- Blog Categories
- Software Development
- Data Science
- AI/ML
- Marketing
- General
- MBA
- Management
- Legal
- Software Development Projects and Ideas
- 12 Computer Science Project Ideas
- 28 Beginner Software Projects
- Top 10 Engineering Project Ideas
- Top 10 Easy Final Year Projects
- Top 10 Mini Projects for Engineers
- 25 Best Django Project Ideas
- Top 20 MERN Stack Project Ideas
- Top 12 Real Time Projects
- Top 6 Major CSE Projects
- 12 Robotics Projects for All Levels
- Java Programming Concepts
- Abstract Class in Java and Methods
- Constructor Overloading in Java
- StringBuffer vs StringBuilder
- Java Identifiers: Syntax & Examples
- Types of Variables in Java Explained
- Composition in Java: Examples
- Append in Java: Implementation
- Loose Coupling vs Tight Coupling
- Integrity Constraints in DBMS
- Different Types of Operators Explained
- Career and Interview Preparation in IT
- Top 14 IT Courses for Jobs
- Top 20 Highest Paying Languages
- 23 Top CS Interview Q&A
- Best IT Jobs without Coding
- Software Engineer Salary in India
- 44 Agile Methodology Interview Q&A
- 10 Software Engineering Challenges
- Top 15 Tech's Daily Life Impact
- 10 Best Backends for React
- Cloud Computing Reference Models
- Web Development and Security
- Find Installed NPM Version
- Install Specific NPM Package Version
- Make API Calls in Angular
- Install Bootstrap in Angular
- Use Axios in React: Guide
- StrictMode in React: Usage
- 75 Cyber Security Research Topics
- Top 7 Languages for Ethical Hacking
- Top 20 Docker Commands
- Advantages of OOP
- Data Science Projects and Applications
- 42 Python Project Ideas for Beginners
- 13 Data Science Project Ideas
- 13 Data Structure Project Ideas
- 12 Real-World Python Applications
- Python Banking Project
- Data Science Course Eligibility
- Association Rule Mining Overview
- Cluster Analysis in Data Mining
- Classification in Data Mining
- KDD Process in Data Mining
- Data Structures and Algorithms
- Binary Tree Types Explained
- Binary Search Algorithm
- Sorting in Data Structure
- Binary Tree in Data Structure
- Binary Tree vs Binary Search Tree
- Recursion in Data Structure
- Data Structure Search Methods: Explained
- Binary Tree Interview Q&A
- Linear vs Binary Search
- Priority Queue Overview
- Python Programming and Tools
- Top 30 Python Pattern Programs
- List vs Tuple
- Python Free Online Course
- Method Overriding in Python
- Top 21 Python Developer Skills
- Reverse a Number in Python
- Switch Case Functions in Python
- Info Retrieval System Overview
- Reverse a Number in Python
- Real-World Python Applications
- Data Science Careers and Comparisons
- Data Analyst Salary in India
- Data Scientist Salary in India
- Free Excel Certification Course
- Actuary Salary in India
- Data Analyst Interview Guide
- Pandas Interview Guide
- Tableau Filters Explained
- Data Mining Techniques Overview
- Data Analytics Lifecycle Phases
- Data Science Vs Analytics Comparison
- Artificial Intelligence and Machine Learning Projects
- Exciting IoT Project Ideas
- 16 Exciting AI Project Ideas
- 45+ Interesting ML Project Ideas
- Exciting Deep Learning Projects
- 12 Intriguing Linear Regression Projects
- 13 Neural Network Projects
- 5 Exciting Image Processing Projects
- Top 8 Thrilling AWS Projects
- 12 Engaging AI Projects in Python
- NLP Projects for Beginners
- Concepts and Algorithms in AIML
- Basic CNN Architecture Explained
- 6 Types of Regression Models
- Data Preprocessing Steps
- Bagging vs Boosting in ML
- Multinomial Naive Bayes Overview
- Bayesian Network Example
- Bayes Theorem Guide
- Top 10 Dimensionality Reduction Techniques
- Neural Network Step-by-Step Guide
- Technical Guides and Comparisons
- Make a Chatbot in Python
- Compute Square Roots in Python
- Permutation vs Combination
- Image Segmentation Techniques
- Generative AI vs Traditional AI
- AI vs Human Intelligence
- Random Forest vs Decision Tree
- Neural Network Overview
- Perceptron Learning Algorithm
- Selection Sort Algorithm
- Career and Practical Applications in AIML
- AI Salary in India Overview
- Biological Neural Network Basics
- Top 10 AI Challenges
- Production System in AI
- Top 8 Raspberry Pi Alternatives
- Top 8 Open Source Projects
- 14 Raspberry Pi Project Ideas
- 15 MATLAB Project Ideas
- Top 10 Python NLP Libraries
- Naive Bayes Explained
- Digital Marketing Projects and Strategies
- 10 Best Digital Marketing Projects
- 17 Fun Social Media Projects
- Top 6 SEO Project Ideas
- Digital Marketing Case Studies
- Coca-Cola Marketing Strategy
- Nestle Marketing Strategy Analysis
- Zomato Marketing Strategy
- Monetize Instagram Guide
- Become a Successful Instagram Influencer
- 8 Best Lead Generation Techniques
- Digital Marketing Careers and Salaries
- Digital Marketing Salary in India
- Top 10 Highest Paying Marketing Jobs
- Highest Paying Digital Marketing Jobs
- SEO Salary in India
- Content Writer Salary Guide
- Digital Marketing Executive Roles
- Career in Digital Marketing Guide
- Future of Digital Marketing
- MBA in Digital Marketing Overview
- Digital Marketing Techniques and Channels
- 9 Types of Digital Marketing Channels
- Top 10 Benefits of Marketing Branding
- 100 Best YouTube Channel Ideas
- YouTube Earnings in India
- 7 Reasons to Study Digital Marketing
- Top 10 Digital Marketing Objectives
- 10 Best Digital Marketing Blogs
- Top 5 Industries Using Digital Marketing
- Growth of Digital Marketing in India
- Top Career Options in Marketing
- Interview Preparation and Skills
- 73 Google Analytics Interview Q&A
- 56 Social Media Marketing Q&A
- 78 Google AdWords Interview Q&A
- Top 133 SEO Interview Q&A
- 27+ Digital Marketing Q&A
- Digital Marketing Free Course
- Top 9 Skills for PPC Analysts
- Movies with Successful Social Media Campaigns
- Marketing Communication Steps
- Top 10 Reasons to Be an Affiliate Marketer
- Career Options and Paths
- Top 25 Highest Paying Jobs India
- Top 25 Highest Paying Jobs World
- Top 10 Highest Paid Commerce Job
- Career Options After 12th Arts
- Top 7 Commerce Courses Without Maths
- Top 7 Career Options After PCB
- Best Career Options for Commerce
- Career Options After 12th CS
- Top 10 Career Options After 10th
- 8 Best Career Options After BA
- Projects and Academic Pursuits
- 17 Exciting Final Year Projects
- Top 12 Commerce Project Topics
- Top 13 BCA Project Ideas
- Career Options After 12th Science
- Top 15 CS Jobs in India
- 12 Best Career Options After M.Com
- 9 Best Career Options After B.Sc
- 7 Best Career Options After BCA
- 22 Best Career Options After MCA
- 16 Top Career Options After CE
- Courses and Certifications
- 10 Best Job-Oriented Courses
- Best Online Computer Courses
- Top 15 Trending Online Courses
- Top 19 High Salary Certificate Courses
- 21 Best Programming Courses for Jobs
- What is SGPA? Convert to CGPA
- GPA to Percentage Calculator
- Highest Salary Engineering Stream
- 15 Top Career Options After Engineering
- 6 Top Career Options After BBA
- Job Market and Interview Preparation
- Why Should You Be Hired: 5 Answers
- Top 10 Future Career Options
- Top 15 Highest Paid IT Jobs India
- 5 Common Guesstimate Interview Q&A
- Average CEO Salary: Top Paid CEOs
- Career Options in Political Science
- Top 15 Highest Paying Non-IT Jobs
- Cover Letter Examples for Jobs
- Top 5 Highest Paying Freelance Jobs
- Top 10 Highest Paying Companies India
- Career Options and Paths After MBA
- 20 Best Careers After B.Com
- Career Options After MBA Marketing
- Top 14 Careers After MBA In HR
- Top 10 Highest Paying HR Jobs India
- How to Become an Investment Banker
- Career Options After MBA - High Paying
- Scope of MBA in Operations Management
- Best MBA for Working Professionals India
- MBA After BA - Is It Right For You?
- Best Online MBA Courses India
- MBA Project Ideas and Topics
- 11 Exciting MBA HR Project Ideas
- Top 15 MBA Project Ideas
- 18 Exciting MBA Marketing Projects
- MBA Project Ideas: Consumer Behavior
- What is Brand Management?
- What is Holistic Marketing?
- What is Green Marketing?
- Intro to Organizational Behavior Model
- Tech Skills Every MBA Should Learn
- Most Demanding Short Term Courses MBA
- MBA Salary, Resume, and Skills
- MBA Salary in India
- HR Salary in India
- Investment Banker Salary India
- MBA Resume Samples
- Sample SOP for MBA
- Sample SOP for Internship
- 7 Ways MBA Helps Your Career
- Must-have Skills in Sales Career
- 8 Skills MBA Helps You Improve
- Top 20+ SAP FICO Interview Q&A
- MBA Specializations and Comparative Guides
- Why MBA After B.Tech? 5 Reasons
- How to Answer 'Why MBA After Engineering?'
- Why MBA in Finance
- MBA After BSc: 10 Reasons
- Which MBA Specialization to choose?
- Top 10 MBA Specializations
- MBA vs Masters: Which to Choose?
- Benefits of MBA After CA
- 5 Steps to Management Consultant
- 37 Must-Read HR Interview Q&A
- Fundamentals and Theories of Management
- What is Management? Objectives & Functions
- Nature and Scope of Management
- Decision Making in Management
- Management Process: Definition & Functions
- Importance of Management
- What are Motivation Theories?
- Tools of Financial Statement Analysis
- Negotiation Skills: Definition & Benefits
- Career Development in HRM
- Top 20 Must-Have HRM Policies
- Project and Supply Chain Management
- Top 20 Project Management Case Studies
- 10 Innovative Supply Chain Projects
- Latest Management Project Topics
- 10 Project Management Project Ideas
- 6 Types of Supply Chain Models
- Top 10 Advantages of SCM
- Top 10 Supply Chain Books
- What is Project Description?
- Top 10 Project Management Companies
- Best Project Management Courses Online
- Salaries and Career Paths in Management
- Project Manager Salary in India
- Average Product Manager Salary India
- Supply Chain Management Salary India
- Salary After BBA in India
- PGDM Salary in India
- Top 7 Career Options in Management
- CSPO Certification Cost
- Why Choose Product Management?
- Product Management in Pharma
- Product Design in Operations Management
- Industry-Specific Management and Case Studies
- Amazon Business Case Study
- Service Delivery Manager Job
- Product Management Examples
- Product Management in Automobiles
- Product Management in Banking
- Sample SOP for Business Management
- Video Game Design Components
- Top 5 Business Courses India
- Free Management Online Course
- SCM Interview Q&A
- Fundamentals and Types of Law
- Acceptance in Contract Law
- Offer in Contract Law
- 9 Types of Evidence
- Types of Law in India
- Introduction to Contract Law
- Negotiable Instrument Act
- Corporate Tax Basics
- Intellectual Property Law
- Workmen Compensation Explained
- Lawyer vs Advocate Difference
- Law Education and Courses
- LLM Subjects & Syllabus
- Corporate Law Subjects
- LLM Course Duration
- Top 10 Online LLM Courses
- Online LLM Degree
- Step-by-Step Guide to Studying Law
- Top 5 Law Books to Read
- Why Legal Studies?
- Pursuing a Career in Law
- How to Become Lawyer in India
- Career Options and Salaries in Law
- Career Options in Law India
- Corporate Lawyer Salary India
- How To Become a Corporate Lawyer
- Career in Law: Starting, Salary
- Career Opportunities: Corporate Law
- Business Lawyer: Role & Salary Info
- Average Lawyer Salary India
- Top Career Options for Lawyers
- Types of Lawyers in India
- Steps to Become SC Lawyer in India
- Tutorials
- Software Tutorials
- C Tutorials
- Recursion in C: Fibonacci Series
- Checking String Palindromes in C
- Prime Number Program in C
- Implementing Square Root in C
- Matrix Multiplication in C
- Understanding Double Data Type
- Factorial of a Number in C
- Structure of a C Program
- Building a Calculator Program in C
- Compiling C Programs on Linux
- Java Tutorials
- Handling String Input in Java
- Determining Even and Odd Numbers
- Prime Number Checker
- Sorting a String
- User-Defined Exceptions
- Understanding the Thread Life Cycle
- Swapping Two Numbers
- Using Final Classes
- Area of a Triangle
- Skills
- Explore Skills
- Management Skills
- Software Engineering
- JavaScript
- Data Structure
- React.js
- Core Java
- Node.js
- Blockchain
- SQL
- Full stack development
- Devops
- NFT
- BigData
- Cyber Security
- Cloud Computing
- Database Design with MySQL
- Cryptocurrency
- Python
- Digital Marketings
- Advertising
- Influencer Marketing
- Performance Marketing
- Search Engine Marketing
- Email Marketing
- Content Marketing
- Social Media Marketing
- Display Advertising
- Marketing Analytics
- Web Analytics
- Affiliate Marketing
- MBA
- MBA in Finance
- MBA in HR
- MBA in Marketing
- MBA in Business Analytics
- MBA in Operations Management
- MBA in International Business
- MBA in Information Technology
- MBA in Healthcare Management
- MBA In General Management
- MBA in Agriculture
- MBA in Supply Chain Management
- MBA in Entrepreneurship
- MBA in Project Management
- Management Program
- Consumer Behaviour
- Supply Chain Management
- Financial Analytics
- Introduction to Fintech
- Introduction to HR Analytics
- Fundamentals of Communication
- Art of Effective Communication
- Introduction to Research Methodology
- Mastering Sales Technique
- Business Communication
- Fundamentals of Journalism
- Economics Masterclass
- Free Courses
- Home
- Blog
- Data Science
- R Cheat Sheet: The One You Should Keep it Handy
R Cheat Sheet: The One You Should Keep it Handy
Updated on Jul 03, 2023 | 13 min read
Share:
Table of Contents
- Introduction
- Getting help with the programming language R
- Data Transformation Cheat Sheet
- How to import Data into R
- Different data types and the basic manipulation of the tables
- Tips on how to create random data and how to do random sampling
- Tips on how to transform data that is inside the data table
- Conclusion
Introduction
R programming language’s status has grown from being a mere programming language made for statistical analysis to a more potent all-round tool. The user base of R has also grown over the past few years. It is now being employed by a host of programmers, scholars, and practitioners. In order to make the most out of any programming language, learning how to get help is quintessential because errors are bound to happen.
So, with the knowledge of syntax, the knowledge on how to access the R help files and find help from other sources is critical for success as an R programmer. Now, here is where the R cheat sheet will come in handy. The R cheat sheet contains all the vital functions along with its calls for an easy reference of the programmers.
Learn More: R Tutorial for Beginners: Become an Expert in R Programming
Getting help with the programming language R
Even the best books to introduce people and ease their way into the world of programming in R are not enough on their own. Sometimes one needs to learn and access the R help files. This help file that we keep talking about presents the user with a piece of detailed information on how to use various dependencies in R. How to make use of a particular function, for every built-in function is baked into these help files. The code examples on how to use the specific function are also there on each of these different help pages.
If you want to access the R help files, to get help on how to use a particular feature, you will have to use any one of the functions that are listed below:
1. ?: The use of a single question mark displays the help files pertaining to any function that the user desires to get help. For example, “?data.frame” would view the page on the R help files that contain the documentation on how to use the function data.frame().
2. ??: If you want to search for a particular substring in the R help files, “??” will do the job for you. So, if you want to know the names of a function which contains the word “list” in them, all you have to do is run “??list” and your problem would be solved
3. RSiteSearch(): This function RSiteSearch() essentially does what it is named after. It essentially does an online search about the query that is passed as the parameter for this function. So, RSiteSearch(“linear models”) will compile the search at the website “RSiteSearch” for the string “linear models.”
If you are struggling to get help for R and the baked-in documentations are not sitting well with you, there are many add-on packages that you can install to get all the help that you need with R. Packages like “sos” is available for download which is offered by CRAN. This R package contains some clear and concise function which would make the search for all kinds of queries through all the help files available on the website “RSiteSearch.”
The installation of the package is also reasonably straightforward. All that you need to do is run the code install.packages(“sos”) in the R console, then all that is left is to load the package. The package loading can be done through the use of the library(“sos”).
With the installation of the “sos” package, you will now have access to the function called findFn(). This findFn() function takes in the search parameter as the argument and then returns the list of hundreds of the web pages, which contain the argument that has been passed. So, for example, if you run the function findFn (“regression”) into your R console, you will be faced with a web page containing a lot of information.
The information includes links to many functions that have the word regression in the name, or even if they have the phrase regression in their help text, you will also find a reference to it if you use the function findFn().
Read: 6 Interesting R Project Ideas For Beginners
Data Transformation Cheat Sheet
The Data Transformation in R cheat sheet covers how to use the dplyr package to manipulate tidy data. Tidy data is a data format where each variable is a column, each observation is a row, and each value is a cell. The dplyr package provides a set of functions that make it easy to perform common data manipulation tasks, such as filtering, arranging, selecting, mutating, summarizing, and joining data frames. The following are the various functions –
Single-Table Verbs
This section shows how to use the six core functions of dplyr to manipulate a single data frame. These functions are filter(), arrange(), select(), mutate(), summarise(), and group_by(). Each function takes a data frame as the first argument and returns a modified data frame as the output. For example, the filter() function can be used to subset rows based on a condition, the arrange() function can be used to sort rows by one or more variables, and the select() function can be used to choose or rename variables.
Two-Table Verbs
This section shows how to use the four join functions of dplyr to combine two data frames based on a common variable. These functions are inner_join(), left_join(), right_join(), and full_join(). Each function takes two data frames as the first two arguments and returns a joined data frame as the output. For example, the inner_join() function can be used to keep only the rows that match in both data frames. The left_join() function can be used to keep all the rows from the first data frame and add columns from the second data frame, and the full_join() function can be used to keep all the rows from both data frames and fill in missing values with NA.
Grouped Mutates & Filters
This section shows how to use the group_by() function in combination with other dplyr functions to perform operations on grouped data. The group_by() function can be used to group a data frame by one or more variables and create a grouped data frame. A grouped data frame behaves like a regular one, but any operation applied to it will be performed on each group separately. For example, the summarise() function can be used to calculate summary statistics for each group, the mutate() function can be used to create new variables based on group values, and the filter() function can be used to subset groups based on a condition.
Other Useful Functions
This section shows how to use other useful functions from dplyr or related packages to help with data transformation. These functions include rename(), relocate(), slice(), pull(), across(), if_else(), case_when(), and more. Each function has a different purpose and syntax, but they all work with tidy data and follow the same logic as dplyr functions. For example, the rename() function can be used to rename variables in a data frame, the relocate() function can be used to move variables to different positions in a data frame, and the slice() function can be used to select rows by their position.
How to import Data into R
The following table is handy because it contains some functions which will come in very handy when you want to import data into R:
Function | What It Does | Example |
read.table() | This function is responsible for reading the data whose columns are not joined together. Usually, this function is employed when the data that you want to read has its columns separated with a comma or a tab. One thing to note is that you can specify the separator yourself alongside some other different arguments which accurately describe the data you want R to read. | read.table(file=“myfile”, sep=“t”, header=FALSE) |
read.csv() | This function in crude terms is a very toned down or watered-down version of the read.table() method. This function has been hard-coded to read the data from any CSV file that is being passed into this function as an argument. CSV files are typically spreadsheets and MS Excel documents. | read.csv(file=“myfile”) |
read.csv2() | This function is essentially a read.csv() function with minor tweaks. Read.csv2() function has a preset where the separator of the data is a semicolon and the comma serves as the floating or decimal point. | read.csv2(file=“myfile”, header=FALSE) |
read.delim() | This function is used when the main motive is to read the files which have been delimited. The default separator that is being used here is tab. | read.delim(file=“myfile”, header=TRUE) |
scan() | This function gives you a finer and much more precise control over the data that you want to be read by R if the data in question is not tabular. | scan(“myfile”,skip=1, nmax=10) |
readLines() | This function is used when reading one line at a time from a text file is the required job we want the program to perform. | readLines(“myfile”) |
read.fwf | If the data you have has dates in fixed-width-format then you should use this function because it reads the dates in the fixed-width-format. In simpler words, if the data that you have has a fixed number of characters in each column then this function should be used. | read.fwf(“myfile”, widths=c(1,2,3) |
The host of function that you will gain access to after running that line of code and the purpose that they serve are listed below:
Top Data Science Skills You Should Learn
Function | What it does | Example |
read.spss | This function takes in the name of an SPSS file as the argument and reads it into the R program. | read.spss(“myfile”) |
read.dta | This function takes in the input of the file name of Stata binary format and it reads it into the R program. | read.dta(“myfile”) |
read.xport | This function takes the argument of the name of a SAS export file and it reads the file into the R program. | read.export(“myfile”) |
Also check out: Why Learn R? Top 8 Reasons To Learn R
Explore our Data Science Online Certifications
upGrad’s Exclusive Data Science Webinar for you –
Transformation & Opportunities in Analytics & Insights
Different data types and the basic manipulation of the tables
1. There are basically three data types that are of major importance when you are programming in R. These three types are namely: numeric, character, and a factor. You can quickly do a search for which kind of data type is this, or you can also typecast by using the following two commands, respectively, is.factor() and as.factor().
2. If you happen to import a table whose variables contain one or more than one entries, which are characters, then R will automatically cast the table as the datatype of the factor. However, that being said you can still cast the data into numeric by forcing R, using the command= as.numeric(as.character(dat1$VAR1)).
3. The command names (dat1)=c(“ID”, “X”, “Y”, “Z”) actually renames the variable in your dataset. You will have to keep in mind and the vector length should match the number of variables that you have; otherwise, you will run into an error.
4. The command fix (dat2) opens the entire data you have in a spreadsheet document where you can edit the cells with a simple double-clicking in the cells.
5. If the data you have only contains numeric values in the table, you can take the transposition of the table. Use, dat2 = t(dat1), and the table named as dat2 will contain the transpose (making all the rows into columns) of the table of data contained in dat1.
Our learners also read: Top Python Free Courses
Read our popular Data Science Articles
Tips on how to create random data and how to do random sampling
1. The function rnorm(10) takes in the argument of 10 and creates ten random samples. These random samples are generated from a normal distribution, which has a zero mean, and the standard deviation of the dataset happens to be 1.
2. The function runif(10) takes ten different random samples to create a distribution that is uniform and whose value is between zero and one.
3. The function round(rnorm(10)*3+15) takes ten samples, which are random from a normal distribution whose mean is 15, and the standard deviation that it has is of 3 and the floating points which are there in the data are removed with the help of the rounding function.
4. The function round(runif(10)*5+15) gives the user back with random integers, which has the value between the values of 15 and 20. The distribution of these values will be uniform.
5. The function sample(c(“A”, “B”, “C”), 10, replace=TRUE) samples and creates a random sample from any vector that has been passed as the argument to this function.
Learn data science courses from the World’s top Universities. Earn Executive PG Programs, Advanced Certificate Programs, or Masters Programs to fast-track your career.
Tips on how to transform data that is inside the data table
1. The function call of the transform function done like this dat2=transform(dat1, VAR1=VAR1*0.4), multiplies the values stored in VAR1 with 0,4 and then re-assign the multiplied value to VAR1 again.
2. The call of the function transform can also be used to create variables with specific dependencies on existing variables. If you call the function like this dat2=transform(dat1, VAR2=VAR1*2), it will create a new variable with the name of VAR2, which will contain the value of VAR1 multiplied with a factor of two.
3. You can also call the transform function to modify the values at any specific site that you require. For performing that task, you will have to call the function like dat2=transform(dat1, VAR1=ifelse(VAR3== “Site 1”, VAR1*0.4, VAR1)). The call, as mentioned earlier of the transform function, multiplies the data stored in VAR1 for the data entries, which are the place known as site 1. The value of the variable VAR1 remains the same everywhere else.
Read : 8 Astonishing Data Science Projects in R For Beginners
Conclusion
The world of programming has seen a boom of languages over the past few years. These programming languages are aimed to eradicate and focus its attention on one aspect of computing. The languages like R have a robust statistical and data science-centric approach mainly because of the baked-in features that this language possesses.
While working in any programming language, having every command on your fingertips is not an easy task. Now, this is where the R cheat sheet comes to the rescue. One thing to remember always is that the best R cheat sheet is the one that you create.
Frequently Asked Questions (FAQs)
1. What is the meaning of C in the R programming language?
2. What are R functions?
3. What are some of the key features of the R programming language?
Get Free Consultation
By submitting, I accept the T&C and
Privacy Policy
Start Your Career in Data Science Today
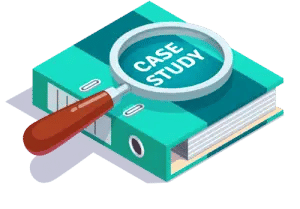