In the ever-evolving world of artificial intelligence (AI) and machine learning (ML), neural networks and deep learning have emerged as powerful techniques that fuel advancements in various fields. As professionals looking to upskill and stay updated in this rapidly changing landscape, it’s essential to understand the fundamentals of neural networks and deep learning.
In this blog, we’ll explore these concepts, delve into their significance in advanced AI and ML, and discuss key details you should know in 2023.
Understanding Neural Networks
Neural networks are computational models inspired by the human brain’s neural structure. They consist of interconnected artificial neurons (also known as nodes) organized into layers. Each neuron receives inputs, processes them through an activation function, and passes the output to the next layer until the desired output is achieved.
Here are some key details to know about neural networks:
Architecture
Neural networks can have various architectures, but the most common one is the feedforward neural network. It comprises an input layer, one or more hidden layers, and an output layer. Each layer consists of multiple neurons, and connections between neurons carry weighted signals.
Training Process
Training a neural network involves feeding it with labeled input data and adjusting the weights between neurons iteratively. This process is known as backpropagation, where the network learns from errors and adjusts the weights to improve its predictions.
Types of Neural Networks
There are different types of neural networks designed for specific tasks:
- Convolutional Neural Networks (CNNs): Ideal for image and video analysis, CNNs use convolutional layers to extract relevant features.
- Recurrent Neural Networks (RNNs): Suitable for sequential data, RNNs have connections that allow information to flow in loops, making them effective for tasks like speech recognition and natural language processing.
- Generative Adversarial Networks (GANs): GANs consist of a generator and a discriminator network that compete with each other, leading to remarkable results in tasks like image generation and style transfer.
Understanding Deep Learning
Deep learning is a subset of ML that focuses on training neural networks with multiple layers. It allows the network to learn and extract hierarchical representations from complex data. Deep learning has gained significant traction due to its ability to solve intricate problems and achieve state-of-the-art performance. Here’s what you should know about deep learning:
Application Areas
Deep learning has revolutionized various fields, including:
- Computer Vision: Deep learning has led to remarkable advancements in image classification, object detection, and facial recognition.
- Natural Language Processing (NLP): Deep learning has improved machine translation, sentiment analysis, text generation, and language understanding.
- Healthcare: Deep learning enables medical image analysis, disease diagnosis, and personalized treatment recommendations.
- Autonomous Vehicles: Deep learning plays a crucial role in perception, object recognition, and decision-making for self-driving cars.
The Future of Neural Networks and Deep Learning
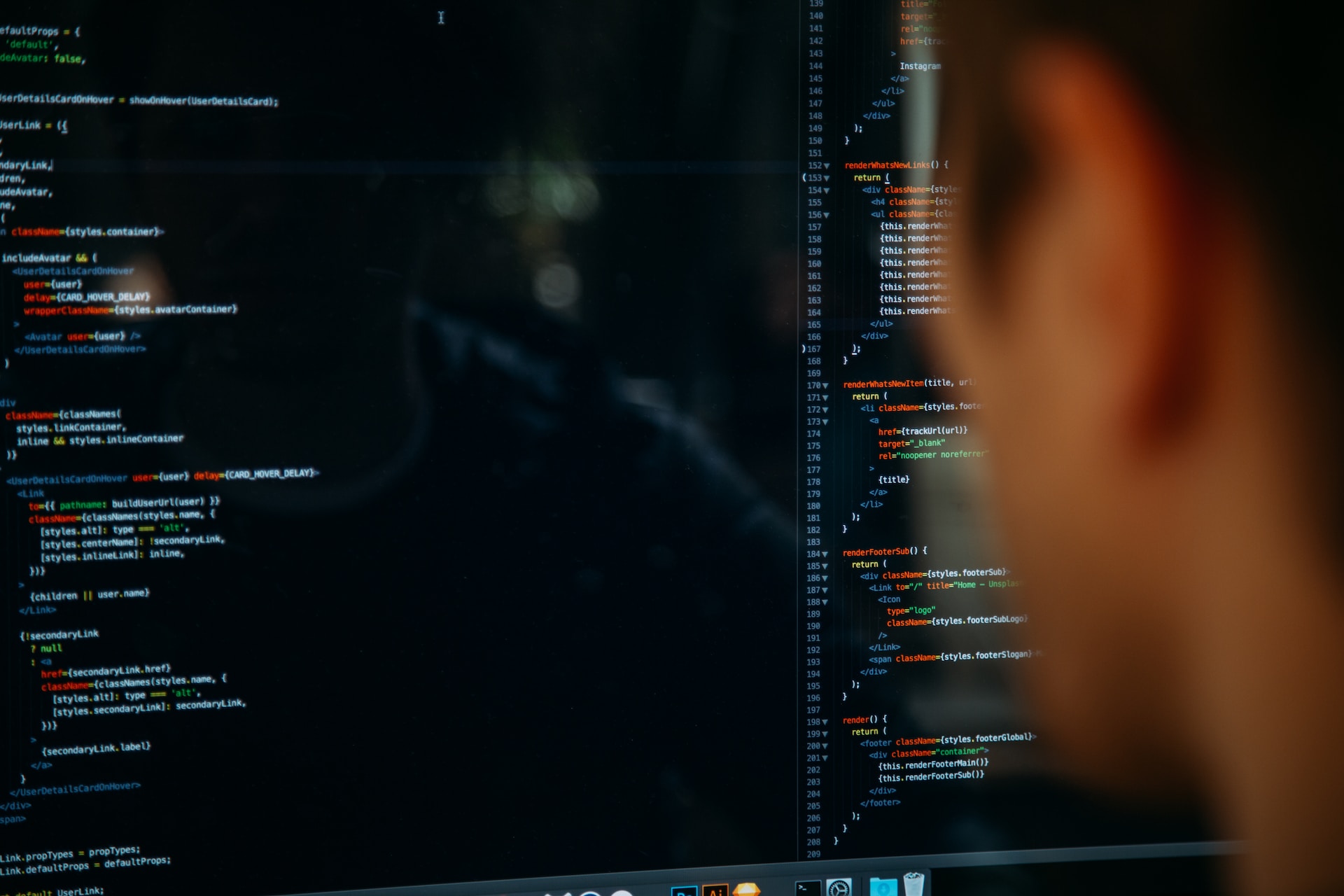
As we look ahead to 2023 and beyond, neural networks and deep learning will continue to shape the future of AI and ML. Here are some trends and developments to keep an eye on:
- Explainable AI: Interpreting the decisions made by neural networks is a growing concern. Researchers are actively working on developing techniques to make neural networks more transparent and interpretable, allowing users to understand and trust the reasoning behind their decisions.
- Transfer Learning and Pretrained Models: Transfer learning, a technique where pre-trained models are fine-tuned for specific tasks, will gain more prominence. Pretrained models offer a head start by leveraging knowledge learned from massive datasets, making it easier to apply deep learning to new domains with limited data.
- Federated Learning: Federated learning enables training models on decentralized data sources without sharing the raw data, addressing privacy concerns. This approach will gain traction as organizations strive to maintain data privacy while benefiting from the collective knowledge of distributed networks.
- Edge Computing: With the proliferation of IoT devices and the need for real-time inference, deep learning models will be deployed at the edge, closer to where the data is generated. This reduces latency, enhances privacy, and enables offline inference, making deep learning more accessible and efficient.
Conclusion
As we move into 2023, neural networks and deep learning will continue to revolutionize various industries, enabling breakthroughs in computer vision, natural language processing, healthcare, and autonomous systems. Embracing these technologies and staying curious about emerging trends will empower professionals to navigate the exciting world of AI and ML with confidence.