- Blog Categories
- Software Development
- Data Science
- AI/ML
- Marketing
- General
- MBA
- Management
- Legal
- Software Development Projects and Ideas
- 12 Computer Science Project Ideas
- 28 Beginner Software Projects
- Top 10 Engineering Project Ideas
- Top 10 Easy Final Year Projects
- Top 10 Mini Projects for Engineers
- 25 Best Django Project Ideas
- Top 20 MERN Stack Project Ideas
- Top 12 Real Time Projects
- Top 6 Major CSE Projects
- 12 Robotics Projects for All Levels
- Java Programming Concepts
- Abstract Class in Java and Methods
- Constructor Overloading in Java
- StringBuffer vs StringBuilder
- Java Identifiers: Syntax & Examples
- Types of Variables in Java Explained
- Composition in Java: Examples
- Append in Java: Implementation
- Loose Coupling vs Tight Coupling
- Integrity Constraints in DBMS
- Different Types of Operators Explained
- Career and Interview Preparation in IT
- Top 14 IT Courses for Jobs
- Top 20 Highest Paying Languages
- 23 Top CS Interview Q&A
- Best IT Jobs without Coding
- Software Engineer Salary in India
- 44 Agile Methodology Interview Q&A
- 10 Software Engineering Challenges
- Top 15 Tech's Daily Life Impact
- 10 Best Backends for React
- Cloud Computing Reference Models
- Web Development and Security
- Find Installed NPM Version
- Install Specific NPM Package Version
- Make API Calls in Angular
- Install Bootstrap in Angular
- Use Axios in React: Guide
- StrictMode in React: Usage
- 75 Cyber Security Research Topics
- Top 7 Languages for Ethical Hacking
- Top 20 Docker Commands
- Advantages of OOP
- Data Science Projects and Applications
- 42 Python Project Ideas for Beginners
- 13 Data Science Project Ideas
- 13 Data Structure Project Ideas
- 12 Real-World Python Applications
- Python Banking Project
- Data Science Course Eligibility
- Association Rule Mining Overview
- Cluster Analysis in Data Mining
- Classification in Data Mining
- KDD Process in Data Mining
- Data Structures and Algorithms
- Binary Tree Types Explained
- Binary Search Algorithm
- Sorting in Data Structure
- Binary Tree in Data Structure
- Binary Tree vs Binary Search Tree
- Recursion in Data Structure
- Data Structure Search Methods: Explained
- Binary Tree Interview Q&A
- Linear vs Binary Search
- Priority Queue Overview
- Python Programming and Tools
- Top 30 Python Pattern Programs
- List vs Tuple
- Python Free Online Course
- Method Overriding in Python
- Top 21 Python Developer Skills
- Reverse a Number in Python
- Switch Case Functions in Python
- Info Retrieval System Overview
- Reverse a Number in Python
- Real-World Python Applications
- Data Science Careers and Comparisons
- Data Analyst Salary in India
- Data Scientist Salary in India
- Free Excel Certification Course
- Actuary Salary in India
- Data Analyst Interview Guide
- Pandas Interview Guide
- Tableau Filters Explained
- Data Mining Techniques Overview
- Data Analytics Lifecycle Phases
- Data Science Vs Analytics Comparison
- Artificial Intelligence and Machine Learning Projects
- Exciting IoT Project Ideas
- 16 Exciting AI Project Ideas
- 45+ Interesting ML Project Ideas
- Exciting Deep Learning Projects
- 12 Intriguing Linear Regression Projects
- 13 Neural Network Projects
- 5 Exciting Image Processing Projects
- Top 8 Thrilling AWS Projects
- 12 Engaging AI Projects in Python
- NLP Projects for Beginners
- Concepts and Algorithms in AIML
- Basic CNN Architecture Explained
- 6 Types of Regression Models
- Data Preprocessing Steps
- Bagging vs Boosting in ML
- Multinomial Naive Bayes Overview
- Bayesian Network Example
- Bayes Theorem Guide
- Top 10 Dimensionality Reduction Techniques
- Neural Network Step-by-Step Guide
- Technical Guides and Comparisons
- Make a Chatbot in Python
- Compute Square Roots in Python
- Permutation vs Combination
- Image Segmentation Techniques
- Generative AI vs Traditional AI
- AI vs Human Intelligence
- Random Forest vs Decision Tree
- Neural Network Overview
- Perceptron Learning Algorithm
- Selection Sort Algorithm
- Career and Practical Applications in AIML
- AI Salary in India Overview
- Biological Neural Network Basics
- Top 10 AI Challenges
- Production System in AI
- Top 8 Raspberry Pi Alternatives
- Top 8 Open Source Projects
- 14 Raspberry Pi Project Ideas
- 15 MATLAB Project Ideas
- Top 10 Python NLP Libraries
- Naive Bayes Explained
- Digital Marketing Projects and Strategies
- 10 Best Digital Marketing Projects
- 17 Fun Social Media Projects
- Top 6 SEO Project Ideas
- Digital Marketing Case Studies
- Coca-Cola Marketing Strategy
- Nestle Marketing Strategy Analysis
- Zomato Marketing Strategy
- Monetize Instagram Guide
- Become a Successful Instagram Influencer
- 8 Best Lead Generation Techniques
- Digital Marketing Careers and Salaries
- Digital Marketing Salary in India
- Top 10 Highest Paying Marketing Jobs
- Highest Paying Digital Marketing Jobs
- SEO Salary in India
- Content Writer Salary Guide
- Digital Marketing Executive Roles
- Career in Digital Marketing Guide
- Future of Digital Marketing
- MBA in Digital Marketing Overview
- Digital Marketing Techniques and Channels
- 9 Types of Digital Marketing Channels
- Top 10 Benefits of Marketing Branding
- 100 Best YouTube Channel Ideas
- YouTube Earnings in India
- 7 Reasons to Study Digital Marketing
- Top 10 Digital Marketing Objectives
- 10 Best Digital Marketing Blogs
- Top 5 Industries Using Digital Marketing
- Growth of Digital Marketing in India
- Top Career Options in Marketing
- Interview Preparation and Skills
- 73 Google Analytics Interview Q&A
- 56 Social Media Marketing Q&A
- 78 Google AdWords Interview Q&A
- Top 133 SEO Interview Q&A
- 27+ Digital Marketing Q&A
- Digital Marketing Free Course
- Top 9 Skills for PPC Analysts
- Movies with Successful Social Media Campaigns
- Marketing Communication Steps
- Top 10 Reasons to Be an Affiliate Marketer
- Career Options and Paths
- Top 25 Highest Paying Jobs India
- Top 25 Highest Paying Jobs World
- Top 10 Highest Paid Commerce Job
- Career Options After 12th Arts
- Top 7 Commerce Courses Without Maths
- Top 7 Career Options After PCB
- Best Career Options for Commerce
- Career Options After 12th CS
- Top 10 Career Options After 10th
- 8 Best Career Options After BA
- Projects and Academic Pursuits
- 17 Exciting Final Year Projects
- Top 12 Commerce Project Topics
- Top 13 BCA Project Ideas
- Career Options After 12th Science
- Top 15 CS Jobs in India
- 12 Best Career Options After M.Com
- 9 Best Career Options After B.Sc
- 7 Best Career Options After BCA
- 22 Best Career Options After MCA
- 16 Top Career Options After CE
- Courses and Certifications
- 10 Best Job-Oriented Courses
- Best Online Computer Courses
- Top 15 Trending Online Courses
- Top 19 High Salary Certificate Courses
- 21 Best Programming Courses for Jobs
- What is SGPA? Convert to CGPA
- GPA to Percentage Calculator
- Highest Salary Engineering Stream
- 15 Top Career Options After Engineering
- 6 Top Career Options After BBA
- Job Market and Interview Preparation
- Why Should You Be Hired: 5 Answers
- Top 10 Future Career Options
- Top 15 Highest Paid IT Jobs India
- 5 Common Guesstimate Interview Q&A
- Average CEO Salary: Top Paid CEOs
- Career Options in Political Science
- Top 15 Highest Paying Non-IT Jobs
- Cover Letter Examples for Jobs
- Top 5 Highest Paying Freelance Jobs
- Top 10 Highest Paying Companies India
- Career Options and Paths After MBA
- 20 Best Careers After B.Com
- Career Options After MBA Marketing
- Top 14 Careers After MBA In HR
- Top 10 Highest Paying HR Jobs India
- How to Become an Investment Banker
- Career Options After MBA - High Paying
- Scope of MBA in Operations Management
- Best MBA for Working Professionals India
- MBA After BA - Is It Right For You?
- Best Online MBA Courses India
- MBA Project Ideas and Topics
- 11 Exciting MBA HR Project Ideas
- Top 15 MBA Project Ideas
- 18 Exciting MBA Marketing Projects
- MBA Project Ideas: Consumer Behavior
- What is Brand Management?
- What is Holistic Marketing?
- What is Green Marketing?
- Intro to Organizational Behavior Model
- Tech Skills Every MBA Should Learn
- Most Demanding Short Term Courses MBA
- MBA Salary, Resume, and Skills
- MBA Salary in India
- HR Salary in India
- Investment Banker Salary India
- MBA Resume Samples
- Sample SOP for MBA
- Sample SOP for Internship
- 7 Ways MBA Helps Your Career
- Must-have Skills in Sales Career
- 8 Skills MBA Helps You Improve
- Top 20+ SAP FICO Interview Q&A
- MBA Specializations and Comparative Guides
- Why MBA After B.Tech? 5 Reasons
- How to Answer 'Why MBA After Engineering?'
- Why MBA in Finance
- MBA After BSc: 10 Reasons
- Which MBA Specialization to choose?
- Top 10 MBA Specializations
- MBA vs Masters: Which to Choose?
- Benefits of MBA After CA
- 5 Steps to Management Consultant
- 37 Must-Read HR Interview Q&A
- Fundamentals and Theories of Management
- What is Management? Objectives & Functions
- Nature and Scope of Management
- Decision Making in Management
- Management Process: Definition & Functions
- Importance of Management
- What are Motivation Theories?
- Tools of Financial Statement Analysis
- Negotiation Skills: Definition & Benefits
- Career Development in HRM
- Top 20 Must-Have HRM Policies
- Project and Supply Chain Management
- Top 20 Project Management Case Studies
- 10 Innovative Supply Chain Projects
- Latest Management Project Topics
- 10 Project Management Project Ideas
- 6 Types of Supply Chain Models
- Top 10 Advantages of SCM
- Top 10 Supply Chain Books
- What is Project Description?
- Top 10 Project Management Companies
- Best Project Management Courses Online
- Salaries and Career Paths in Management
- Project Manager Salary in India
- Average Product Manager Salary India
- Supply Chain Management Salary India
- Salary After BBA in India
- PGDM Salary in India
- Top 7 Career Options in Management
- CSPO Certification Cost
- Why Choose Product Management?
- Product Management in Pharma
- Product Design in Operations Management
- Industry-Specific Management and Case Studies
- Amazon Business Case Study
- Service Delivery Manager Job
- Product Management Examples
- Product Management in Automobiles
- Product Management in Banking
- Sample SOP for Business Management
- Video Game Design Components
- Top 5 Business Courses India
- Free Management Online Course
- SCM Interview Q&A
- Fundamentals and Types of Law
- Acceptance in Contract Law
- Offer in Contract Law
- 9 Types of Evidence
- Types of Law in India
- Introduction to Contract Law
- Negotiable Instrument Act
- Corporate Tax Basics
- Intellectual Property Law
- Workmen Compensation Explained
- Lawyer vs Advocate Difference
- Law Education and Courses
- LLM Subjects & Syllabus
- Corporate Law Subjects
- LLM Course Duration
- Top 10 Online LLM Courses
- Online LLM Degree
- Step-by-Step Guide to Studying Law
- Top 5 Law Books to Read
- Why Legal Studies?
- Pursuing a Career in Law
- How to Become Lawyer in India
- Career Options and Salaries in Law
- Career Options in Law India
- Corporate Lawyer Salary India
- How To Become a Corporate Lawyer
- Career in Law: Starting, Salary
- Career Opportunities: Corporate Law
- Business Lawyer: Role & Salary Info
- Average Lawyer Salary India
- Top Career Options for Lawyers
- Types of Lawyers in India
- Steps to Become SC Lawyer in India
- Tutorials
- Software Tutorials
- C Tutorials
- Recursion in C: Fibonacci Series
- Checking String Palindromes in C
- Prime Number Program in C
- Implementing Square Root in C
- Matrix Multiplication in C
- Understanding Double Data Type
- Factorial of a Number in C
- Structure of a C Program
- Building a Calculator Program in C
- Compiling C Programs on Linux
- Java Tutorials
- Handling String Input in Java
- Determining Even and Odd Numbers
- Prime Number Checker
- Sorting a String
- User-Defined Exceptions
- Understanding the Thread Life Cycle
- Swapping Two Numbers
- Using Final Classes
- Area of a Triangle
- Skills
- Explore Skills
- Management Skills
- Software Engineering
- JavaScript
- Data Structure
- React.js
- Core Java
- Node.js
- Blockchain
- SQL
- Full stack development
- Devops
- NFT
- BigData
- Cyber Security
- Cloud Computing
- Database Design with MySQL
- Cryptocurrency
- Python
- Digital Marketings
- Advertising
- Influencer Marketing
- Performance Marketing
- Search Engine Marketing
- Email Marketing
- Content Marketing
- Social Media Marketing
- Display Advertising
- Marketing Analytics
- Web Analytics
- Affiliate Marketing
- MBA
- MBA in Finance
- MBA in HR
- MBA in Marketing
- MBA in Business Analytics
- MBA in Operations Management
- MBA in International Business
- MBA in Information Technology
- MBA in Healthcare Management
- MBA In General Management
- MBA in Agriculture
- MBA in Supply Chain Management
- MBA in Entrepreneurship
- MBA in Project Management
- Management Program
- Consumer Behaviour
- Supply Chain Management
- Financial Analytics
- Introduction to Fintech
- Introduction to HR Analytics
- Fundamentals of Communication
- Art of Effective Communication
- Introduction to Research Methodology
- Mastering Sales Technique
- Business Communication
- Fundamentals of Journalism
- Economics Masterclass
- Free Courses
- Home
- Blog
- Data Science
- Top 30 Data Science Tools: Benefits and How to Choose the Right Tool for Your Needs in 2025
Top 30 Data Science Tools: Benefits and How to Choose the Right Tool for Your Needs in 2025
Updated on Jan 13, 2025 | 23 min read
Share:
Table of Contents
Data science is reshaping industries by enabling businesses to analyze vast datasets, predict trends, and drive data-informed decisions. To achieve these goals, data science tools play a pivotal role, offering functionalities like data preprocessing, visualization, and advanced machine learning capabilities.
However, selecting the right tools can be challenging due to the sheer variety of options. This guide explores the top 30 data science tools and technologies for 2025, their benefits and applications. Dive right in!
Top 30 Data Science Tools You Should Know
Data science tools are essential for extracting, analyzing, and visualizing data. Each tool has unique strengths and applications, catering to various data science needs. Below is a list of 30 tools and their overviews to help you understand how they can benefit your projects.
Tool |
Overview |
MS Excel | Widely-used spreadsheet software for data analysis and visualization. |
Google Sheets | Cloud-based spreadsheet application with real-time collaboration features. |
Numbers (Apple) | Apple's spreadsheet software designed for data organization and analysis. |
LibreOffice Calc | Free, open-source spreadsheet program offering various data analysis functions. |
Apache Spark | Unified analytics engine for large-scale data processing with built-in modules for SQL, streaming, and machine learning. |
MATLAB | High-level language and environment for numerical computation and visualization. |
SAS | Statistical software suite for advanced analytics, business intelligence, and data management. |
KNIME | Open-source platform for data integration, processing, analysis, and exploration. |
Apache Flink | Stream-processing framework for distributed, high-performing, always-available, and accurate data streaming applications. |
RapidMiner Studio | Data science platform for building and deploying predictive models with a visual workflow designer. |
Orange Data Mining | Open-source data visualization and analysis tool for both novices and experts, through visual programming or Python scripting. |
Weka | Collection of machine learning algorithms for data mining tasks, offering tools for data pre-processing, classification, regression, clustering, and visualization. |
BigML | Cloud-based machine learning platform providing a range of algorithms for predictive modeling. |
Google Analytics | Web analytics service offering statistics and analytical tools for marketing and SEO purposes. |
Amazon SageMaker | Fully managed service that provides every developer and data scientist with the ability to build, train, and deploy machine learning models quickly. |
Azure Machine Learning | Cloud-based service for building, training, and deploying machine learning models. |
Google Cloud AI Platform | Suite of cloud services for training, hosting, and managing machine learning models. |
Python | High-level programming language renowned for its readability and extensive libraries for data analysis and machine learning. |
R Programming | Language and environment specifically designed for statistical computing and graphics. |
Jupyter Notebook | Open-source web application that allows you to create and share documents containing live code, equations, visualizations, and narrative text. |
MongoDB | NoSQL database program that uses JSON-like documents with optional schemas, ideal for handling large volumes of diverse data. |
D3.js | JavaScript library for producing dynamic, interactive data visualizations in web browsers. |
Julia | High-level, high-performance programming language for technical computing, with syntax familiar to users of other technical computing environments. |
Scala | General-purpose programming language providing support for functional programming and a strong static type system. |
Java | High-level, class-based, object-oriented programming language widely used for building enterprise-scale applications. |
C++ | General-purpose programming language with low-level memory manipulation features, suitable for performance-critical applications. |
Tableau | Interactive data visualization software focused on business intelligence. |
Matplotlib | Comprehensive library for creating static, animated, and interactive visualizations in Python. |
Minitab | Statistical software designed for data analysis, including tools for statistical modeling and graphical analysis. |
Power BI | Business analytics service by Microsoft providing interactive visualizations and business intelligence capabilities with an interface simple enough for end users to create their own reports and dashboards. |
Seaborn | Python data visualization library based on Matplotlib, providing a high-level interface for drawing attractive statistical graphics. |
Plotly | Graphing library that makes interactive, publication-quality graphs online, supporting several programming languages including Python and JavaScript. |
Now, let's dive deeper into each category to understand these tools better, starting with Data Science Software and General-purpose Tools, which are foundational to any data science workflow.
Data Science Software and General-Purpose Tools
Data science software and general-purpose tools are essential for managing and analyzing data. Versatile and user-friendly, they support tasks like data cleaning, organization, and visualization. Particularly suited for foundational tasks and quick exploratory analysis, these tools are ideal for beginners and experienced data scientists alike. From spreadsheets to specialized platforms, they form the backbone of many workflows, offering both simplicity and robust functionality.
1. MS Excel
MS Excel is a trusted tool in data science, known for its ease of use and wide range of functions. It allows users to clean, analyze, and visualize data quickly.
Usage
MS Excel is best suited for small to medium-sized datasets and quick exploratory analysis.
Key Features
- Built-in data manipulation tools
- Charting and visualization capabilities
- Macro support for repetitive tasks
Industry Use Cases
Enhances decision-making in business settings by enabling the creation of financial models, generation of detailed reports, and efficient organization of datasets.
2. Google Sheets
Google Sheets is a cloud-based spreadsheet tool designed for collaboration and real-time data analysis.
Usage
Perfect for collaborative data entry, sharing, and basic analysis tasks.
Key Features
- Real-time editing and sharing
- Integration with Google Workspace tools
- Support for add-ons to extend functionality
Industry Use Cases
Improves team productivity by reducing data discrepancies through real-time updates, making it ideal for tracking project metrics and performing collaborative data cleaning.
Also Read: How to Use IMPORTRANGE Functions in Google Sheets?
3. Numbers (Apple)
Numbers is a spreadsheet application tailored for Apple users, offering a sleek interface and seamless integration with macOS and iOS.
- Usage
Ideal for users in the Apple ecosystem looking for intuitive data organization and visually appealing presentations. - Key Features
- Pre-built templates for professional documents
- Real-time collaboration via iCloud
- Interactive charts and graphs
- Industry Use Cases
Enhances presentation quality with polished reports and visually engaging charts, commonly used by small teams and individual professionals.
Also Read: Understanding Types of Data: Why is Data Important, its 4 Types, Job Prospects, and More
4. LibreOffice Calc
LibreOffice Calc is an open-source alternative to proprietary spreadsheet software, offering powerful features without any cost.
- Usage
Suitable for users seeking cost-effective solutions for data manipulation and analysis. - Key Features
- Extensive formula library
- Data plotting and charting tools
- Support for multiple file formats
- Industry Use Cases
Widely adopted by budget-conscious users and organizations for tasks like statistical calculations, report generation, and organizing datasets.
Also Read: Ultimate Guide to Work with Excel Spreadsheets Using Python
5. Apache Spark
Apache Spark is a powerful analytics engine designed for processing large-scale data across distributed systems.
- Usage
Best suited for big data applications requiring high-speed computation and in-memory processing. - Key Features
- Scalable and fault-tolerant architecture
- Modules for machine learning, streaming, and SQL
- Multi-language support (Python, Java, Scala)
- Industry Use Cases
Frequently used in industries like finance and telecommunications for real-time analytics, large-scale data transformations, and predictive modeling.
Also Read: Top 3 Apache Spark Applications / Industry Use Cases & Why It Matters
6. MATLAB
MATLAB is a high-level programming environment known for its numerical computation and data visualization capabilities.
- Usage
Ideal for tasks requiring advanced mathematical modeling, algorithm development, and simulation. - Key Features
- Built-in mathematical functions and toolboxes
- Advanced data visualization and plotting tools
- Seamless integration with hardware for real-time testing
- Industry Use Cases
Commonly used in academia and industries like engineering and robotics for tasks like signal processing, control systems, and prototyping.
Also Read: 21 Best Ideas for MATLAB Projects & Topics For Beginners [2025] | MATLAB Data Types: Everything You Need to Know
7. SAS
SAS (Statistical Analysis System) is a comprehensive platform for advanced analytics, business intelligence, and data management.
- Usage
Designed for handling large-scale data analysis in enterprise environments. - Key Features
- Extensive library of statistical tools
- Advanced data integration and transformation capabilities
- Robust security and governance features
- Industry Use Cases
Widely used in industries like healthcare, banking, and insurance for predictive modeling, fraud detection, and risk management.
Also Read: Fraud Detection in Machine Learning: What You Need To Know | Credit Card Fraud Detection Project – Machine Learning Project
8. KNIME
KNIME is an open-source platform for creating data workflows and conducting advanced analytics through a visual interface.
- Usage
Perfect for users looking for a drag-and-drop interface to design and execute data science workflows. - Key Features
- Integration with popular machine learning libraries
- Workflow automation and reusable components
- Support for Python, R, and Java
- Industry Use Cases
Commonly used for data preprocessing, machine learning model development, and text mining in academic and business settings.
Also Read: Python vs Java: Which One Should You Master for Your Career? | R vs Python Data Science: The Difference
9. Apache Flink
Apache Flink is a stream-processing framework designed for high-performance, distributed, and fault-tolerant data applications.
- Usage
Best suited for real-time data processing and streaming analytics. - Key Features
- Low-latency and high-throughput capabilities
- Advanced event-driven processing features
- Compatibility with major big data ecosystems
- Industry Use Cases
Often used in IoT applications, real-time fraud detection, and monitoring systems across industries like telecommunications and finance.
Also Read: Flink Vs. Spark: Difference Between Flink and Spark
10. RapidMiner Studio
RapidMiner Studio is a comprehensive data science platform offering tools for building and deploying predictive models.
- Usage
Ideal for data scientists and analysts seeking a visual workflow environment for model development. - Key Features
- Pre-built machine learning algorithms
- Integration with big data and cloud platforms
- Easy deployment of models in production
- Industry Use Cases
Frequently used for customer segmentation, demand forecasting, and predictive analytics in marketing and retail.
Also Read: 7 Most Used Machine Learning Algorithms in Python You Should Know About
11. Orange Data Mining
Orange Data Mining is an open-source tool that simplifies data mining and machine learning through visual programming.
- Usage
Suitable for beginners and experts who want to analyze data without extensive coding. - Key Features
- Interactive data visualization tools
- Support for machine learning and text mining
- Modular design for adding custom functionality
- Industry Use Cases
Often used in education, research, and business for clustering, classification, and feature analysis.
Also Read: What is Text Mining in Data Mining? Steps, Techniques Used, Real-world Applications & Challenges
12. Weka
Weka is a collection of machine learning algorithms and tools for data mining tasks, designed for both beginners and professionals.
- Usage
Ideal for tasks like data preprocessing, classification, clustering, and regression. - Key Features
- User-friendly graphical interface
- Support for various machine learning algorithms
- Integration with other tools through Java APIs
- Industry Use Cases
Commonly used in academic research and teaching for algorithm evaluation, feature selection, and predictive modeling.
While general-purpose tools handle foundational tasks, cloud-based data science tools excel in scalability and collaboration, making them essential for modern workflows. Here are some of the top cloud-based tools.
Also Read: How to Become a Data Scientist – Answer in 9 Easy Steps
Cloud-based Data Science Tools
Data science is increasingly moving to the cloud, addressing modern challenges like managing distributed teams and massive datasets. Cloud-based tools provide the flexibility to store, process, and analyze large datasets while enabling seamless collaboration and real-time processing.
With robust machine learning capabilities and easy integration, these tools are indispensable for efficient, scalable workflows.
13. BigML
BigML is a cloud-based machine learning platform that simplifies predictive modeling through an intuitive interface.
- Usage
Suitable for building machine learning models without extensive programming experience. - Key Features
- Pre-built models for classification, regression, and clustering
- Real-time predictions via REST APIs
- Visual workflows for streamlined model creation
- Industry Use Cases
Frequently used in education, customer analytics, and risk assessment projects.
Also Read: 4 Key Benefits of Machine Learning in Cloud: Everything You Need to Know
14. Google Analytics
Google Analytics is a web analytics service that helps track and report website traffic and user behavior.
- Usage
Ideal for marketers and analysts who need actionable insights into website performance. - Key Features
- Real-time tracking of website activity
- Audience segmentation and behavior analysis
- Integration with Google Ads and other marketing tools
- Industry Use Cases
Commonly used to optimize marketing campaigns, track user engagement, and improve website usability.
Also Read: How to Use Google Analytics: Comprehensive Guide For Beginners
15. Amazon SageMaker
Amazon SageMaker is a fully managed service that enables developers and data scientists to build, train, and deploy machine learning models.
- Usage
Best suited for organizations seeking an end-to-end machine learning solution in the cloud. - Key Features
- Built-in algorithms for training and deployment
- Support for custom models using popular frameworks
- Automatic model tuning and monitoring
- Industry Use Cases
Used widely in e-commerce, healthcare, and finance for fraud detection, personalized recommendations, and predictive maintenance.
Also Read: Why Do We Need AWS Sagemaker?
16. Azure Machine Learning
Azure Machine Learning is a cloud-based service for building, deploying, and managing machine learning models.
- Usage
Designed for professionals working on machine learning projects with seamless integration into the Microsoft ecosystem. - Key Features
- Automated machine learning for quick model building
- End-to-end lifecycle management
- Compatibility with open-source frameworks
- Industry Use Cases
Commonly applied in industries like energy, manufacturing, and retail for demand forecasting and anomaly detection.
Also Read: Azure Databricks: Everything You Need to Know
17. Google Cloud AI Platform
Google Cloud AI Platform is a suite of AI and machine learning services designed to handle the entire model development lifecycle.
- Usage
Ideal for businesses and researchers aiming to scale AI solutions with minimal overhead. - Key Features
- Pre-trained models and APIs for quick deployment
- Scalable training infrastructure for large datasets
- Integration with other Google Cloud services
- Industry Use Cases
Frequently used for natural language processing, image recognition, and predictive analytics.
Also Read: AWS v/s Google v/s Azure: Who will win the Cloud War?
While cloud-based tools excel in scalability and accessibility, programming language-driven tools and technologies offer unmatched flexibility and control. Here’s a look at the top tools on the list.
Programming Language-driven Data Science Tools and Technologies
Programming languages offer unmatched flexibility for creating custom solutions, experimenting with advanced algorithms, and tackling complex analytical challenges. With extensive libraries and frameworks, these tools empower both beginners and experts to handle everything from data manipulation to machine learning with precision and control.
18. Python
Python is a versatile and beginner-friendly programming language widely used in data science for its simplicity and robust library ecosystem.
- Usage
Suitable for data analysis, machine learning, and automation tasks. - Key Features
- Extensive libraries like Pandas, NumPy, and Scikit-learn
- Support for visualization with Matplotlib and Seaborn
- Broad community support and open-source resources
- Industry Use Cases
Commonly applied in industries like finance, healthcare, and tech for data modeling, machine learning, and web scraping.
19. R Programming
R is a language specifically designed for statistical computing and graphics, making it a favorite among statisticians and data analysts.
- Usage
Ideal for complex statistical modeling, data visualization, and reporting. - Key Features
- Libraries like ggplot2 and dplyr for data manipulation and visualization
- Built-in support for statistical tests and machine learning algorithms
- Integration with Markdown for creating dynamic reports
- Industry Use Cases
Frequently used in academia, research, and healthcare for tasks like clinical trial analysis, survey data visualization, and predictive modeling.
Also Read: R Tutorial for Beginners: Become an Expert in R Programming | R For Data Science: Why Should You Choose R for Data Science?
20. Jupyter Notebook
Jupyter Notebook is an open-source web application that allows users to create and share documents containing live code, equations, and visualizations.
- Usage
Ideal for creating reproducible workflows and sharing results interactively. - Key Features
- Supports multiple programming languages, including Python, R, and Julia
- Combines code, output, and narrative text in one document
- Extensible with widgets and plugins
- Industry Use Cases
Widely used in education, collaborative research, and exploratory data analysis.
Also Read: What Is Exploratory Data Analysis in Data Science? Tools, Process & Types
21. MongoDB
MongoDB is a NoSQL database program that stores data in a flexible, JSON-like format, making it ideal for handling unstructured data.
- Usage
Best suited for applications requiring fast, scalable, and schema-less data storage. - Key Features
- High scalability and performance
- Support for real-time analytics and aggregation
- Easy integration with modern programming languages
- Industry Use Cases
Commonly used in e-commerce, IoT, and mobile applications for managing product catalogs, logs, and user data.
22. D3.js
D3.js is a JavaScript library for creating dynamic, interactive data visualizations directly in web browsers.
- Usage
Designed for building custom visualizations that require precision and interactivity. - Key Features
- Data binding to HTML, SVG, and CSS
- Support for animations and transitions
- Flexibility to create unique, interactive visualizations
- Industry Use Cases
Frequently used in journalism, marketing, and web development to create engaging visual data stories.
Also Read: Top 10 JavaScript Libraries to Learn | 10 Practical Applications of JavaScript And Career Tips
23. Julia
Julia is a high-performance programming language tailored for numerical and computational tasks, offering a syntax familiar to Python and MATLAB users.
- Usage
Suitable for tasks requiring high-speed computations, such as numerical simulations and large-scale modeling. - Key Features
- Optimized for parallel computing and scalability
- Extensive library ecosystem for scientific computing
- Easy integration with Python, R, and C++
- Industry Use Cases
Commonly applied in fields like physics, finance, and engineering for solving complex equations and simulations.
24. Scala
Scala is a general-purpose programming language that integrates object-oriented and functional programming paradigms, making it popular in big data applications.
- Usage
Ideal for processing large-scale data and developing distributed systems. - Key Features
- Interoperability with Java
- Libraries for data science and machine learning, such as Breeze and SparkML
- Support for asynchronous and parallel computing
- Industry Use Cases
Widely used in industries like ad tech and finance for big data analytics and real-time processing.
Also Read: Build your Career with Currying in Scala | Java vs Scala: Key Differences. Similarities and Which One to Choose in 2025
25. Java
Java is a versatile programming language known for its stability and scalability, commonly used for enterprise-level applications.
- Usage
Best suited for developing robust data pipelines and scalable machine learning models. - Key Features
- Cross-platform compatibility
- Rich library ecosystem for data processing and analytics
- Strong support for integration with big data tools like Hadoop and Spark
- Industry Use Cases
Often applied in industries like banking and telecommunications for fraud detection, credit scoring, and risk management.
26. C++
C++ is a powerful programming language known for its performance and control, making it suitable for computationally intensive tasks.
- Usage
Ideal for developing custom algorithms and performance-critical applications. - Key Features
- Low-level memory manipulation for optimized performance
- Libraries for scientific computing and machine learning
- Broad compatibility with various platforms and devices
- Industry Use Cases
Frequently used in robotics, gaming, and scientific research for real-time systems and simulations.
Also Read: Top 12 Data Science Programming Languages 2025 [Hand-Picked] | Top Python IDEs: Choosing the Best IDE for Your Python Development Needs
After working with programming language-driven tools to process and analyze data, the next step is presenting your findings effectively. Visualization data science tools are essential for transforming raw data and analysis into clear, impactful visuals. Let’s take a look at some of them.
Visualization Data Science Tools
Data visualization tools transform raw data into meaningful visuals, making it easier to understand trends, patterns, and insights. Visualization tools play a crucial role in turning raw data into actionable insights, enabling clear communication of trends and patterns to support data-driven decision-making and storytelling. These tools are essential for effectively presenting findings and driving informed decisions, whether you’re a beginner or an expert.
27. Tableau
Tableau is an interactive data visualization software that enables users to create dashboards and share insights seamlessly.
- Usage
Best suited for creating interactive dashboards and visual analytics. - Key Features
- Drag-and-drop interface for building visualizations
- Integration with various data sources
- Advanced analytics with no programming required
- Industry Use Cases
Widely used in business intelligence, marketing, and sales for tracking KPIs, analyzing trends, and visualizing large datasets.
Also Read: What is Tableau? Features, Functions & Data Visualizations [With Examples] | Tableau Tutorial For Beginners: Learn Tableau Step by Step
28. Matplotlib
Matplotlib is a comprehensive Python library for creating static, animated, and interactive visualizations.
- Usage
Ideal for creating detailed plots and charts in Python-based data workflows. - Key Features
- Extensive customization options for visualizations
- Compatibility with other Python libraries
- Support for 2D and limited 3D plotting
- Industry Use Cases
Frequently used in academic research, technical reporting, and exploratory data analysis.
Also Read: Matplotlib in Python: Explained Various Plots with Examples | Matplotlib in Python: A Detailed Understanding on Functionalities and Installation
29. Power BI
Power BI is a business analytics service by Microsoft that offers tools for creating interactive visualizations and reports.
- Usage
Designed for professionals who need to create shareable business intelligence dashboards. - Key Features
- Integration with Microsoft products and third-party services
- Real-time data updates and monitoring
- AI-driven insights for enhanced decision-making
- Industry Use Cases
Commonly applied in business settings for financial reporting, sales analysis, and operational performance tracking.
Also Read: Power BI Architecture: Components, Function, Benefits & Applications
30. Seaborn
Seaborn is a Python-based data visualization library built on top of Matplotlib, offering a high-level interface for creating statistical graphics.
- Usage
Best suited for producing attractive, informative plots with minimal code. - Key Features
- Built-in themes for consistent aesthetics
- Simplified APIs for complex plots
- Integrated support for pandas data structures
- Industry Use Cases
Commonly used in data analysis pipelines for creating heatmaps, pair plots, and distribution visualizations.
Also Read: Data Visualisation: The What, The Why, and The How! | Top 10 Data Visualization Techniques for Successful Presentations
Advance your career with the Post Graduate Diploma in Data Science from IIIT Bangalore. Gain industry-relevant skills, learn from top faculty, and work on real-world projects to master the field of data science. Apply Now to secure your spot in this sought-after program and take a giant leap toward a data science career!
Now we have explored all the top 30 data science tools. But why should you rely on these tools in the first place? Let’s get into the key reasons why using data science tools in 2025 is more important than ever.
upGrad’s Exclusive Data Science Webinar for you –
How upGrad helps for your Data Science Career?
5 Key Reasons to Use Data Science Tools in 2025
Data science tools have become essential for analyzing and interpreting information effectively. They simplify complex processes, allowing you to focus on uncovering valuable insights and presenting them clearly. As businesses increasingly rely on data, tools in 2025 offer advanced capabilities like real-time insights and AI-driven automation to maintain a competitive edge. Here are five key reasons why these tools are critical for success in 2025:
1. Efficient Data Handling
Managing large datasets can quickly become overwhelming without the right tools. Data science tools simplify this process, making it more manageable and efficient.
- Tools like MS Excel and Google Sheets help organize and clean smaller datasets.
- Big data platforms such as Apache Spark handle massive datasets with ease.
- Features like automated error-checking, data cleaning algorithms, and batch processing in data science software reduce manual effort, saving time and ensuring consistency.
With these tools, you can focus on extracting insights instead of getting bogged down in repetitive tasks.
Also Read: What is Big Data? A Comprehensive Guide to Big Data and Big Data Analytics
2. Enhanced Analytical Accuracy
Accuracy is key to any data analysis project, and data science tools ensure precision at every step.
- Programming tools like Python and R offer powerful libraries for statistical modeling and machine learning, reducing the risk of human error.
- Specialized software like SAS and MATLAB ensures calculations are both reliable and efficient.
- Built-in error-checking and validation features in many data science technologies help maintain the integrity of your results.
By using these tools, you can trust that your analyses are accurate and actionable.
Also Read: What is Predictive Analysis? Why is it Important? | Sentiment Analysis: What is it and Why Does it Matter?
3. Advanced Visualization Capabilities
Data science tools and technologies play a crucial role in transforming raw data into visuals that communicate effectively.
- Tools like Tableau and Power BI create interactive dashboards for presenting real-time insights.
- Libraries like Matplotlib and Seaborn in Python allow you to produce detailed, customized plots.
- Visualization-focused data science software simplifies complex trends, making them more accessible to non-technical audiences.
These tools help bridge the gap between raw data and impactful storytelling, ensuring your audience understands the bigger picture.
4. Accessibility and Collaboration
In 2025, collaboration is central to solving data problems, and cloud-based data science tools are designed to support teamwork.
- Platforms like Google Cloud AI and Azure Machine Learning allow you to build and share models seamlessly.
- Cloud-based spreadsheet tools like Google Sheets enable real-time collaboration on data cleaning and analysis.
- Scalable cloud technologies ensure your team can access and work on large datasets from anywhere.
These tools make teamwork more productive, enabling you to deliver results faster and more effectively.
5. Staying Competitive in a Rapidly Evolving Field
The field of data science continues to expand, and staying competitive means keeping up with the latest tools and technologies.
- Tools like Jupyter Notebook, Python, and KNIME enhance your ability to tackle diverse projects, from machine learning to predictive analytics.
- Visualization tools like Tableau and Power BI are increasingly sought-after in the job market.
- As demand for skilled professionals grows, mastering these data science tools and software ensures you remain relevant and ready for future challenges.
Investing in your proficiency with these tools isn’t just about staying competitive—it’s about excelling in the evolving world of data science.
Now that we understand the importance of these tools, let’s explore how to select the right one for your needs.
How to Select the Right Type of Data Science Tool for Your Needs
Selecting the right tools not only enhances productivity but also ensures the best fit for your data's complexity and project goals. by focusing on your specific needs, goals, and the nature of your data, you can narrow down your choices effectively.
Here’s a guide to help you make informed decisions when selecting the right tools, software, and technologies for your data science projects:
1. Understand the Type and Size of Your Data
The characteristics of your dataset play a crucial role in determining the tools you need.
- For small to medium-sized datasets, general-purpose tools like MS Excel or Google Sheets are sufficient.
- For large-scale data processing, consider big data platforms like Apache Spark or cloud-based tools like Google Cloud AI.
- If your data is unstructured (e.g., text, images), explore tools like MongoDB or Python libraries designed for handling such data.
Also Read: Characteristics of Big Data: Types & 5V’s
2. Define Your Analysis Goals
Clarifying your objectives will help you identify tools that align with your end goals.
- For statistical modeling and predictive analytics, consider R Programming, Python, or SAS.
- If your goal is to visualize trends and insights, tools like Tableau, Power BI, or Matplotlib are excellent choices.
- For machine learning tasks, platforms like Amazon SageMaker or Azure Machine Learning offer robust capabilities.
Also Read: How to Become a Data Analyst with No Experience in 2025?: A Complete Roadmap
3. Assess Your Level of Expertise
Your familiarity with programming and data science tools can guide your selection process.
- Beginners may prefer tools with a user-friendly interface, such as KNIME or RapidMiner Studio.
- Experienced users can benefit from the flexibility of programming-driven tools like Python, R, or Julia.
- If you’re looking for a balance, hybrid tools like Jupyter Notebook combine usability with advanced features.
Also Read: Top 30 Data Analytics Project Ideas to Elevate Your Skills |Want to Be a Data Analyst? Here are Top Skills & Tools to Master
4. Consider Collaboration and Accessibility
If you’re working in a team or require remote access, collaboration-friendly tools are essential.
- Cloud-based platforms like Google Sheets or Amazon SageMaker enable real-time collaboration.
- Tools like KNIME and Tableau offer easy sharing of workflows and dashboards.
- Ensure the tool you choose integrates well with your team’s existing technologies.
5. Budget and Scalability
Evaluate your budget and how the tool fits into your long-term plans.
- Free or open-source tools like LibreOffice Calc, Python, and R are great for budget-conscious learners.
- Paid options like MATLAB or Tableau provide advanced features but may require a subscription or license.
- For growing datasets and complex tasks, ensure the tool you choose can scale to meet future demands.
6. Explore Compatibility with Your Workflow
A good data science tool should integrate smoothly with the other technologies you use.
- Check for compatibility with your operating system, data storage solutions, or programming languages.
- Tools like Python and Power BI are versatile and integrate well with various platforms and data sources.
Also Read: What are Data Structures & Algorithm? | 14 Fascinating Data Analytics Real-Life Applications
How upGrad Can Support Your Data Science Learning Journey?
At upGrad, we are committed to empowering learners with the knowledge and skills needed to excel in the ever-evolving field of data science. Whether you're starting from scratch or advancing your expertise, upGrad provides hands-on learning and career support to help you achieve tangible results in the competitive data science field.
Industry experts design our programs to ensure you gain hands-on experience with the latest tools and technologies in data science. Whether you’re just starting out or looking to enhance your expertise, upGrad has the perfect program for you. Here are some of our top offerings:
Unlock the power of data with our popular Data Science courses, designed to make you proficient in analytics, machine learning, and big data!
Explore our Popular Data Science Courses
Elevate your career by learning essential Data Science skills such as statistical modeling, big data processing, predictive analytics, and SQL!
Top Data Science Skills to Learn
Stay informed and inspired with our popular Data Science articles, offering expert insights, trends, and practical tips for aspiring data professionals!
Read our popular Data Science Articles
Frequently Asked Questions
1. What are data science tools, and why are they important?
2. Which programming languages are most commonly used in data science?
3. What are some must-know data visualization tools?
4. How do cloud-based data science tools help in collaborative projects?
5. What factors should I consider when choosing a data science tool?
6. Are there any free data science tools for beginners?
7. How can upGrad help me learn data science effectively?
8. Can I learn data science without prior programming experience?
9. How long does it take to become proficient in data science?
10. What career opportunities are available after learning data science?
11. Does upGrad provide placement support for data science learners?
Get Free Consultation
By submitting, I accept the T&C and
Privacy Policy
Start Your Career in Data Science Today
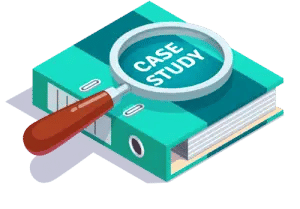
Top Resources