Big data analytics tools are invaluable in harnessing the power of data across industries. As data volumes grow exponentially, organizations need robust, significant data software to aggregate information, spot patterns, predict future trends, optimize operations, and aid real-time decision-making. The right big data tools provide the capabilities to efficiently process extensive datasets and unlock deep insights that can transform business growth.
Overview of Key Big Data Analytics Tools
There is a wide range of big data analytics tools available today that fulfil different functions across the data analysis pipeline. Some key categories and leading options include:
Big Data Collection and Storage Tools
Hadoop
- Open-source big data framework for storing and processing large datasets across clusters of commodity hardware
- Enables scalable and cost-efficient data lakes
Apache Kafka
- Performs real-time data streaming from diverse sources
- Facilitates building data pipelines and applications reliant on streaming data
Big Data Processing Tools
Spark
- Open-source data processing engine designed for large-scale data analytics and AI
- Enables rapid batch processing and stream processing for extract-transform-load tasks
Flink
- Performs continuous batch and stream data processing with minimal latency
- Suitable for real-time applications and iterative workloads
Big Data Visualization Tools
Tableau
- Drag and drop interface to visualize data patterns
- Generates interactive dashboards, reports and analytics
Qlik
- Creates dynamic data visualizations, dashboards and apps
- Provides self-service BI capabilities
Power BI
- Interactive data visualization and business intelligence platform
- User-friendly interface to analyze data and share insights
Big Data Infrastructure Tools
Cloudera
- Leading enterprise data cloud platform
- Enables deploying Hadoop and Spark on robust enterprise-grade infrastructure
Key Benefits of Using Big Data Analytics Tools
Deploying the right big data tools provides multifaceted benefits spanning:
- Scalable Data Storage and Management: Tools like Hadoop enable cost-efficient storage and analysis of multi-structured datasets in optimized data lakes. This allows unhindered data growth.
- Real-Time Data Streaming and Processing: With data velocity intensifying, platforms like Spark and Flink allow building applications to consume real-time data from diverse sources and obtain low-latency insights.
- Interactive Data Visualization and Exploration: Modern big data visualization tools like Tableau make analyzing complex data sets intuitive through easy-to-use graphical interfaces. This uncovers hidden patterns.
- Improved Decision Making: By providing the foundation for advanced analytics, big data tools lead to data-driven decisions through actionable intelligence. This catalyzes innovation.
In summary, big data analytics tools are unlocking immense potential within enterprise data assets to sharpen competitive edge.
Key Capabilities to Evaluate in Big Data Tools
While evaluating big data analytics tools, some vital aspects to analyze across criteria include:
- Scale: Ability to handle exponentially growing data volumes
- Velocity: Enabling real-time data processing with minimal latency
- Variety: Managing diverse structured and multi-structured data types
- Visualization: Data exploration through intuitive and interactive visual interfaces
- Analytics: Embedded predictive modeling, machine learning algorithms
- Security: Granular user access, authentication, encryption
- Governance: Metadata management, data lifecycle management, and regulatory compliance
Choosing solutions that sufficiently fulfil these critical criteria is imperative to tap the true potential of big data analytics.
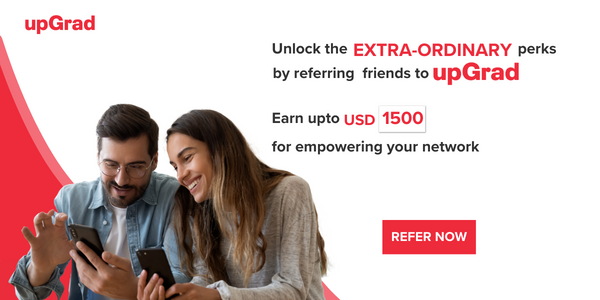
Conclusion
In conclusion, with data emerging as the most valuable asset in the digital era, leveraging robust and scalable big data software solutions is becoming imperative for organizations across sectors. Investing in enterprise-grade tools that allow harnessing complex data to derive actionable intelligence will be the key competitive differentiator going forward.
Frequently Asked Questions
1. What are some popular open-source big data tools?
Some widely used open-source big data tools include Hadoop, Spark, Kafka, Flink, Elasticsearch, Airflow, and Presto.
2. What are some leading cloud-based big data analytics tools?
Prominent cloud-based extensive data services include Amazon EMR, Azure HDInsight, Google BigQuery, Snowflake, and Databricks.
3. What are some key differences between Hadoop and Spark?
While Hadoop provides distributed data storage and batch processing, Spark offers increased processing speeds for iterative workloads. Spark also has libraries for SQL, streaming, machine learning and graph analytics.
4. What are some essential capabilities of big data visualization tools?
Critical capabilities of visualization tools involve an easy drag-and-drop interface, dynamic and interactive dashboards, geospatial analytics, real-time report generation, and sharing of data stories.
5. What are some popular enterprise Hadoop distributions?
Cloudera, Hortonworks, MapR, and cloud providers like AWS, Azure, and Google Cloud offer leading enterprise-grade Hadoop distributions.
6. What are the key features of a robust data analytics architecture?
A robust modern analytics architecture should provide scalable data storage, real-time data pipelines, interactive BI, advanced analytics, and maximum governance and security.
7. Why should organizations invest in big data capabilities?
Processing high-velocity, high-variety data with analytics tools improves decision automation, boosts operational efficiency, allows better customer experiences, and accelerates innovation and revenue growth.