In a rapidly evolving digital landscape, Machine learning has become the biggest boon technology. From reducing human interaction to increasing the scalability of global enterprises, the list of benefits from machine learning is never-ending. Machine learning can unlock the full potential of productive algorithms and models. This algorithm not only allows us to analyse the vast amount of data and provide predictions that shape the future.
This article will explore What machine learning is, Models of machine learning, how predictive algorithms work, and how machine learning unlocks endless possibilities.
What is Machine Learning?
Machine learning comes within computer science and artificial intelligence, focusing on data and algorithms and imitating how humans gradually learn. It allows software applications to predict the result accurately without training them. The predictive algorithms use historical data as input to give expected output by understanding the keyword. Machine learning is often categorised by how algorithms become accurate in giving predictions.
Machine learning models
These models are mathematical functions that take requests as input data, make predictions and then provide an output in response. Three 3-types of machine learning models are available based on different business goals and data sets.
Supervised learning
The Supervised Learning machine learning model is easy to comprehend. It involves using known labels or results as an output for input data, also referred to as training data. So, it works on the principle of input-output pairs.
Unsupervised learning
With Unsupervised Learning Algorithms, users can undertake more intricate processing tasks than supervised learning.
Reinforcement Learning
Reinforcement Learning is a Machine Learning method concerned with how software agents should act in an environment. It is a feedback-based meaning model that takes feedback after each action.
Predictive algorithms & importance
Predictive algorithms in machine learning work on making predictions about future outcomes using past data. These algorithms study the patterns in the data to understand relationships and then imply the data to forecast what might happen next.
Predictive algorithms are valuable to almost every sector as they help make decisions, optimise operations and help to gain a competitive edge in fields including- finance, healthcare, transportation, marketing etc.
Who is using machine learning?
These days machine learning is being utilised by almost every sector, including-
Finance
Financial institutions use machine learning to detect fraud, risk assessment, algorithmic trading, credit scoring, and customer segmentation.
Natural language processing
Machine learning also provides voice assistants, language translation, chatbots, and text summarisation to simplify individual tasks.
E-commerce and Retail
Machine learning is used for personalised recommendations, pricing optimisation, and retailer customer sentiment analysis.
Transportation and Logistics
Machine learning is vital in route optimisation, traffic detection, and autonomous vehicle development.
Marketing and Advertising
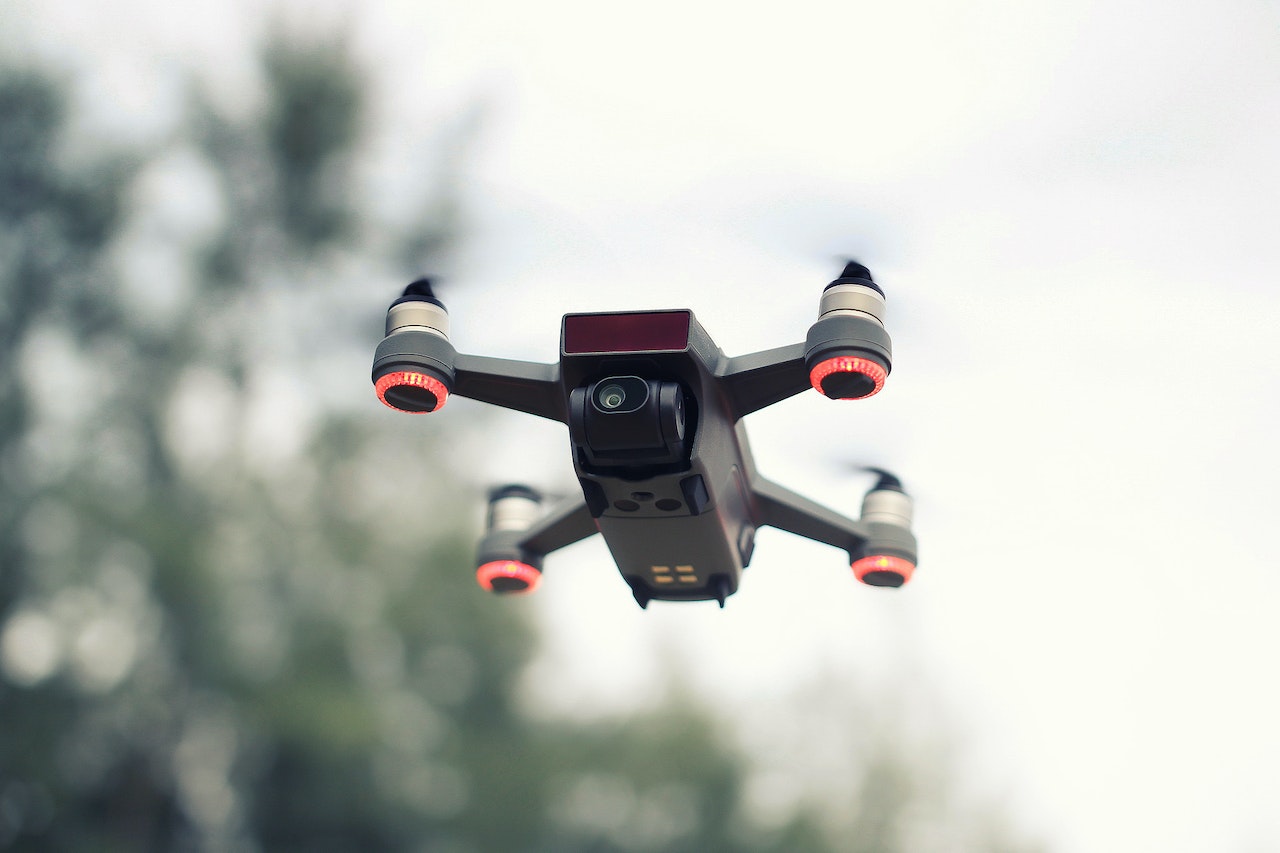
Machine learning is used for customer segmentation, targeted advertising, sentiment analysis, click-through rate prediction, and campaign optimisation.
Manufacturing and Supply Chain
In this sector, it helps in supply chain optimisation, detection in manufacturing processes, and quality control.
Agriculture
In agriculture, it helps to detect disease in plants, precision agriculture, and automated farming systems.
Government and Public Services
Most importantly, machine learning is strongly utilised in fraud detection, cybersecurity, traffic management, and public safety. These are just a few examples, whereas machine learning has applications in numerous other sectors, demonstrating its wide-ranging impact across various industries.
Conclusion
Machine learning is indeed a powerful tool to predict data. It is making human tasks easier and conquering almost every sector. It’s important to note that machine learning depends on data quality. To achieve accurate predictions, it’s essential to use data of high quality.