python, which was first released in 1989, is a popular programming language among programmers and developers. Python is one of the most widely used programming languages, trailing behind Java and C.
You can learn about python for data science and enhance your skillset for pursuing a career. Python can perform many of the same activities as R, including data manipulation, engineering, feature selection, web scraping, and app development. Python is a programming language that can be used to deploy and execute machine learning on a big scale. Python code is more versatile and robust than R code. Python does not have many gathering and analysis, and machine learning modules a few years ago. Python has recently caught up and now offers cutting-edge APIs for machine learning and artificial intelligence. Numpy, Pandas, Scipy, Scikit-learn, and Seaborn are five Python libraries that can be used to perform most data science tasks. Get to know more about top R libraries for data science.
Python is intended to be a very understandable language. It typically uses English terms instead of punctuation and has fewer syntactical structures than other languages. Python is a must-have skill for students around the world who want to become exceptional software engineers, particularly if they work in the Web Development field. I'll go over some of the primary benefits of learning Python:
- Python is interpreted: Python is handled by the interpreter at runtime. Before running your software, users need not require compiling. This is comparable to the programming languages PERL and PHP.
- Python is Interactive: You can sit at a Python interface and write your programs by interacting directly with the interpreter.
- Python is Object-Oriented: Python supports the Object-Oriented programming style or approach, which encapsulates code inside objects.
- Python is a Complete beginner Language: Python is an excellent language for beginning programmers, as it allows for the creation of a variety of programs, ranging from simple text analysis to web browsers and games.
Python supports both utilitarian and structured programming methodologies, as well as object-oriented programming (OOP). It can be used as a scripting language or compiled into byte-code for large-scale application development. It allows dynamic type verification and supports extremely high-level dynamic data types.
What is R?
R is a free and open-source programming language for quantitative analysis and data visualization. R, which was first released in 1992, has a diverse ecosystem that includes complex information models and beautiful data reporting capabilities. For simpler statistical analysis, visualization, and reporting, R is often used within RStudio which is an Integrated Development Environment (IDE). Shiny allows R programmes to be utilized immediately and actively on the web.
In 1995, Ross Ihaka and Robert Gentleman released R, an open-source adaptation of the S programming language. The goal was to create a language that aimed at making data analysis, statistics, and graphical models easier and more user-friendly. R was first used mostly in academia and research, but it has recently gained popularity in the business world. As a result, R has become one of the most used statistical languages in the business world.
R's vast community, which offers assistance through mailing groups, user-contributed documentation, and a very prominent Stack Overflow group, is one of its key strengths. CRAN, a massive repository of curated R packages that anyone can freely contribute to, is another option. These packages contain a set of R functions and data that make it simple to get started with the most up-to-date techniques right now. CRAN (open-source repository) contains approximately 12000 packages. R is the preferred option for statistical analysis, particularly for specialist analytical tasks, due to its extensive library.
R offers a wide range of libraries and tools for the following steps:
- Data cleansing and preparation
- Making visual representations
- Machine learning & Deep Learning algorithms
R vs Python: Table of Comparison
Parameters | R | Python |
Popularity | Widely used in academia and statistics | Commonly used in industry and academia |
Learning Curve | The steeper learning curve for beginners | Relatively easier for beginners |
Syntax | Syntax emphasises statistical analysis | Syntax emphasises general programming |
Data Manipulation | Excellent for data manipulation and analysis | Robust data manipulation libraries |
Visualisation | Powerful visualisation libraries (ggplot2) | A rich ecosystem of visualisation libraries |
Machine Learning | Extensive range of machine learning packages | Comprehensive machine learning libraries |
Community Support | Active and supportive community | An oversized and vibrant community of users |
Integration | Limited integration with non-R tools | Seamless integration with other tools |
Performance | Slower execution for large datasets | Faster execution for large datasets |
R vs Python: Detailed Comparison
The approach to data science is where the two languages differ the most. Large communities support both open-source programming languages, which are constantly expanding their libraries and tools. However, although R is primarily used for quantitative statistical analysis, Python offers a broader approach to data manipulation. However, although R is primarily being used for statistical analysis, Python offers a broader approach to data manipulation.
Python, like C++ and Java, is a multi-purpose language with a legible syntax that is simple to pick up. Python is used by programmers in scalable production environments to conduct data analysis and machine learning. R, on the other hand, is a statistical programming language that relies largely on statistical models and specialized analytics. R is a statistical programming language that allows data scientists to perform in-depth statistical research with only a few code lines and stunning data visualizations.
R vs Python: Use of R and Python
When and How to Use R?
R is primarily utilized when data analysis tasks necessitate isolated computing or processing on separate servers. Because of the large number of packages and readily accessible tests that often offer you the appropriate tools to get up and running, it's fantastic for exploratory work and useful for practically any form of data analysis.
R is even capable of being used as part of a big data solution. Installing the RStudio IDE, which makes R user-friendly for those without programming experience, is a recommended initial step for getting started with R. If one wants to learn about various important R packages, it can follow at top libraries of R
Some of the Important Packages to be installed are as follows :
- dplyr, plyr and data.table for manipulation of the packages
- caret for performing various ML (Machine Learning) operations
- stringr for performing String Manipulation
- ggvis, lattice, and ggplot2 for data visualization purposes
- zoo for performing operations with regular/irregular time series data
When and How to Use Python?
When data analysis operations need to be connected with web apps or statistical code needs to be embedded into a production database, Python is a good choice. It's a wonderful tool for implementing algorithms for operational use because it's a full-fledged programming language.
To use Python for data analysis, you'll need to install
- NumPy/SciPy (scientific /computational operations)
- pandas (data manipulation)
- matplotlib for plotting and data visualization
- scikit-learn for machine learning
Python is smooth and easy to learn owing to its simple syntax. It's thought to be a useful language for new programmers.
R vs Python: Popularity
R:
R has been widely used in academia and statistics for many years. It has a strong presence in the academic and research community due to its extensive statistical analysis capabilities.
Python:
Python has recently gained popularity and is widely used in industry and academia. Due to its versatility and extensive libraries, it has become the go-to language for various applications, including data science.
R vs Python: Learning Curve
R:
R has a steeper learning curve, especially for beginners with no programming background. Its syntax and focus on statistical analysis can be challenging for newcomers.
Python:
Python has a relatively easier learning curve than R. Its syntax is straightforward and readable, making it more accessible for beginners. Python's focus on general-purpose programming also contributes to its ease of learning.
R vs Python: Syntax
R:
R syntax is explicitly designed for statistical analysis and data manipulation. It provides a wide range of statistical functions and operators that make it convenient for complex data operations.
Python:
Python syntax is more generalized and emphasizes general-purpose programming. While it also supports statistical analysis, its syntax is more versatile and can be applied to various domains beyond data science.
R vs Python: Data Manipulation
R:
R excels in data manipulation and analysis. It offers a variety of built-in functions and packages, such as dplyr and tidyr, which provide efficient and intuitive ways to handle and clean data.
Python:
Python offers robust data manipulation libraries such as Pandas, which provide potent data structures and functions for data wrangling. Python's libraries are known for their efficiency and versatility in handling large datasets.
R vs Python: Visualization
R:
R has a powerful visualization library called ggplot2, which allows users to create visually appealing and customizable plots. It offers a wide range of statistical and exploratory visualization techniques.
Python:
Python has a rich ecosystem of visualization libraries, including Matplotlib, Seaborn, and Plotly. These libraries provide extensive options for creating static and interactive visualizations, catering to different data science needs.
R vs Python: Machine Learning
R:
R offers extensive machine learning packages, such as Caret and Random Forest, making it a popular choice for statistical modeling and predictive analytics. It has a long history of statistical modeling and includes specialized functions for various algorithms.
Python:
Python provides comprehensive machine-learning libraries covering many machine-learning algorithms, such as sci-kit-learn and TensorFlow. Python's machine-learning ecosystem is vast and continuously expanding, making it suitable for various applications.
R vs Python: Community Support
R:
R has an active and supportive community, especially in academia and statistics. There are numerous online forums, mailing lists, and dedicated websites where R users can seek help, share knowledge, and collaborate.
Python:
Python has a large and vibrant community of users and developers. It has an extensive online presence, including dedicated forums, communities, and documentation, making it easy to find support, resources, and solutions to programming or data science-related queries.
R vs Python: Integration
R:
R's integration with non-R tools can be limited. While it can be connected with other programming languages, the level of integration is not as seamless as Python. R is often a standalone tool for statistical analysis and data manipulation.
Python:
Python offers seamless integration with other tools and languages. It can be easily integrated with databases, web frameworks, big data technologies, and other programming languages, allowing for flexible and scalable data science workflows.
R vs Python: Performance
R:
R can be slower in execution, especially when dealing with large datasets. It may require optimization techniques or a switch to alternative packages for better performance.
Python:
Python, when used with efficient libraries such as NumPy and Pandas, can deliver
R vs Python: Pros and Cons
R: Pros and Cons
Pros
- R has a vibrant community and a thriving ecosystem of cutting-edge packages. CRAN, Bioconductor, and GitHub all have packages.
- R is a statistical programming language created by statisticians for statisticians. They can use R code and packages to explain ideas and concepts. We don’t need a Computer Science/ Technical background to get started.
- R is more suited for the visualization of data because of its powerful packages like ggplot2, googleVis , rCharts and ggvis
Cons
- R is difficult at the very beginning to learn in contrast to python
- R's programs and functions are divided into several packages. As a result, it is slower than programs like MATLAB and Python.
- Due to the large number of libraries in CRAN, some of the packages are of poor quality.
Python: Pros and Cons
Pros
- Python is a simple and intuitive general-purpose programming language. This results in a relatively flat learning curve, as well as an increase in the speed with which you can build programs.
- Python is very easy to understand, and due to its simplicity, it is productive in nature. Developers do not need to spend a lot of time learning the programming language's syntax or behavior.
- During execution, Python allocates the data type automatically. Naming variables and their data types are not a concern for the programmer/developer.
Cons
- Python code is being executed line by line. However, because Python is an interpreted language, it frequently results in delayed execution.
- Data visualization in Python is less appealing or attractive in contrast to R.
- It does not provide a replacement for the hundreds of necessary R packages.
How are they Similar?
R and Python are similar in several ways when it comes to data science:
- Data Manipulation: R and Python provide powerful libraries (such as Pandas in Python and dplyr in R) that offer extensive functionality for data manipulation, including filtering, merging, reshaping, and aggregating datasets.
- Statistical Analysis: Both languages have robust statistical analysis capabilities. They offer various statistical functions, hypothesis testing, regression analysis, and other statistical techniques for data exploration and modeling.
- Visualization: While R has the popular ggplot2 library for visualization, Python also offers a variety of visualization libraries, such as Matplotlib, Seaborn, and Plotly. Both languages allow users to create informative and visually appealing plots and charts.
- Machine Learning: Both R and Python have extensive machine learning libraries. R has packages like the caret, Random Forest, and glmnet, while Python provides scikit-learn, TensorFlow, PyTorch, and Keras. Both languages cover various machine learning algorithms, enabling users to build predictive models.
What Should You Choose Between R and Python Data Science?
Choosing between R and Python for data science depends on several factors, such as personal preference, project requirements, and existing expertise. Here are some considerations to help make a decision:
- Background and Learning Curve: If you have a background in statistics or come from an academic/research field, R's syntax and focus on statistical analysis might be more familiar and suitable. On the other hand, if you have a programming background or prefer a universal language, Python's syntax and ease of learning could be advantageous.
- Industry Usage: Consider the industry you're working in or aiming to enter. Python is widely adopted in various industries, including tech, finance, and retail, making it a practical choice for career prospects and industry-specific applications.
- Project Requirements: Evaluate the specific requirements of your project. R's extensive statistical packages and libraries may be more suitable if your work heavily involves statistical analysis, econometrics, or advanced modeling.
- Team Collaboration: Consider the expertise of your team members or colleagues. If you're working with a team with experience in one language, leveraging their existing knowledge and resources can lead to better collaboration and efficiency.
Conclusion
We can conclude in this blog that opting for r or Python normally depends upon the following.
- The main objective of the operation: Python generally suits those operations in which deployment is being focused at. R, on the other hand, is usually suited when data analysis is being focused on.
- The amount of time for learning from the very beginning also decides which language to go for. Python is most suited when time is less as being easy to learn, whereas R, being more difficult than Python, is suited when an ample amount of time is available.
- Respective Organization/Industry in which we are working using which tool (R or Python).
Enhance your skills with our top Data Science Certifications. Explore the programs below to find your ideal match.
Explore our Popular Data Science Certifications
Elevate your expertise with our Top Data Science Skills to Learn for upskilling. Browse the programs below to find the perfect fit for your goals.
Top Data Science Skills to Learn to upskill
SL. No | Top Data Science Skills to Learn | |
1 |
Data Analysis Online Courses | Inferential Statistics Online Courses |
2 |
Hypothesis Testing Online Courses | Logistic Regression Online Courses |
3 |
Linear Regression Courses | Linear Algebra for Analysis Online Courses |
Boost your skills with our top-rated Data Science articles. Browse through the curated resources below to discover the perfect fit for you.
Read our popular Data Science Articles
Frequently Asked Questions (FAQs)
1. Which is better, R or Python for data science?
2. Is R better than Python?
3. Is R better than Python for machine learning?
4. Do data scientists use both R and Python?
Get Free Consultation
By submitting, I accept the T&C and
Privacy Policy
Start Your Career in Data Science Today
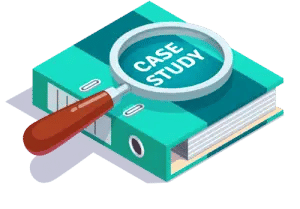
Top Resources