Natural language processing revolves around making computers understand and interpret human language. Different natural language processing tools are used to apply machine learning algorithms to process and evaluate natural language data, like text or speech.
In recent times, NLP has become applicable in multiple fields. Check out the top applications of NLP in the real world.
Text summarization
NLP can be useful for summarizing long articles and documents into shorter versions. This technique is often applied in applications for research paper summaries, news aggregation services, and other services for content curation.
For instance, news aggregators use NLP to convert a lengthy piece of news into an easier-to-read and concise version.
Speech recognition
Natural Language Processing tools is useful for converting spoken language into text, enabling voice-based interfaces and dictation. They are often used in applications like virtual assistants, speech-to-text transcription services, and various other voice-based applications.
The best NLP examples in speech recognition include virtual assistants like Amazon Alexa or Google Assistant. These virtual assistants leverage NLP to understand spoken instructions and can provide answers in natural language. These tools ensure that users no longer have to type out inquiries or commands.
Language Translation
In the past, translating a sentence from one language to another used to be excessively confusing and challenging. But with the help of NLP and artificial intelligence, algorithms can now be trained on texts of various languages.
Therefore, it has become easier to provide the same meaning in another language. This technology is also useful for translating traditionally more difficult languages like Chinese and Russian.
Sentiment Analysis
NLP is useful for analyzing text data to identify the sentiment of the writer toward a specific service, product, or brand. The concept of sentiment analysis is commonly applied in market research, customer feedback analysis, and social media monitoring.
One of the most common NLP applications for sentiment analysis is stock market evaluation. It revolves around traders and investors examining social media sentiment related to a specific stock or market. Sentiment analysis ensures that investors have the necessary information to modify their strategies and make more informed investment decisions.
Chatbots in
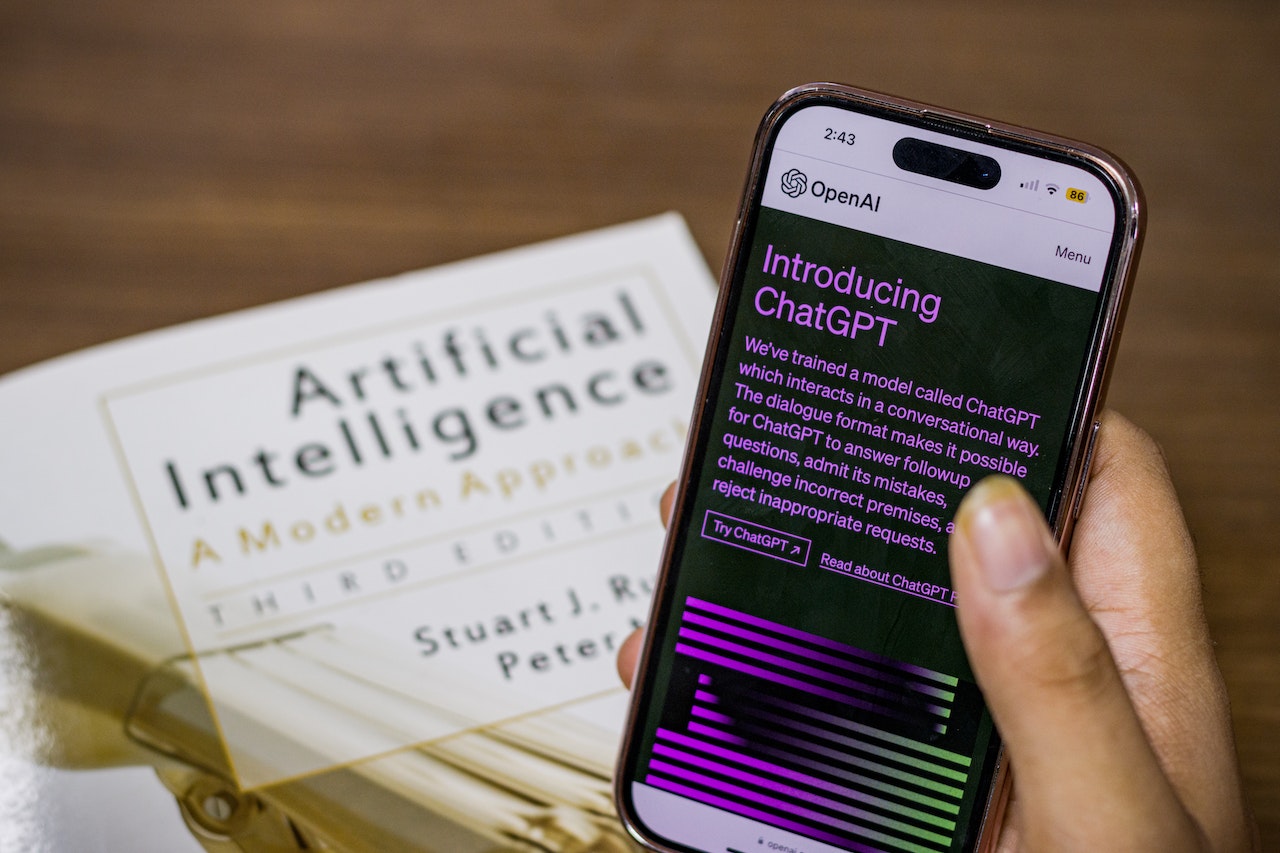
NLP is useful for developing conversational interfaces for chatbots that can comprehend and respond to queries in natural language. For instance, a chatbot like ChatGPT uses NLP to understand and answer the different questions raised by users.
Wrapping up
NLP applications in the real world are quite broad and powerful. In the years ahead, we will notice even better use cases of this cutting-edge technology.
FAQs:
- What constitutes the four pillars of natural language processing?
The four pillars of natural language processing include outcome orientation, rapport, flexibility, and sensory acuity.
- What is a common example of NLP?
Email filters are an extremely common example of natural language processing. The spam filters are basically where it all started. NLP can help with the identification of uncovered word patterns that can be linked to spam messages.
- Is Google Translate an example of NLP?
One of the major applications of natural language processing is machine translation. Therefore, one of the core systems powering Google Translate is NLP.
- Which coding language is the most suitable for natural language processing?
Python is the most suitable language for natural language processing. Some other languages preferred for NLP include Java and R.